realvisxl-v3-multi-controlnet-lora
Maintainer: fofr

279
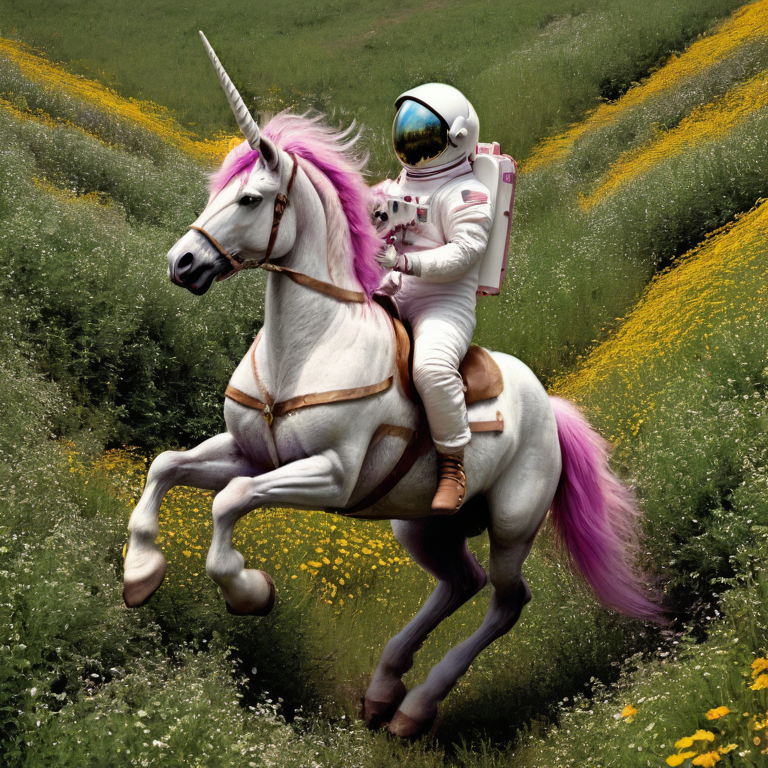
Property | Value |
---|---|
Model Link | View on Replicate |
API Spec | View on Replicate |
Github Link | View on Github |
Paper Link | View on Arxiv |
Get summaries of the top AI models delivered straight to your inbox:
Model overview
The realvisxl-v3-multi-controlnet-lora
model is a powerful AI model developed by fofr that builds upon the RealVis XL V3 architecture. This model supports a range of advanced features, including img2img, inpainting, and the ability to use up to three simultaneous ControlNets with different input images. The model also includes custom Replicate LoRA loading, which allows for additional fine-tuning and optimization.
Similar models include the sdxl-controlnet-lora from batouresearch, which focuses on Canny ControlNet with LoRA support, and the controlnet-x-ip-adapter-realistic-vision-v5 from usamaehsan, which offers a range of inpainting and ControlNet capabilities.
Model inputs and outputs
The realvisxl-v3-multi-controlnet-lora
model takes a variety of inputs, including an input image, a prompt, and optional mask and seed values. The model can also accept up to three ControlNet images, each with its own conditioning strength, start, and end controls.
Inputs
- Prompt: The text prompt that describes the desired image.
- Image: The input image for img2img or inpainting mode.
- Mask: The input mask for inpainting mode, where black areas will be preserved and white areas will be inpainted.
- Seed: The random seed value, which can be left blank to randomize.
- ControlNet 1, 2, and 3 Images: Up to three separate input images for the ControlNet conditioning.
- ControlNet Conditioning Scales, Starts, and Ends: Controls for adjusting the strength and timing of the ControlNet conditioning.
Outputs
- Generated Images: The model outputs one or more images based on the provided inputs.
Capabilities
The realvisxl-v3-multi-controlnet-lora
model offers a wide range of capabilities, including high-quality img2img and inpainting, the ability to use multiple ControlNets simultaneously, and support for custom LoRA loading. This allows for a high degree of customization and fine-tuning to achieve desired results.
What can I use it for?
