sticker-maker
Maintainer: fofr

257
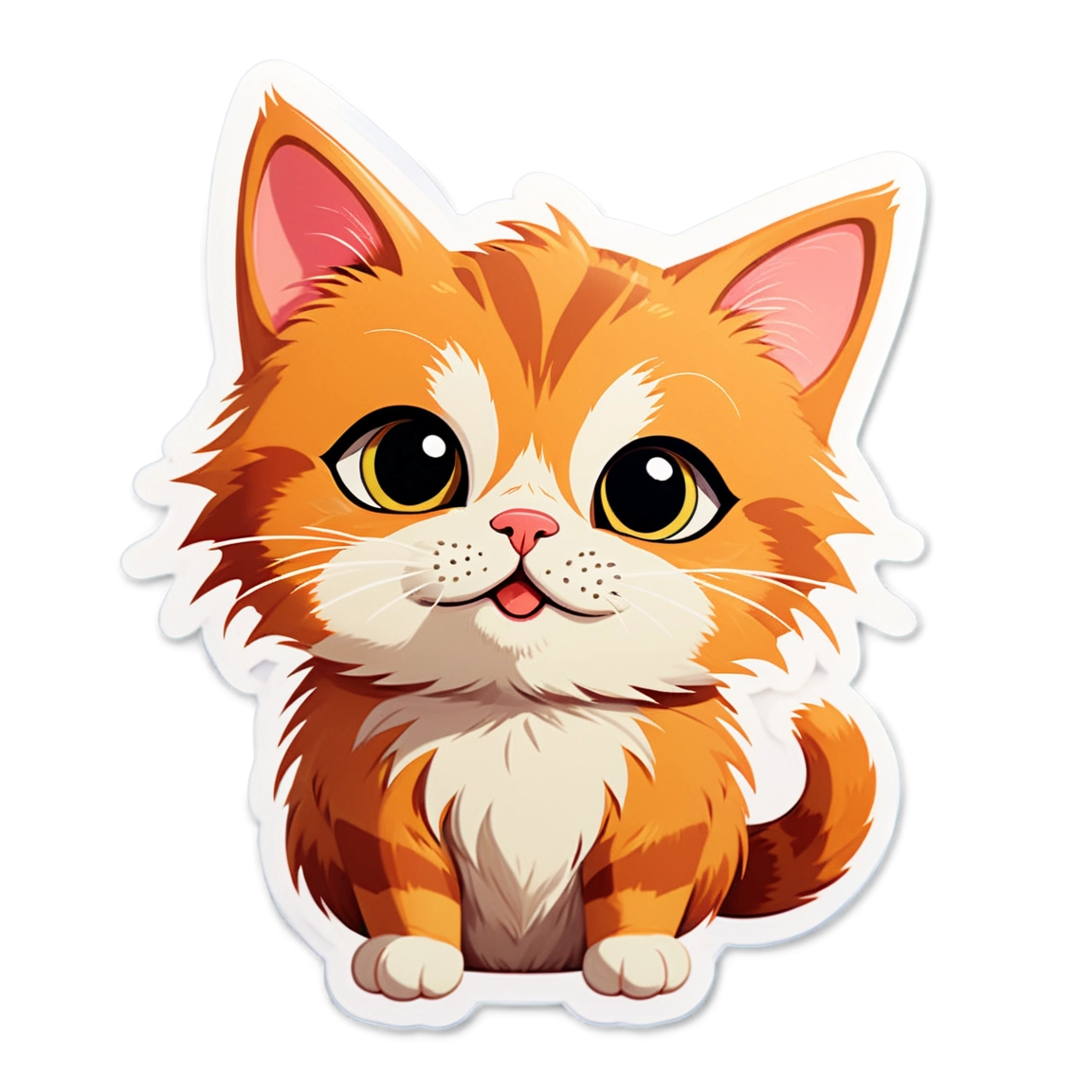
Property | Value |
---|---|
Model Link | View on Replicate |
API Spec | View on Replicate |
Github Link | View on Github |
Paper Link | No paper link provided |
Get summaries of the top AI models delivered straight to your inbox:
Model overview
The sticker-maker
model is a powerful AI tool that enables users to generate high-quality graphics with transparent backgrounds, making it an ideal solution for creating custom stickers. Compared to similar models like AbsoluteReality V1.8.1, Reliberate v3, and any-comfyui-workflow, the sticker-maker
model offers a streamlined and user-friendly interface, allowing users to quickly and easily create unique sticker designs.
