Fofr
Models by this creator
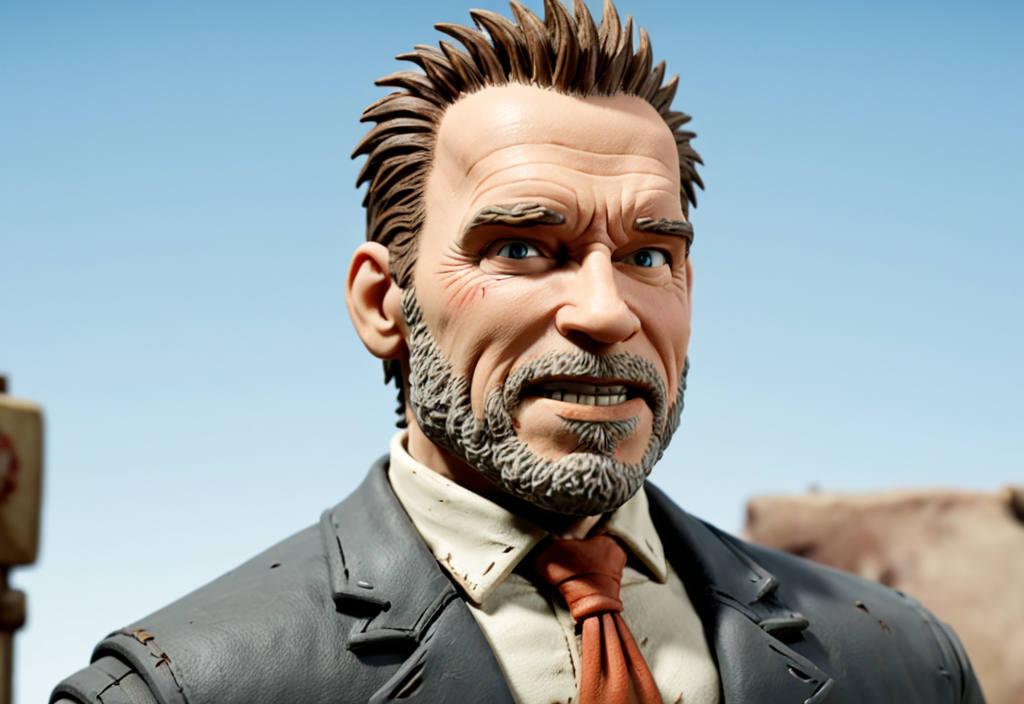
face-to-many

10.7K
The face-to-many model is a versatile AI tool that allows you to turn any face into a variety of artistic styles, such as 3D, emoji, pixel art, video game, claymation, or toy. Developed by fofr, this model is part of a larger collection of creative AI tools from the Replicate platform. Similar models include sticker-maker for generating stickers with transparent backgrounds, real-esrgan for high-quality image upscaling, and instant-id for creating realistic images of people. Model inputs and outputs The face-to-many model takes in an image of a person's face and a target style, allowing you to transform the face into a range of artistic representations. The model outputs an array of generated images in the selected style. Inputs Image**: An image of a person's face to be transformed Style**: The desired artistic style to apply, such as 3D, emoji, pixel art, video game, claymation, or toy Prompt**: A text description to guide the image generation (default is "a person") Negative Prompt**: Text describing elements you don't want in the image Prompt Strength**: The strength of the prompt, with higher numbers leading to a stronger influence Denoising Strength**: How much of the original image to keep, with 1 being a complete destruction and 0 being the original Instant ID Strength**: The strength of the InstantID model used for facial recognition Control Depth Strength**: The strength of the depth controlnet, affecting how much it influences the output Seed**: A fixed random seed for reproducibility Custom LoRA URL**: An optional URL to a custom LoRA (Learned Residual Adapter) model LoRA Scale**: The strength of the custom LoRA model Outputs An array of generated images in the selected artistic style Capabilities The face-to-many model excels at transforming faces into a wide range of artistic styles, from the detailed 3D rendering to the whimsical pixel art or claymation. The model's ability to capture the essence of the original face while applying these unique styles makes it a powerful tool for creative projects, digital art, and even product design. What can I use it for? With the face-to-many model, you can create unique and eye-catching visuals for a variety of applications, such as: Generating custom avatars or character designs for video games, apps, or social media Producing stylized portraits or profile pictures with a distinctive flair Designing fun and engaging stickers, emojis, or other digital assets Prototyping physical products like toys, figurines, or collectibles Exploring creative ideas and experimenting with different artistic interpretations of a face Things to try The face-to-many model offers a wide range of possibilities for creative experimentation. Try combining different styles, adjusting the input parameters, or using custom LoRA models to see how the output can be further tailored to your specific needs. Explore the limits of the model's capabilities and let your imagination run wild!