With its advanced features, the realvisxl-v3-multi-controlnet-lora
model can be used for a variety of creative and practical applications. Artists and designers could use it to generate photorealistic images, experiment with different ControlNet combinations, or refine existing images. Businesses could leverage the model for tasks like product visualization, architectural rendering, or even custom content creation.
Things to try
One interesting aspect of the realvisxl-v3-multi-controlnet-lora
model is the ability to use up to three ControlNets simultaneously. This allows users to explore the interplay between different visual cues, such as depth, edges, and body poses, to create unique and compelling images. Experimenting with the various ControlNet conditioning strengths, starts, and ends can lead to a wide range of stylistic and compositional outcomes.
This summary was produced with help from an AI and may contain inaccuracies - check out the links to read the original source documents!
Related Models
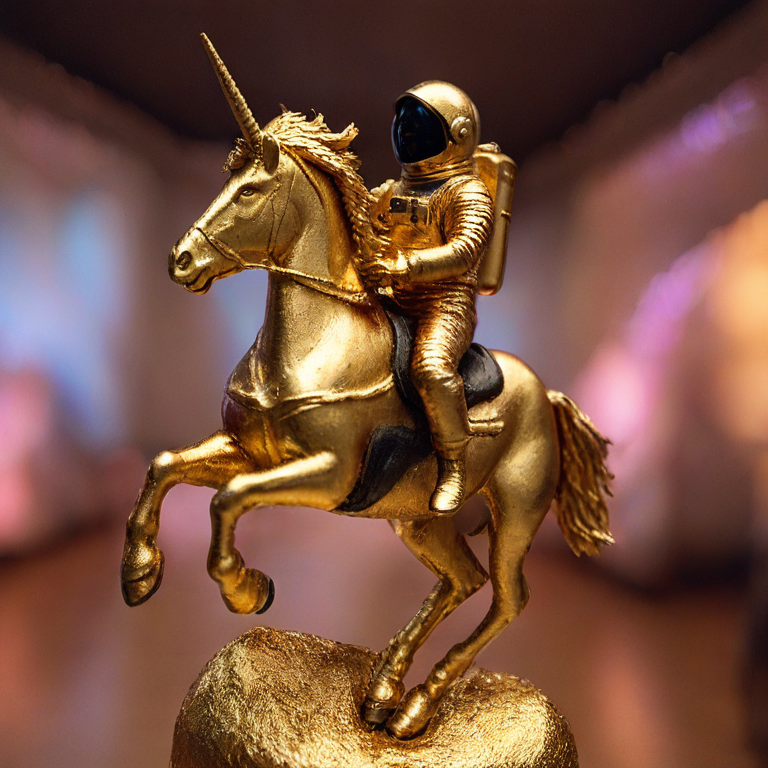
sdxl-multi-controlnet-lora

171
The sdxl-multi-controlnet-lora model, created by the Replicate user fofr, is a powerful image generation model that combines the capabilities of SDXL (Stable Diffusion XL) with multi-controlnet and LoRA (Low-Rank Adaptation) loading. This model offers a range of features, including img2img, inpainting, and the ability to use up to three simultaneous controlnets with different input images. It can be considered similar to other models like realvisxl-v3-multi-controlnet-lora, sdxl-controlnet-lora, and instant-id-multicontrolnet, all of which leverage the power of controlnets and LoRA to enhance image generation capabilities. Model inputs and outputs The sdxl-multi-controlnet-lora model accepts a variety of inputs, including an image, a mask for inpainting, a prompt, and various parameters to control the generation process. The model can output up to four images based on the input, with the ability to resize the output images to a specified width and height. Some key inputs and outputs include: Inputs Image**: Input image for img2img or inpaint mode Mask**: Input mask for inpaint mode, with black areas preserved and white areas inpainted Prompt**: Input prompt to guide the image generation Controlnet 1-3 Images**: Input images for up to three simultaneous controlnets Controlnet 1-3 Conditioning Scale**: Controls the strength of the controlnet conditioning Controlnet 1-3 Start/End**: Controls when the controlnet conditioning starts and ends Outputs Output Images**: Up to four generated images based on the input Capabilities The sdxl-multi-controlnet-lora model excels at generating high-quality, diverse images by leveraging the power of multiple controlnets and LoRA. It can seamlessly blend different input images and prompts to create unique and visually stunning outputs. The model's ability to handle inpainting and img2img tasks further expands its versatility, making it a valuable tool for a wide range of image-related applications. What can I use it for? The sdxl-multi-controlnet-lora model can be used for a variety of creative and practical applications. For example, it could be used to generate concept art, product visualizations, or personalized images for marketing materials. The model's inpainting and img2img capabilities also make it suitable for tasks like image restoration, object removal, and photo manipulation. Additionally, the multi-controlnet feature allows for the creation of highly detailed and context-specific images, making it a powerful tool for educational, scientific, or industrial applications that require precise visual representations. Things to try One interesting aspect of the sdxl-multi-controlnet-lora model is the ability to experiment with the different controlnet inputs and conditioning scales. By leveraging a variety of controlnet images, such as Canny edges, depth maps, or pose information, users can explore how the model blends and integrates these visual cues to generate unique and compelling outputs. Additionally, adjusting the controlnet conditioning scales can help users find the optimal balance between the input image and the generated output, allowing for fine-tuned control over the final result.