Model inputs and outputs
The sticker-maker
model takes a variety of inputs, including a seed for reproducibility, the number of steps to use, the desired width and height of the output images, a prompt to guide the generation, a negative prompt to exclude certain elements, the output format, and the desired quality of the output images. The model then generates one or more images with transparent backgrounds, which can be used to create custom stickers.
Inputs
- Seed: Fix the random seed for reproducibility
- Steps: The number of steps to use in the generation process
- Width: The desired width of the output images
- Height: The desired height of the output images
- Prompt: The text prompt used to guide the generation
- Negative Prompt: Specify elements to exclude from the generated images
- Output Format: The format of the output images (e.g., WEBP)
- Output Quality: The quality of the output images, from 0 to 100 (100 is best)
- Number of Images: The number of images to generate
Outputs
- Array of image URLs: The generated images with transparent backgrounds, which can be used to create custom stickers
Capabilities
The sticker-maker
model is capable of generating a wide variety of sticker designs, ranging from cute and whimsical to more abstract and artistic. By adjusting the input prompts and settings, users can create stickers that fit their specific needs and preferences.
What can I use it for?
The sticker-maker
model is a versatile tool that can be used for a variety of applications, such as creating custom stickers for personal use, selling on platforms like Etsy, or incorporating into larger design projects. The transparent backgrounds of the generated images make them easy to incorporate into various designs and layouts.
Things to try
To get the most out of the sticker-maker
model, you can experiment with different input prompts and settings to see how they affect the generated stickers. Try prompts that evoke specific moods or styles, or mix and match different elements to create unique designs. You can also try generating multiple stickers and selecting the ones that best fit your needs.
This summary was produced with help from an AI and may contain inaccuracies - check out the links to read the original source documents!
Related Models
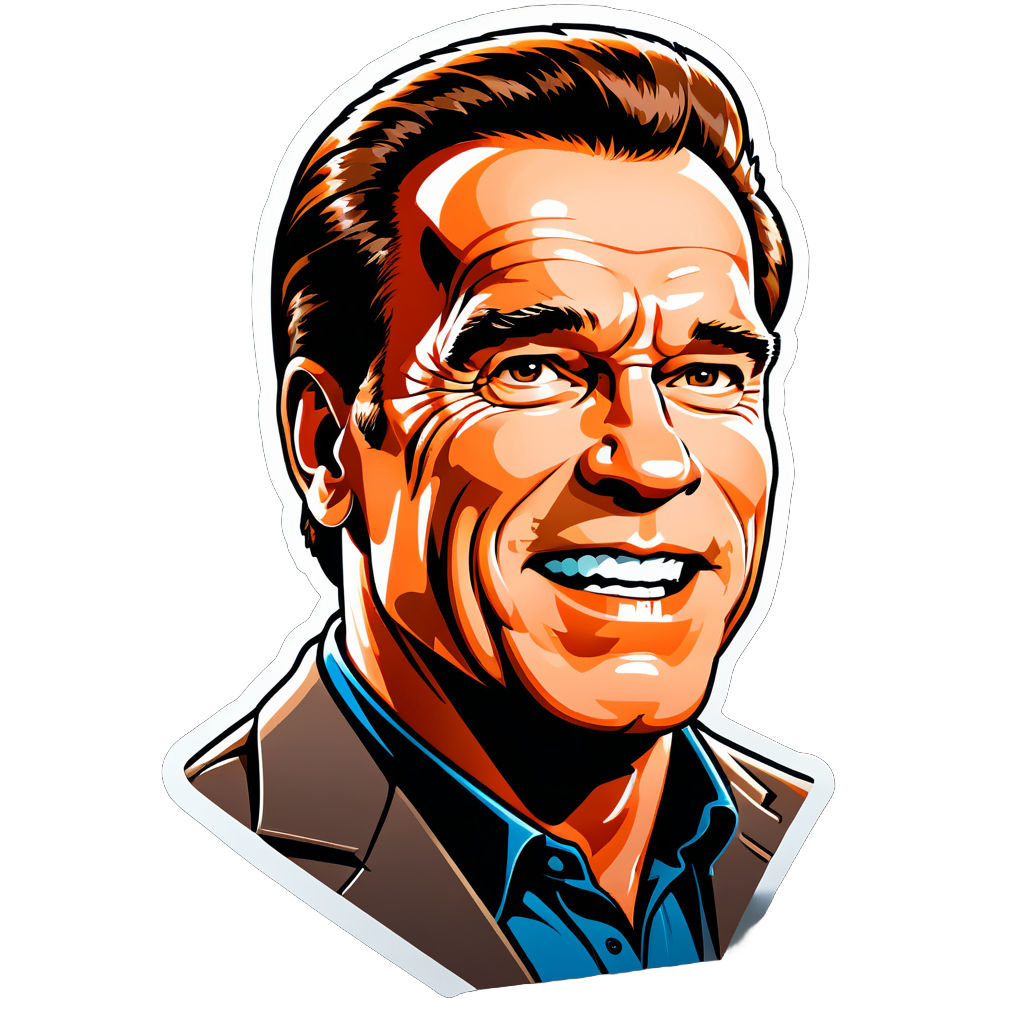
face-to-sticker