Updated 5/8/2024
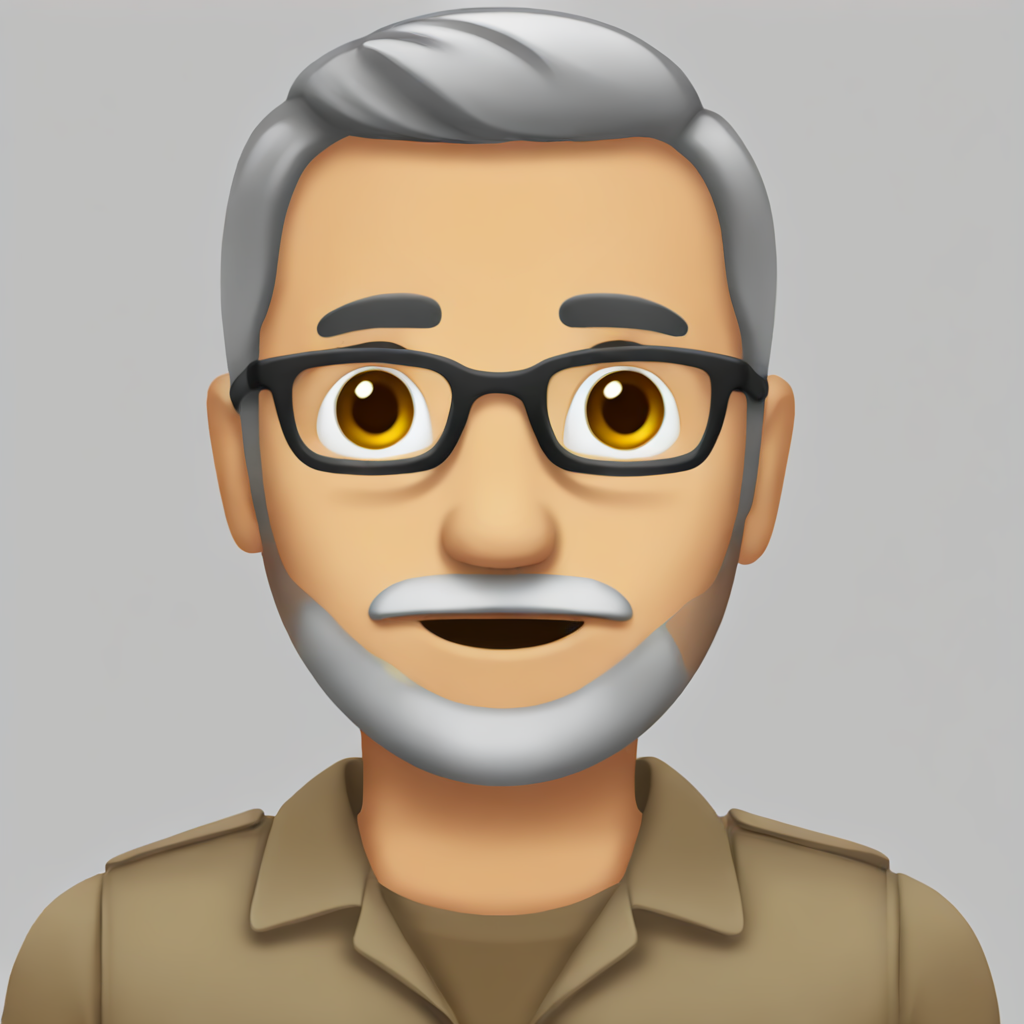
sdxl-emoji

4.2K
sdxl-emoji is an SDXL (Stable Diffusion XL) fine-tuned model created by fofr that specializes in generating images based on Apple Emojis. This model builds upon the capabilities of the original Stable Diffusion model, adding specialized knowledge and training to produce high-quality, emoji-themed images. It can be seen as a variant of similar SDXL models like sdxl-color, realistic-emoji, sdxl-2004, sdxl-deep-down, and sdxl-black-light, each with their own unique focus and capabilities. Model inputs and outputs The sdxl-emoji model accepts a variety of inputs, including text prompts, images, and various parameters to control the generation process. Users can provide a prompt describing the type of emoji they want to generate, along with optional modifiers like the size, color, or style. The model can also take in an existing image and perform inpainting or image-to-image generation tasks. Inputs Prompt**: A text description of the emoji you want to generate Image**: An existing image to use as a starting point for inpainting or image-to-image generation Seed**: A random seed value to control the randomness of the generation process Width/Height**: The desired dimensions of the output image Num Outputs**: The number of images to generate Guidance Scale**: The scale for classifier-free guidance, which affects the balance between the prompt and the model's own generation Num Inference Steps**: The number of denoising steps to perform during the generation process Outputs Image(s)**: One or more generated images matching the input prompt and parameters Capabilities The sdxl-emoji model excels at generating a wide variety of emoji-themed images, from simple cartoon-style emojis to more realistic, photorealistic renderings. It can capture the essence of different emoji expressions, objects, and scenes, and combine them in unique and creative ways. The model's fine-tuning on Apple's emoji dataset allows it to produce results that closely match the visual style and aesthetics of official emojis. What can I use it for? The sdxl-emoji model can be a powerful tool for a variety of applications, such as: Social media and messaging**: Generate custom emoji-style images to use in posts, messages, and other digital communications. Creative projects**: Incorporate emoji-inspired visuals into design projects, illustrations, or digital art. Education and learning**: Use the model to create engaging, emoji-themed educational materials or learning aids. Branding and marketing**: Develop unique, emoji-based brand assets or promotional materials. Things to try With the sdxl-emoji model, you can experiment with a wide range of prompts and parameters to explore the limits of its capabilities. Try generating emojis with different expressions, moods, or settings, or combine them with other visual elements to create more complex scenes and compositions. You can also explore the model's ability to perform inpainting or image-to-image generation tasks, using existing emoji-themed images as starting points for further refinement or transformation.