Updated Invalid Date
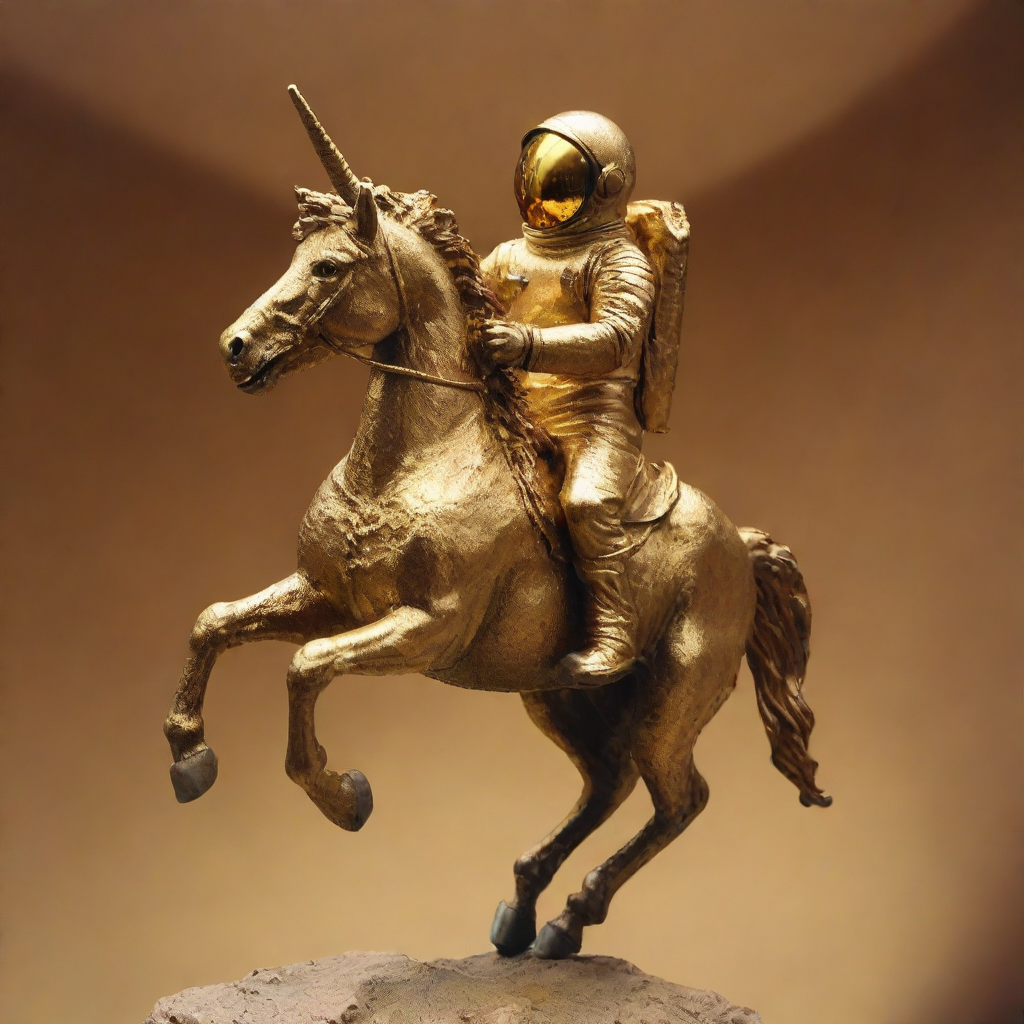
sdxl-lcm-multi-controlnet-lora

5
The sdxl-lcm-multi-controlnet-lora model is a powerful AI model developed by fofr that combines several advanced techniques for generating high-quality images. This model builds upon the SDXL architecture and incorporates LCM (Latent Classifier Guidance) lora for a significant speed increase, as well as support for multi-controlnet, img2img, and inpainting capabilities. Similar models in this ecosystem include the sdxl-multi-controlnet-lora and sdxl-lcm-lora-controlnet models, which also leverage SDXL, ControlNet, and LoRA techniques for image generation. Model Inputs and Outputs The sdxl-lcm-multi-controlnet-lora model accepts a variety of inputs, including a prompt, an optional input image for img2img or inpainting, and up to three different control images for the multi-controlnet functionality. The model can generate multiple output images based on the provided inputs. Inputs Prompt**: The text prompt that describes the desired image. Image**: An optional input image for img2img or inpainting tasks. Mask**: An optional mask image for inpainting, where black areas will be preserved and white areas will be inpainted. Controlnet 1-3 Images**: Up to three different control images that can be used to guide the image generation process. Outputs Images**: The model outputs one or more generated images based on the provided inputs. Capabilities The sdxl-lcm-multi-controlnet-lora model offers several advanced capabilities for image generation. It can perform both text-to-image and image-to-image tasks, including inpainting. The multi-controlnet functionality allows the model to incorporate up to three different control images, such as depth maps, edge maps, or pose information, to guide the generation process. What Can I Use It For? The sdxl-lcm-multi-controlnet-lora model can be a valuable tool for a variety of applications, from digital art and creative projects to product mockups and visualization tasks. Its ability to blend multiple control inputs