789
The face-to-sticker model is a tool that allows you to turn any face into a sticker. This model is created by the Replicate user fofr, who has also developed similar AI models like sticker-maker, face-to-many, and become-image. These models all focus on transforming faces into different visual styles using AI. Model inputs and outputs The face-to-sticker model takes an image of a person's face as input and generates a sticker-like output. You can also customize the model's output by adjusting parameters like the prompt, steps, width, height, and more. Inputs Image**: An image of a person's face to be converted into a sticker Prompt**: A text description of what you want the sticker to look like (default is "a person") Negative prompt**: Things you do not want to see in the sticker Prompt strength**: The strength of the prompt, with higher numbers leading to a stronger influence Steps**: The number of steps to take when generating the sticker Width and height**: The size of the output sticker Seed**: A number to fix the random seed for reproducibility Upscale**: Whether to upscale the sticker by 2x Upscale steps**: The number of steps to take when upscaling the sticker Ip adapter noise and weight**: Parameters that control the influence of the IP adapter on the final sticker Outputs The generated sticker image Capabilities The face-to-sticker model can take any face and transform it into a unique sticker-like image. This can be useful for creating custom stickers, emojis, or other graphics for social media, messaging, or other applications. What can I use it for? You can use the face-to-sticker model to create custom stickers and graphics for a variety of purposes, such as: Personalizing your messaging and social media with unique, AI-generated stickers Designing custom merchandise or products with your own face or the faces of others Experimenting with different visual styles and effects to create new and interesting graphics Things to try One interesting thing to try with the face-to-sticker model is to experiment with different prompts and parameters to see how they affect the final sticker. Try prompts that evoke different moods, emotions, or visual styles, and see how the model responds. You can also play with the upscaling and IP adapter settings to create more detailed or stylized stickers.