Updated 5/8/2024
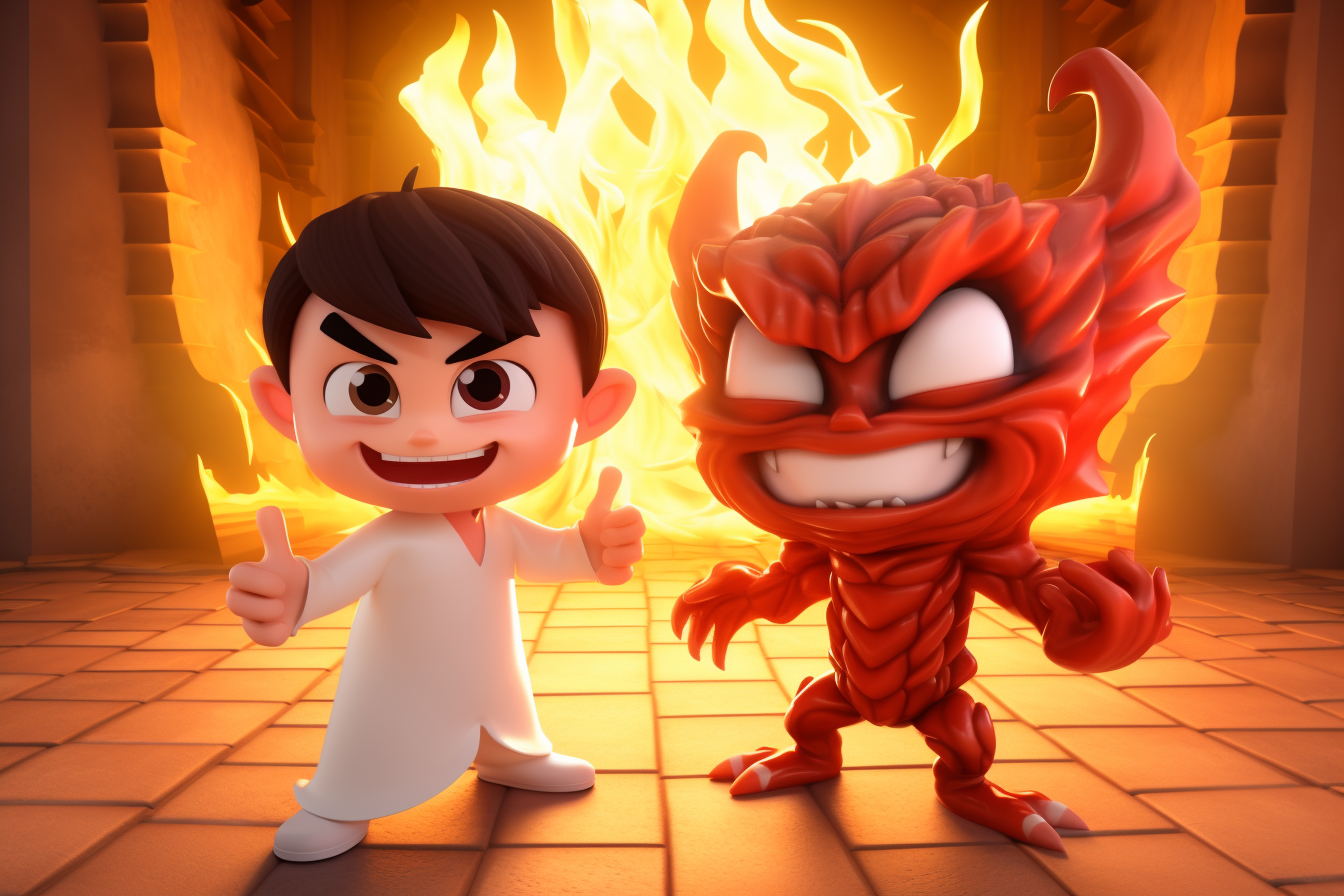
prompt-classifier

1.6K
prompt-classifier is a model that determines the toxicity of text-to-image prompts. It is a fine-tuned version of the llama-13b language model, with a focus on assessing the safety and appropriateness of prompts used to generate images. The model outputs a safety ranking between 0 (safe) and 10 (toxic) for a given prompt. This can be useful for content creators, AI model developers, and others who work with text-to-image generation to ensure their prompts do not produce harmful or undesirable content. Similar models include codellama-13b-instruct, a 13 billion parameter Llama tuned for coding and conversation, and llamaguard-7b, a 7 billion parameter Llama 2-based input-output safeguard model. Model inputs and outputs prompt-classifier takes a text prompt as input and outputs a safety ranking between 0 and 10, indicating the level of toxicity or inappropriateness in the prompt. Inputs Prompt**: The text prompt to be evaluated for safety. Seed**: A random seed value, which can be left blank to randomize the seed. Debug**: A boolean flag to enable debugging output. Top K**: The number of most likely tokens to consider when decoding the text. Top P**: The percentage of most likely tokens to consider when decoding the text. Temperature**: A value adjusting the randomness of the output, with higher values being more random. Max New Tokens**: The maximum number of new tokens to generate. Min New Tokens**: The minimum number of new tokens to generate (or -1 to disable). Stop Sequences**: A comma-separated list of sequences to stop generation at. Replicate Weights**: The path to fine-tuned weights produced by a Replicate fine-tune job. Outputs The model outputs a list of strings representing the predicted safety ranking for the input prompt. Capabilities The prompt-classifier model is designed to assess the toxicity and safety of text-to-image prompts. This can be useful for content creators, AI model developers, and others who work with text-to-image generation to ensure their prompts do not produce harmful or undesirable content. What can I use it for? The prompt-classifier model can be used to screen text-to-image prompts before generating images, helping to ensure the prompts do not produce content that is toxic, inappropriate, or unsafe. This can be particularly helpful for content creators, publishers, and others who rely on text-to-image generation, as it allows them to proactively identify and address potentially problematic prompts. Things to try One interesting thing to try with the prompt-classifier model is to use it as part of a broader system for managing and curating text-to-image prompts. For example, you could integrate the model into a workflow where prompts are automatically evaluated for safety before being used to generate images. This could help streamline the content creation process and reduce the risk of producing harmful or undesirable content.