and generate high-quality images makes it a versatile choice for professionals and hobbyists alike. Things to Try One interesting aspect of the sdxl-lcm-multi-controlnet-lora model is its ability to blend multiple control inputs, allowing you to experiment with different combinations of cues to generate unique and creative images. Try using different control images, such as depth maps, edge maps, or pose information, to see how they influence the output. Additionally, you can adjust the conditioning scales for each controlnet to find the right balance between the control inputs and the text prompt.
Updated Invalid Date
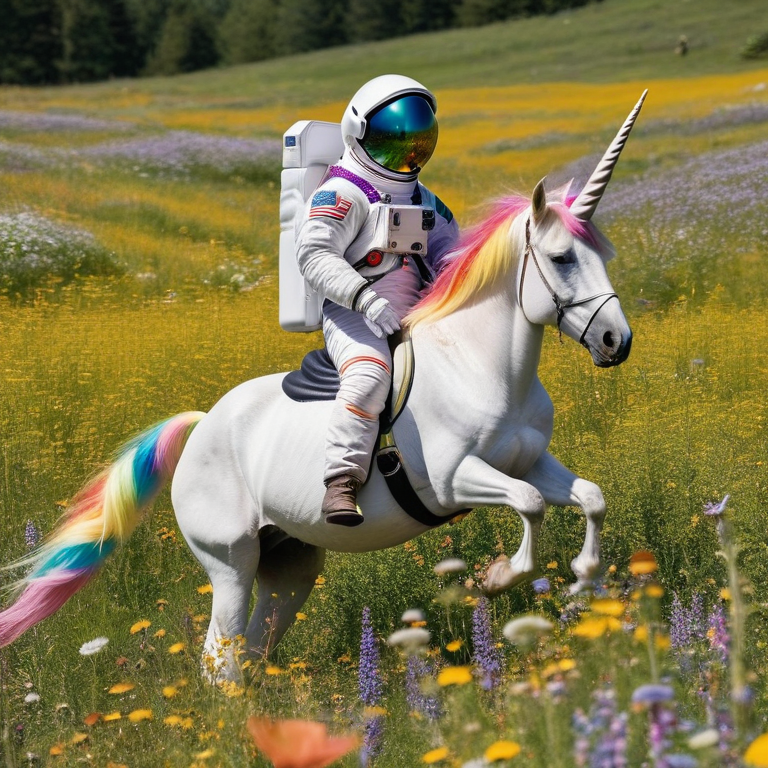
realvisxl-v3

397
The realvisxl-v3 is an advanced AI model developed by fofr that aims to produce highly photorealistic images. It is based on the SDXL (Stable Diffusion XL) model and has been further tuned for enhanced realism. This model can be contrasted with similar offerings like realvisxl-v3.0-turbo, realvisxl4, and realvisxl-v3-multi-controlnet-lora, which also target photorealism but with different approaches and capabilities. Model inputs and outputs The realvisxl-v3 model accepts a variety of inputs, including text prompts, images, and optional parameters like seed, guidance scale, and number of inference steps. The model can then generate one or more output images based on the provided inputs. Inputs Prompt**: The text prompt that describes the desired image to be generated. Negative prompt**: An optional text prompt that describes elements that should be excluded from the generated image. Image**: An optional input image that can be used for image-to-image or inpainting tasks. Mask**: An optional input mask that can be used for inpainting tasks, where black areas will be preserved and white areas will be inpainted. Seed**: An optional random seed value to ensure reproducible results. Width and height**: The desired width and height of the output image. Outputs Generated image(s)**: One or more images generated based on the provided inputs. Capabilities The realvisxl-v3 model