Updated Invalid Date
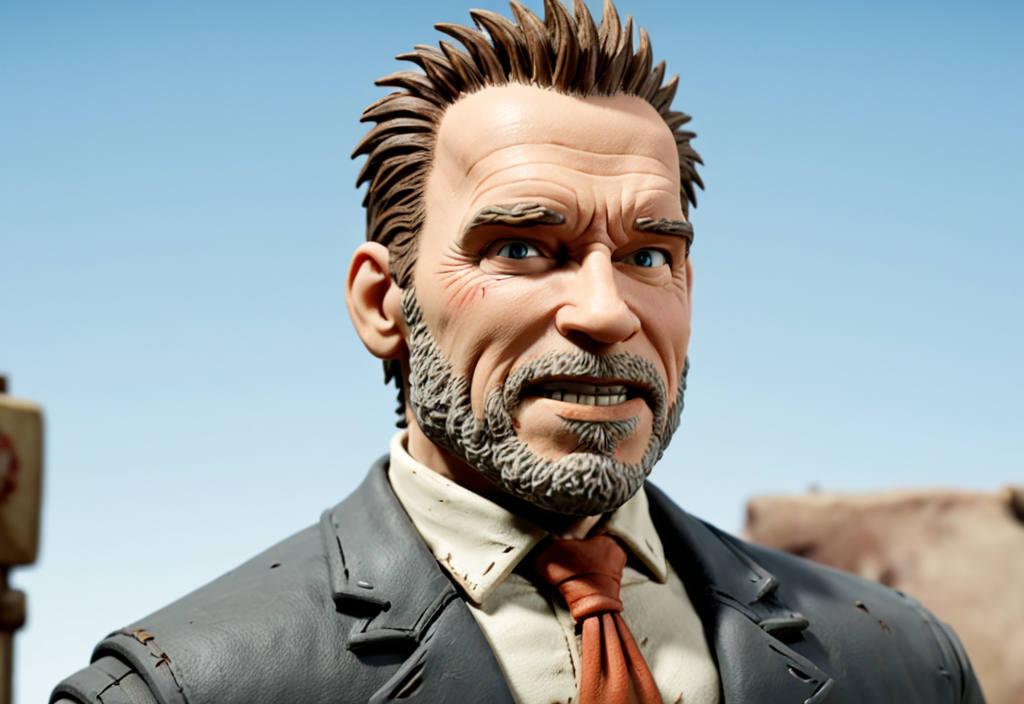
face-to-many

11.9K
The face-to-many model is a versatile AI tool that allows you to turn any face into a variety of artistic styles, such as 3D, emoji, pixel art, video game, claymation, or toy. Developed by fofr, this model is part of a larger collection of creative AI tools from the Replicate platform. Similar models include sticker-maker for generating stickers with transparent backgrounds, real-esrgan for high-quality image upscaling, and instant-id for creating realistic images of people. Model inputs and outputs The face-to-many model takes in an image of a person's face and a target style, allowing you to transform the face into a range of artistic representations. The model outputs an array of generated images in the selected style. Inputs Image**: An image of a person's face to be transformed Style**: The desired artistic style to apply, such as 3D, emoji, pixel art, video game, claymation, or toy Prompt**: A text description to guide the image generation (default is "a person") Negative Prompt**: Text describing elements you don't want in the image Prompt Strength**: The strength of the prompt, with higher numbers leading to a stronger influence Denoising Strength**: How much of the original image to keep, with 1 being a complete destruction and 0 being the original Instant ID Strength**: The strength of the InstantID model used for facial recognition Control Depth Strength**: The strength of the depth controlnet, affecting how much it influences the output Seed**: A fixed random seed for reproducibility Custom LoRA URL**: An optional URL to a custom LoRA (Learned Residual Adapter) model LoRA Scale**: The strength of the custom LoRA model Outputs An array of generated images in the selected artistic style Capabilities The face-to-many model excels at transforming faces into a wide range of artistic styles, from the detailed 3D rendering to the whimsical pixel art or claymation. The model's ability to capture the essence of the original face while applying these unique styles makes it a powerful tool for creative projects, digital art, and even product design. What can I use it for? With the face-to-many model, you can create unique and eye-catching visuals for a variety of applications, such as: Generating custom avatars or character designs for video games, apps, or social media Producing stylized portraits or profile pictures with a distinctive flair Designing fun and engaging stickers, emojis, or other digital assets Prototyping physical products like toys, figurines, or collectibles Exploring creative ideas and experimenting with different artistic interpretations of a face Things to try The face-to-many model offers a wide range of possibilities for creative experimentation. Try combining different styles, adjusting the input parameters, or using custom LoRA models to see how the output can be further tailored to your specific needs. Explore the limits of the model's capabilities and let your imagination run wild!