Updated 5/8/2024
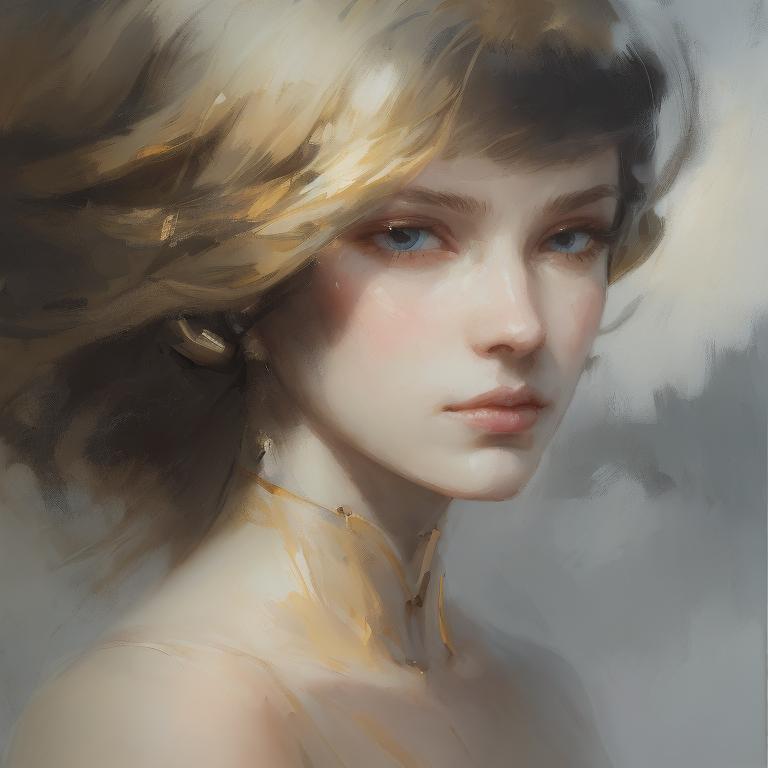
latent-consistency-model

909
The latent-consistency-model is a powerful AI model developed by fofr that offers super-fast image generation at 0.6s per image. It combines several key capabilities, including img2img, large batching, and Canny controlnet support. This model can be seen as a refinement and extension of similar models like sdxl-controlnet-lora and instant-id-multicontrolnet, which also leverage ControlNet technology for enhanced image generation. Model inputs and outputs The latent-consistency-model accepts a variety of inputs, including a prompt, image, width, height, number of images, guidance scale, and various ControlNet-related parameters. The model's outputs are an array of generated image URLs. Inputs Prompt**: The text prompt that describes the desired image Image**: An input image for img2img Width**: The width of the output image Height**: The height of the output image Num Images**: The number of images to generate per prompt Guidance Scale**: The scale for classifier-free guidance Control Image**: An image for ControlNet conditioning Prompt Strength**: The strength of the prompt when using img2img Sizing Strategy**: How to resize images, such as by width/height or based on input/control image LCM Origin Steps**: The number of steps for the LCM origin Canny Low Threshold**: The low threshold for the Canny edge detector Num Inference Steps**: The number of denoising steps Canny High Threshold**: The high threshold for the Canny edge detector Control Guidance Start**: The start of the ControlNet guidance Control Guidance End**: The end of the ControlNet guidance Controlnet Conditioning Scale**: The scale for ControlNet conditioning Outputs An array of URLs for the generated images Capabilities The latent-consistency-model is capable of generating high-quality images at a lightning-fast pace, making it an excellent choice for applications that require real-time or batch image generation. Its integration of ControlNet technology allows for enhanced control over the generated images, enabling users to influence the final output using various conditioning parameters. What can I use it for? The latent-consistency-model can be used in a variety of applications, such as: Rapid prototyping and content creation for designers, artists, and marketing teams Generative art projects that require quick turnaround times Integration into web applications or mobile apps that need to generate images on the fly Exploration of different artistic styles and visual concepts through the use of ControlNet conditioning Things to try One interesting aspect of the latent-consistency-model is its ability to generate images with a high degree of consistency, even when using different input parameters. This can be especially useful for creating cohesive visual styles or generating variations on a theme. Experiment with different prompts, image inputs, and ControlNet settings to see how the model responds and explore the possibilities for your specific use case.
Updated 5/8/2024
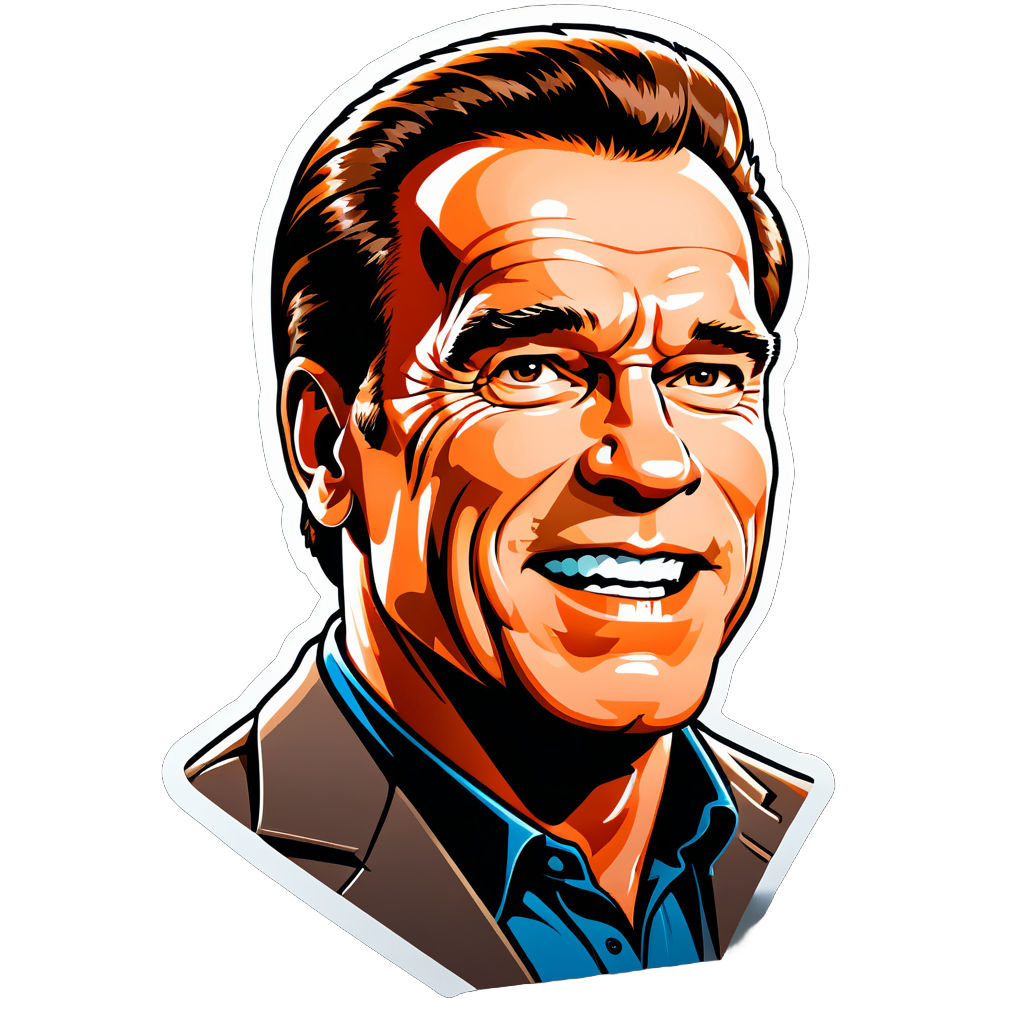
face-to-sticker

727
The face-to-sticker model is a tool that allows you to turn any face into a sticker. This model is created by the Replicate user fofr, who has also developed similar AI models like sticker-maker, face-to-many, and become-image. These models all focus on transforming faces into different visual styles using AI. Model inputs and outputs The face-to-sticker model takes an image of a person's face as input and generates a sticker-like output. You can also customize the model's output by adjusting parameters like the prompt, steps, width, height, and more. Inputs Image**: An image of a person's face to be converted into a sticker Prompt**: A text description of what you want the sticker to look like (default is "a person") Negative prompt**: Things you do not want to see in the sticker Prompt strength**: The strength of the prompt, with higher numbers leading to a stronger influence Steps**: The number of steps to take when generating the sticker Width and height**: The size of the output sticker Seed**: A number to fix the random seed for reproducibility Upscale**: Whether to upscale the sticker by 2x Upscale steps**: The number of steps to take when upscaling the sticker Ip adapter noise and weight**: Parameters that control the influence of the IP adapter on the final sticker Outputs The generated sticker image Capabilities The face-to-sticker model can take any face and transform it into a unique sticker-like image. This can be useful for creating custom stickers, emojis, or other graphics for social media, messaging, or other applications. What can I use it for? You can use the face-to-sticker model to create custom stickers and graphics for a variety of purposes, such as: Personalizing your messaging and social media with unique, AI-generated stickers Designing custom merchandise or products with your own face or the faces of others Experimenting with different visual styles and effects to create new and interesting graphics Things to try One interesting thing to try with the face-to-sticker model is to experiment with different prompts and parameters to see how they affect the final sticker. Try prompts that evoke different moods, emotions, or visual styles, and see how the model responds. You can also play with the upscaling and IP adapter settings to create more detailed or stylized stickers.