is capable of producing highly realistic and photorealistic images based on text prompts. It can handle a wide range of subject matter, from landscapes and portraits to fantastical scenes. The model's tuning for realism results in outputs that are often indistinguishable from real photographs. What can I use it for? The realvisxl-v3 model can be a valuable tool for a variety of applications, such as digital art creation, content generation for marketing and advertising, and visual prototyping for product design. Its ability to generate photorealistic images can be particularly useful for projects that require high-quality visual assets, like virtual reality environments, movie and game assets, and product visualizations. Things to try One interesting aspect of the realvisxl-v3 model is its ability to handle a wide range of subject matter, from realistic scenes to more fantastical elements. You could try experimenting with different prompts that combine realistic and imaginative elements, such as "a photo of a futuristic city with flying cars" or "a portrait of a mythical creature in a realistic setting." The model's tuning for realism can produce some surprising and captivating results in these types of prompts.
Updated Invalid Date
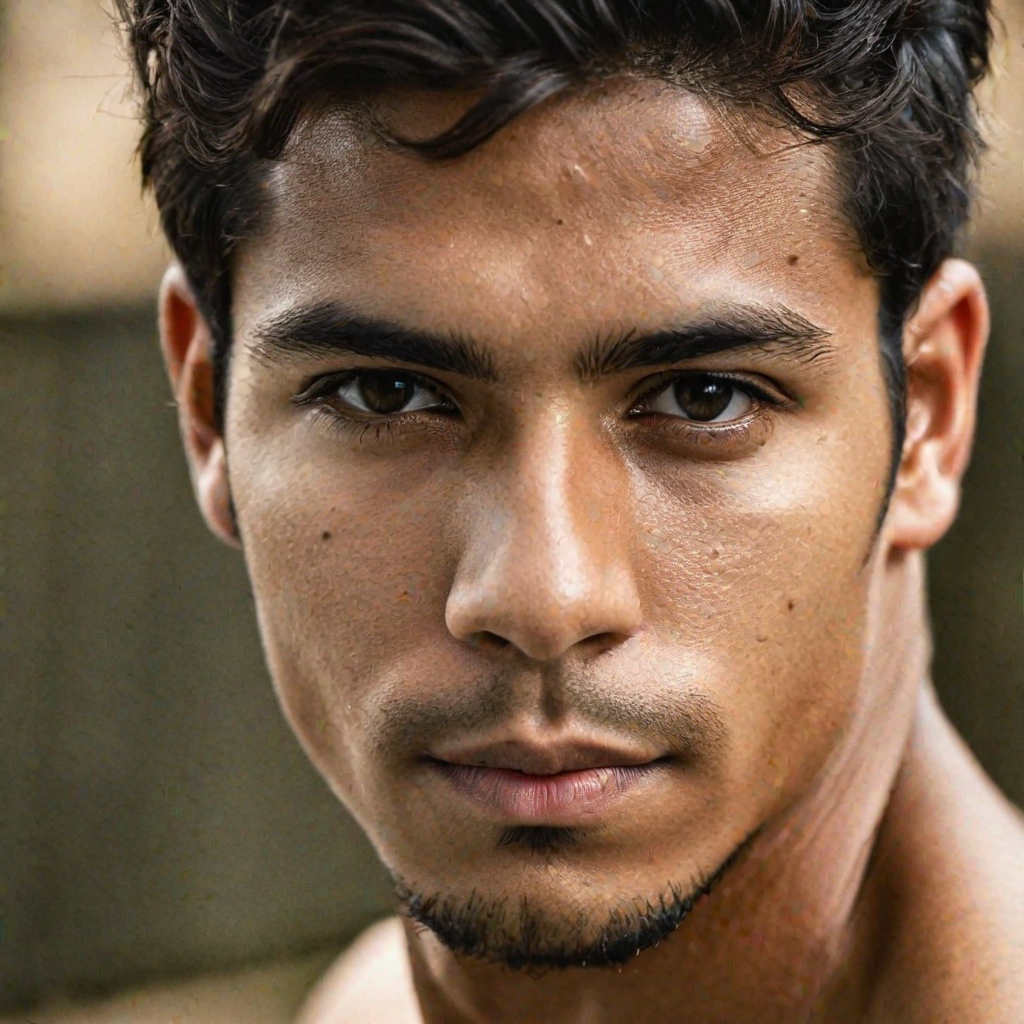
realvisxl2-lora-inference

2
The realvisxl2-lora-inference model is a proof of concept (POC) implementation by lucataco to run inference on the SG161222/RealVisXL_V2.0 model using Cog. Cog is a framework for packaging machine learning models as standard containers. This model is similar to other LoRA (Low-Rank Adaptation) models created by lucataco, such as the ssd-lora-inference, realvisxl2-lcm, realvisxl-v2.0, realvisxl-v2-img2img, and realvisxl-v1-img2img models. Model inputs and outputs The realvisxl2-lora-inference model takes in a prompt, an optional input image, and various parameters to control the image generation process. The model outputs one or more generated images. Inputs Prompt**: The input text prompt to guide the image generation. Lora URL**: The URL of