Updated Invalid Date
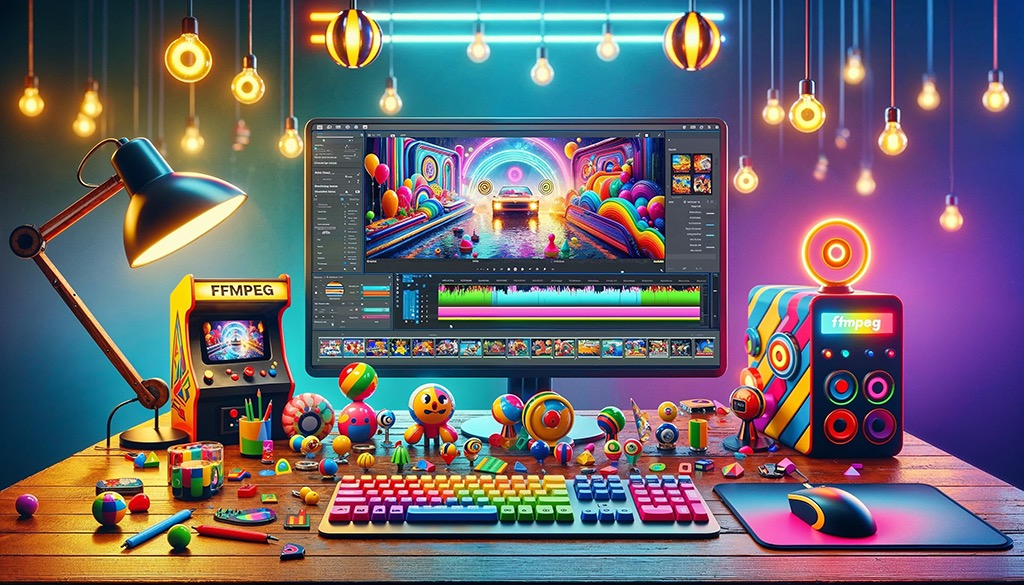
toolkit

2
The toolkit model is a versatile video processing tool created by Replicate developer fofr. It can perform a variety of common video tasks, such as converting videos to MP4 format, creating GIFs from videos, extracting audio from videos, and converting a folder of frames into a video or GIF. This model is a helpful CPU-based tool that wraps common FFmpeg tasks, making it easy to perform common video manipulations. It can be particularly useful for tasks like creating web content, making video assets for social media, or preparing video files for further editing. The toolkit model complements other video-focused models created by fofr, like the sticker-maker, face-to-many, and become-image models. Model inputs and outputs The toolkit model accepts a variety of input files, including videos, GIFs, and zipped folders of frames. Users can specify the desired task, such as converting to MP4, creating a GIF, or extracting audio. They can also adjust the frames per second (FPS) of the output, with the default setting keeping the original FPS or using 12 FPS for GIFs. Inputs Task**: The specific operation to perform, such as converting to MP4, creating a GIF, or extracting audio Input File**: The video, GIF, or zipped folder of frames to be processed FPS**: The frames per second for the output (0 keeps the original FPS, or defaults to 12 FPS for GIFs) Outputs The processed video or audio file, returned as a URI Capabilities The toolkit model can handle a wide range of common video tasks, making it a versatile tool for content creators and video editors. It can convert videos to MP4 format, create GIFs from videos, extract audio from videos, and even convert a zipped folder of frames into a video or GIF. This allows users to quickly and easily prepare video assets for a variety of purposes, from social media content to video editing projects. What can I use it for? The toolkit model is well-suited for a variety of video-related tasks. Content creators can use it to convert video files for easy sharing on social media platforms or websites. Video editors can leverage it to extract audio from footage or convert a series of images into a video or GIF. Businesses may find it useful for preparing video assets for marketing campaigns or client presentations. The model's ability to handle common video manipulations in a straightforward manner makes it a valuable tool for a wide range of video-centric workflows. Things to try One interesting use case for the toolkit model is processing a zipped folder of frames into a video or GIF. This could be useful for animators or designers who need to create short animated sequences from a series of individual images. The model's flexibility in handling different input formats and output specifications makes it a versatile tool for a variety of video-related projects.