Updated 5/8/2024
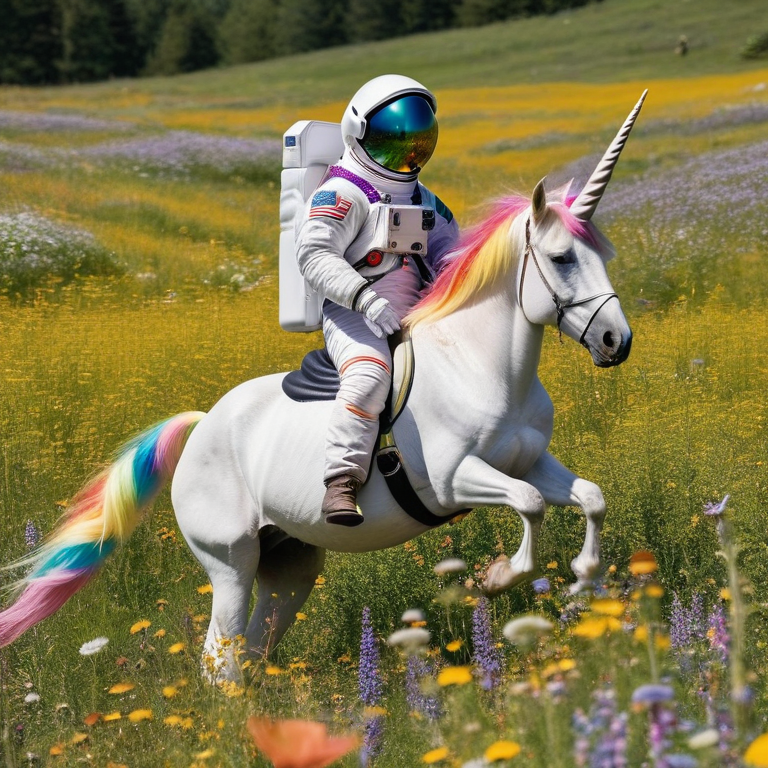
realvisxl-v3

374
The realvisxl-v3 is an advanced AI model developed by fofr that aims to produce highly photorealistic images. It is based on the SDXL (Stable Diffusion XL) model and has been further tuned for enhanced realism. This model can be contrasted with similar offerings like realvisxl-v3.0-turbo, realvisxl4, and realvisxl-v3-multi-controlnet-lora, which also target photorealism but with different approaches and capabilities. Model inputs and outputs The realvisxl-v3 model accepts a variety of inputs, including text prompts, images, and optional parameters like seed, guidance scale, and number of inference steps. The model can then generate one or more output images based on the provided inputs. Inputs Prompt**: The text prompt that describes the desired image to be generated. Negative prompt**: An optional text prompt that describes elements that should be excluded from the generated image. Image**: An optional input image that can be used for image-to-image or inpainting tasks. Mask**: An optional input mask that can be used for inpainting tasks, where black areas will be preserved and white areas will be inpainted. Seed**: An optional random seed value to ensure reproducible results. Width and height**: The desired width and height of the output image. Outputs Generated image(s)**: One or more images generated based on the provided inputs. Capabilities The realvisxl-v3 model is capable of producing highly realistic and photorealistic images based on text prompts. It can handle a wide range of subject matter, from landscapes and portraits to fantastical scenes. The model's tuning for realism results in outputs that are often indistinguishable from real photographs. What can I use it for? The realvisxl-v3 model can be a valuable tool for a variety of applications, such as digital art creation, content generation for marketing and advertising, and visual prototyping for product design. Its ability to generate photorealistic images can be particularly useful for projects that require high-quality visual assets, like virtual reality environments, movie and game assets, and product visualizations. Things to try One interesting aspect of the realvisxl-v3 model is its ability to handle a wide range of subject matter, from realistic scenes to more fantastical elements. You could try experimenting with different prompts that combine realistic and imaginative elements, such as "a photo of a futuristic city with flying cars" or "a portrait of a mythical creature in a realistic setting." The model's tuning for realism can produce some surprising and captivating results in these types of prompts.