the LoRA model to load. Scheduler**: The scheduler algorithm to use for image generation. Guidance Scale**: The scale for classifier-free guidance. Num Inference Steps**: The number of denoising steps to perform. Width/Height**: The desired width and height of the output image. Num Outputs**: The number of images to generate. Prompt Strength**: The strength of the prompt when using img2img or inpaint modes. Refine**: The type of refiner to use for the generated image. High Noise Frac**: The fraction of noise to use for the expert_ensemble_refiner. Refine Steps**: The number of refine steps to perform. Lora Scale**: The LoRA additive scale. Apply Watermark**: Whether to apply a watermark to the generated image. Outputs Output Images**: One or more generated images, returned as image URLs. Capabilities The realvisxl2-lora-inference model is capable of generating photorealistic images based on input text prompts. It can be used for a variety of creative and visual tasks, such as generating concept art, product renderings, and illustrations. What can I use it for? The realvisxl2-lora-inference model can be used for a variety of creative and visual tasks, such as: Generating concept art or illustrations for product design, marketing, or entertainment. Creating product renderings for e-commerce or visual development. Exploring visual ideas and scenarios based on text prompts. Experimenting with different prompts and parameters to discover novel image generation results. Things to try Some ideas for things to try with the realvisxl2-lora-inference model: Experiment with different prompts and parameters to see how they affect the generated images. Try using the model in conjunction with other image editing or manipulation tools to further refine the results. Explore the model's capabilities for generating images of specific subjects, scenes, or styles. Compare the outputs of the realvisxl2-lora-inference model to those of other similar models, such as the ones created by lucataco, to understand their strengths and differences.
Updated Invalid Date