Updated Invalid Date
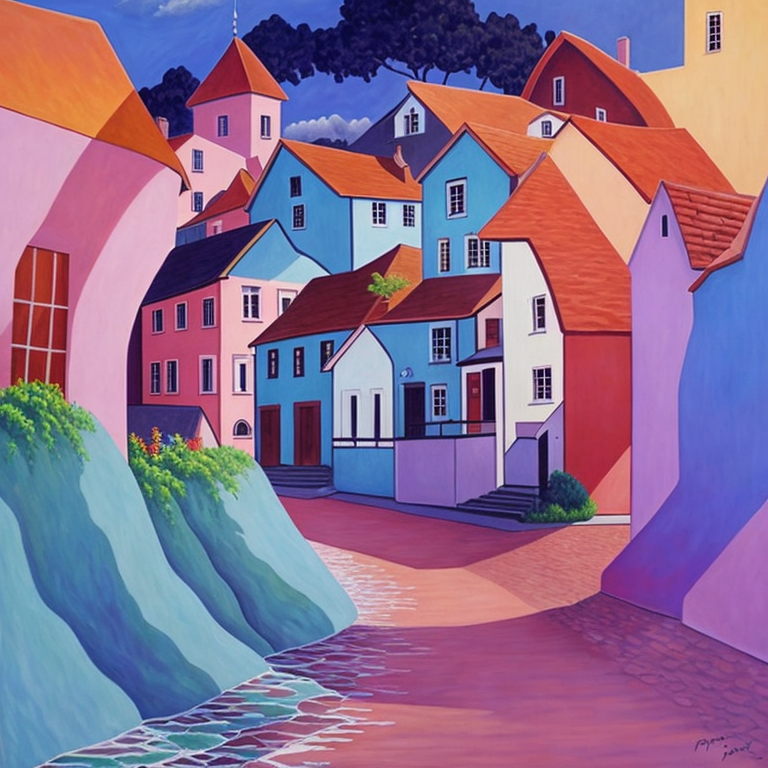
illusions

4
The illusions model is a Cog implementation of the Monster Labs' QR code control net that allows users to create visual illusions using img2img and masking support. This model is part of a collection of AI models created by fofr, who has also developed similar models like become-image, image-merger, sticker-maker, image-merge-sdxl, and face-to-many. Model inputs and outputs The illusions model allows users to generate images that create visual illusions. The model takes in a prompt, an optional input image for img2img, an optional mask image for inpainting, and a control image. It also allows users to specify various parameters like the seed, width, height, number of outputs, guidance scale, negative prompt, prompt strength, and controlnet conditioning. Inputs Prompt**: The text prompt that guides the image generation. Image**: An optional input image for img2img. Mask Image**: An optional mask image for inpainting. Control Image**: An optional control image. Seed**: The seed to use for reproducible image generation. Width**: The width of the generated image. Height**: The height of the generated image. Num Outputs**: The number of output images to generate. Guidance Scale**: The scale for classifier-free guidance. Negative Prompt**: The negative prompt to guide image generation. Prompt Strength**: The strength of the prompt when using img2img or inpainting. Sizing Strategy**: How to resize images, such as using the width/height, resizing based on the input image, or resizing based on the control image. Controlnet Start**: When the controlnet conditioning starts. Controlnet End**: When the controlnet conditioning ends. Controlnet Conditioning Scale**: How strong the controlnet conditioning is. Outputs Output Images**: An array of generated image URLs. Capabilities The illusions model can generate a variety of visual illusions, such as optical illusions, trick art, and other types of mind-bending imagery. By using the img2img and masking capabilities, users can create unique and surprising effects by combining existing images with the model's generative abilities. What can I use it for? The illusions model could be used for a range of applications, such as creating unique artwork, designing optical illusion-based posters or graphics, or even generating visuals for interactive entertainment experiences. The model's ability to work with existing images makes it a versatile tool for both professional and amateur creators looking to add a touch of visual trickery to their projects. Things to try One interesting thing to try with the illusions model is to experiment with using different control images and see how they affect the generated illusions. You could also try using the img2img and masking capabilities to transform existing images in unexpected ways, or to combine multiple images to create more complex visual effects.
Updated Invalid Date