Updated 5/8/2024
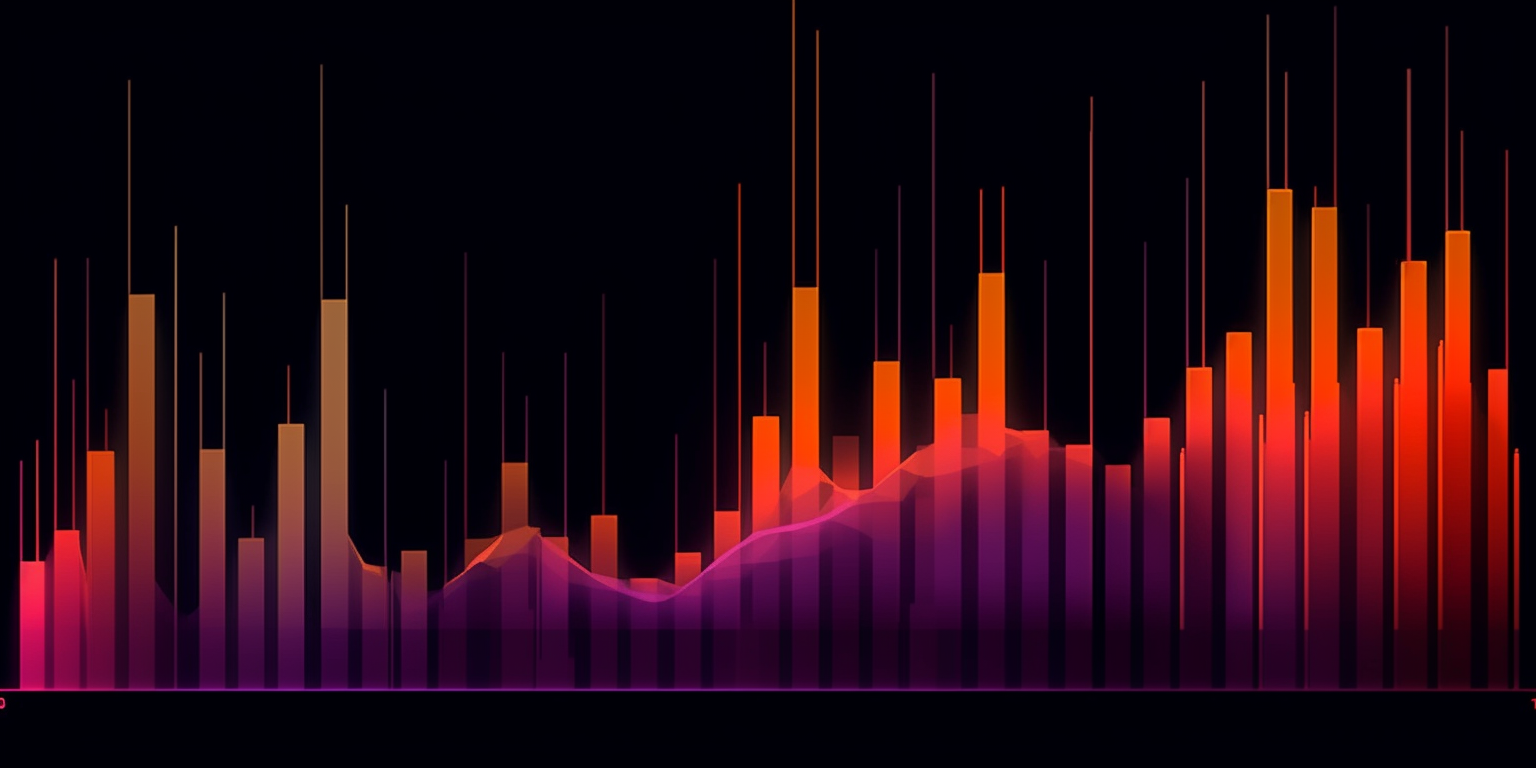
audio-to-waveform

328
The audio-to-waveform model allows you to create a waveform video from an audio file. This is similar to models like the toolkit model, which provides a video toolkit for converting, making GIFs, and extracting audio. The audio-to-waveform model is particularly useful for visualizing audio data in an engaging way. Model inputs and outputs The audio-to-waveform model takes an audio file as input and produces a waveform video as output. The input audio file can be in any format, and the model allows you to customize the appearance of the waveform, such as the background color, foreground opacity, bar count, and bar width. Inputs audio**: The audio file to create the waveform from bg_color**: The background color of the waveform (default is #000000) fg_alpha**: The opacity of the foreground waveform (default is 0.75) bar_count**: The number of bars in the waveform (default is 100) bar_width**: The width of the bars in the waveform (default is 0.4) bars_color**: The color of the waveform bars (default is #ffffff) caption_text**: The caption text to display in the video (default is empty) Outputs Output**: The generated waveform video Capabilities The audio-to-waveform model can be used to create visually appealing waveform videos from audio files. This can be useful for creating music visualizations, podcast previews, or other audio-based content. What can I use it for? The audio-to-waveform model can be used in a variety of projects, such as: Creating music videos or visualizations for songs Generating waveform previews for podcasts or audiobooks Incorporating waveform graphics into presentations or social media content Exploring the visual representation of audio data Things to try One interesting thing to try with the audio-to-waveform model is experimenting with different input parameters to create unique waveform styles. For example, you could try adjusting the bar width, bar count, or colors to see how it changes the overall look and feel of the generated video. Additionally, you could explore using the model alongside other tools, such as the toolkit model, to create more complex multimedia projects.
Updated 5/8/2024
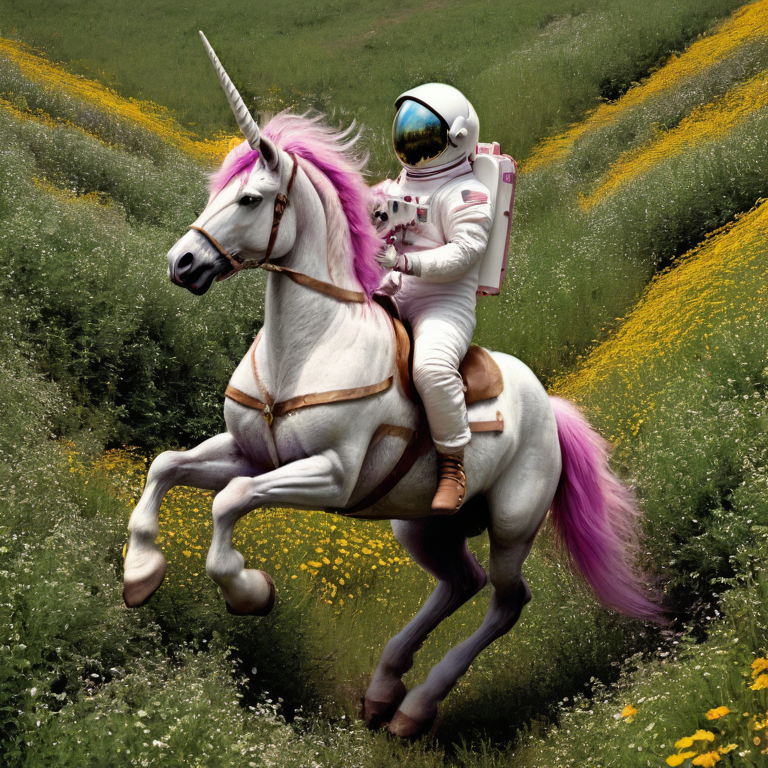
realvisxl-v3-multi-controlnet-lora

252
The realvisxl-v3-multi-controlnet-lora model is a powerful AI model developed by fofr that builds upon the RealVis XL V3 architecture. This model supports a range of advanced features, including img2img, inpainting, and the ability to use up to three simultaneous ControlNets with different input images. The model also includes custom Replicate LoRA loading, which allows for additional fine-tuning and optimization. Similar models include the sdxl-controlnet-lora from batouresearch, which focuses on Canny ControlNet with LoRA support, and the controlnet-x-ip-adapter-realistic-vision-v5 from usamaehsan, which offers a range of inpainting and ControlNet capabilities. Model inputs and outputs The realvisxl-v3-multi-controlnet-lora model takes a variety of inputs, including an input image, a prompt, and optional mask and seed values. The model can also accept up to three ControlNet images, each with its own conditioning strength, start, and end controls. Inputs Prompt**: The text prompt that describes the desired image. Image**: The input image for img2img or inpainting mode. Mask**: The input mask for inpainting mode, where black areas will be preserved and white areas will be inpainted. Seed**: The random seed value, which can be left blank to randomize. ControlNet 1, 2, and 3 Images**: Up to three separate input images for the ControlNet conditioning. ControlNet Conditioning Scales, Starts, and Ends**: Controls for adjusting the strength and timing of the ControlNet conditioning. Outputs Generated Images**: The model outputs one or more images based on the provided inputs. Capabilities The realvisxl-v3-multi-controlnet-lora model offers a wide range of capabilities, including high-quality img2img and inpainting, the ability to use multiple ControlNets simultaneously, and support for custom LoRA loading. This allows for a high degree of customization and fine-tuning to achieve desired results. What can I use it for? With its advanced features, the realvisxl-v3-multi-controlnet-lora model can be used for a variety of creative and practical applications. Artists and designers could use it to generate photorealistic images, experiment with different ControlNet combinations, or refine existing images. Businesses could leverage the model for tasks like product visualization, architectural rendering, or even custom content creation. Things to try One interesting aspect of the realvisxl-v3-multi-controlnet-lora model is the ability to use up to three ControlNets simultaneously. This allows users to explore the interplay between different visual cues, such as depth, edges, and body poses, to create unique and compelling images. Experimenting with the various ControlNet conditioning strengths, starts, and ends can lead to a wide range of stylistic and compositional outcomes.
Updated 5/8/2024
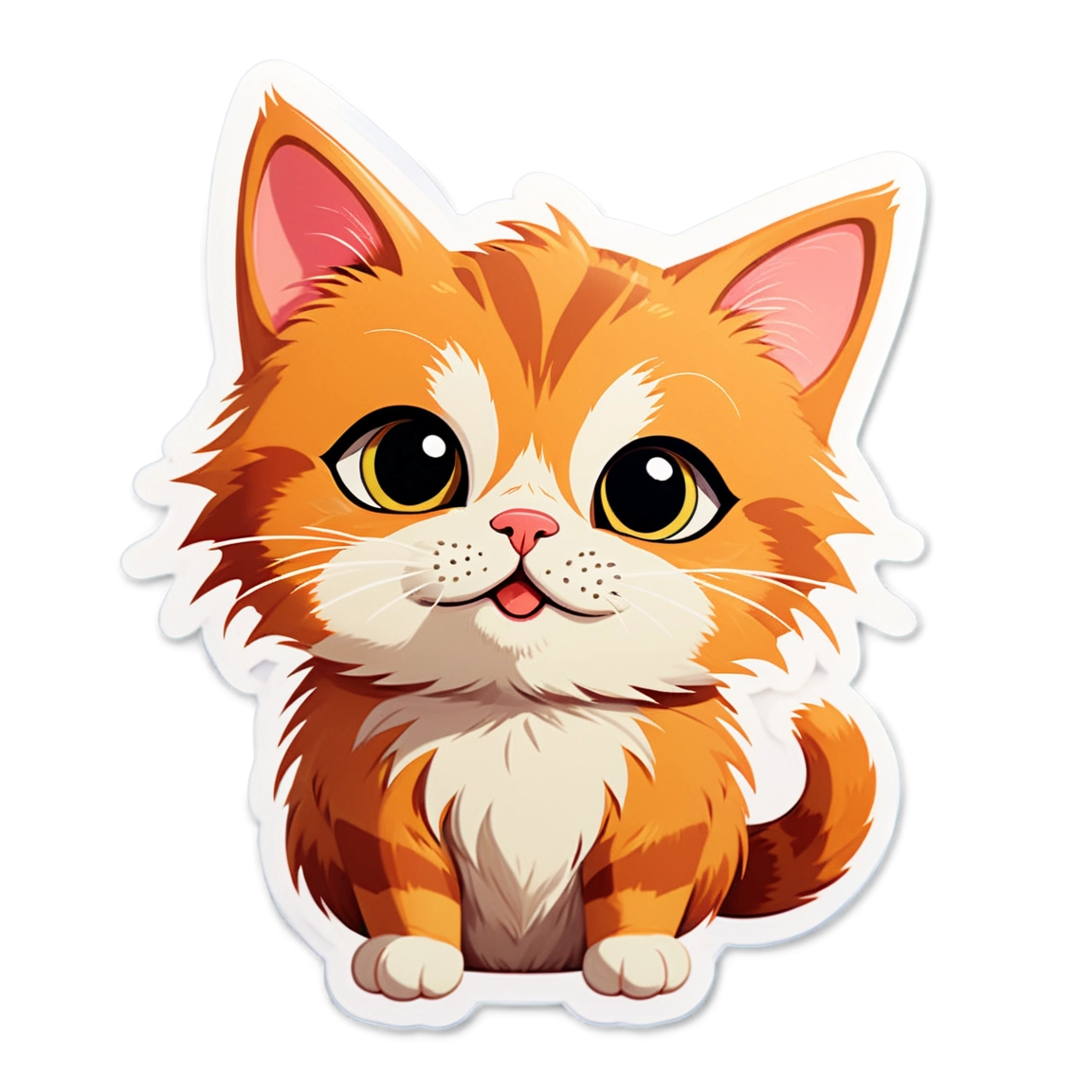
sticker-maker

240
The sticker-maker model is a powerful AI tool that enables users to generate high-quality graphics with transparent backgrounds, making it an ideal solution for creating custom stickers. Compared to similar models like AbsoluteReality V1.8.1, Reliberate v3, and any-comfyui-workflow, the sticker-maker model offers a streamlined and user-friendly interface, allowing users to quickly and easily create unique sticker designs. Model inputs and outputs The sticker-maker model takes a variety of inputs, including a seed for reproducibility, the number of steps to use, the desired width and height of the output images, a prompt to guide the generation, a negative prompt to exclude certain elements, the output format, and the desired quality of the output images. The model then generates one or more images with transparent backgrounds, which can be used to create custom stickers. Inputs Seed**: Fix the random seed for reproducibility Steps**: The number of steps to use in the generation process Width**: The desired width of the output images Height**: The desired height of the output images Prompt**: The text prompt used to guide the generation Negative Prompt**: Specify elements to exclude from the generated images Output Format**: The format of the output images (e.g., WEBP) Output Quality**: The quality of the output images, from 0 to 100 (100 is best) Number of Images**: The number of images to generate Outputs Array of image URLs**: The generated images with transparent backgrounds, which can be used to create custom stickers Capabilities The sticker-maker model is capable of generating a wide variety of sticker designs, ranging from cute and whimsical to more abstract and artistic. By adjusting the input prompts and settings, users can create stickers that fit their specific needs and preferences. What can I use it for? The sticker-maker model is a versatile tool that can be used for a variety of applications, such as creating custom stickers for personal use, selling on platforms like Etsy, or incorporating into larger design projects. The transparent backgrounds of the generated images make them easy to incorporate into various designs and layouts. Things to try To get the most out of the sticker-maker model, you can experiment with different input prompts and settings to see how they affect the generated stickers. Try prompts that evoke specific moods or styles, or mix and match different elements to create unique designs. You can also try generating multiple stickers and selecting the ones that best fit your needs.
Updated 5/8/2024
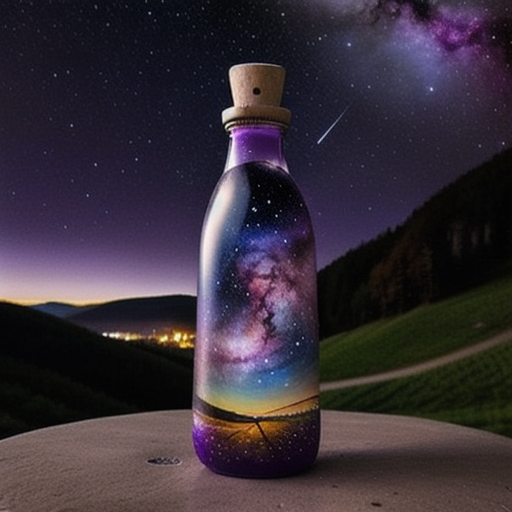
any-comfyui-workflow

192
The any-comfyui-workflow model allows you to run any ComfyUI workflow on Replicate. ComfyUI is a visual AI tool used to create and customize generative AI models. This model provides a way to run those workflows on Replicate's infrastructure, without needing to set up the full ComfyUI environment yourself. It includes support for many popular model weights and custom nodes, making it a flexible solution for working with ComfyUI. Model inputs and outputs The any-comfyui-workflow model takes two main inputs: a JSON file representing your ComfyUI workflow, and an optional input file (image, tar, or zip) to use within that workflow. The workflow JSON must be the "API format" exported from ComfyUI, which contains the details of your workflow without the visual elements. Inputs Workflow JSON**: Your ComfyUI workflow in JSON format, exported using the "Save (API format)" option Input File**: An optional image, tar, or zip file containing input data for your workflow Outputs Output Files**: The outputs generated by running your ComfyUI workflow, which can include images, videos, or other files Capabilities The any-comfyui-workflow model is a powerful tool for working with ComfyUI, as it allows you to run any workflow you've created on Replicate's infrastructure. This means you can leverage the full capabilities of ComfyUI, including the various model weights and custom nodes that have been integrated, without needing to set up the full development environment yourself. What can I use it for? With the any-comfyui-workflow model, you can explore and experiment with a wide range of generative AI use cases. Some potential applications include: Creative Content Generation**: Use ComfyUI workflows to generate unique images, animations, or other media assets for creative projects. AI-Assisted Design**: Integrate ComfyUI workflows into your design process to quickly generate concepts, visualizations, or prototypes. Research and Experimentation**: Test out new ComfyUI workflows and custom nodes to push the boundaries of what's possible with generative AI. Things to try One interesting aspect of the any-comfyui-workflow model is the ability to customize your JSON input to change parameters like seeds, prompts, or other workflow settings. This allows you to fine-tune the outputs and explore the creative potential of ComfyUI in more depth. You could also try combining the any-comfyui-workflow model with other Replicate models, such as become-image or instant-id, to create more complex AI-powered workflows.
Updated 5/8/2024