gpt-j-6b
Maintainer: replicate

8
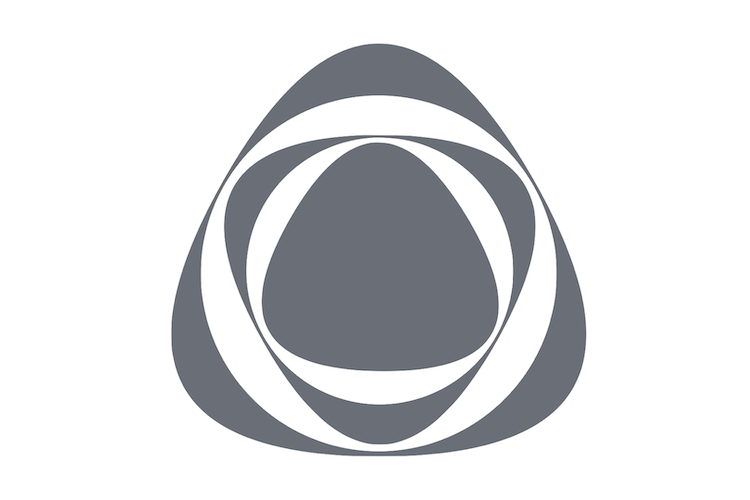
Property | Value |
---|---|
Model Link | View on Replicate |
API Spec | View on Replicate |
Github Link | View on Github |
Paper Link | No paper link provided |
Get summaries of the top AI models delivered straight to your inbox:
Model overview
gpt-j-6b
is a large language model developed by EleutherAI, a non-profit AI research group. It is a fine-tunable model that can be adapted for a variety of natural language processing tasks. Compared to similar models like stable-diffusion, flan-t5-xl, and llava-13b, gpt-j-6b
is specifically designed for text generation and language understanding.
Model inputs and outputs
The gpt-j-6b
model takes a text prompt as input and generates a completion in the form of more text. The model can be fine-tuned on a specific dataset, allowing it to adapt to various tasks like question answering, summarization, and creative writing.
Inputs
- Prompt: The initial text that the model will use to generate a completion.
Outputs
- Completion: The text generated by the model based on the input prompt.
Capabilities
gpt-j-6b
is capable of generating human-like text across a wide range of domains, from creative writing to task-oriented dialog. It can be used for tasks like summarization, translation, and open-ended question answering. The model's performance can be further improved through fine-tuning on specific datasets.
What can I use it for?
The gpt-j-6b
model can be used for a variety of applications, such as:
- Content Generation: Generating high-quality text for articles, stories, scripts, and more.
- Chatbots and Virtual Assistants: Building conversational AI systems that can engage in natural dialogue.
- Question Answering: Answering open-ended questions by retrieving and synthesizing relevant information.
- Summarization: Condensing long-form text into concise summaries.
These capabilities make gpt-j-6b
a versatile tool for businesses, researchers, and developers looking to leverage advanced natural language processing in their projects.
Things to try
One interesting aspect of gpt-j-6b
is its ability to perform few-shot learning, where the model can quickly adapt to a new task or domain with only a small amount of fine-tuning data. This makes it a powerful tool for rapid prototyping and experimentation. You could try fine-tuning the model on your own dataset to see how it performs on a specific task or application.
This summary was produced with help from an AI and may contain inaccuracies - check out the links to read the original source documents!
Related Models
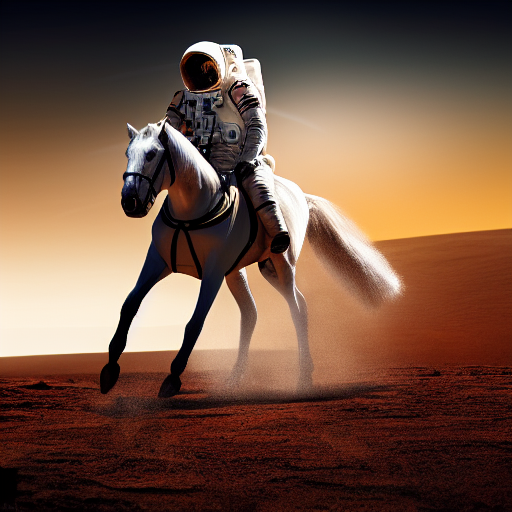
stable-diffusion

107.9K
Stable Diffusion is a latent text-to-image diffusion model capable of generating photo-realistic images given any text input. Developed by Stability AI, it is an impressive AI model that can create stunning visuals from simple text prompts. The model has several versions, with each newer version being trained for longer and producing higher-quality images than the previous ones. The main advantage of Stable Diffusion is its ability to generate highly detailed and realistic images from a wide range of textual descriptions. This makes it a powerful tool for creative applications, allowing users to visualize their ideas and concepts in a photorealistic way. The model has been trained on a large and diverse dataset, enabling it to handle a broad spectrum of subjects and styles. Model inputs and outputs Inputs Prompt**: The text prompt that describes the desired image. This can be a simple description or a more detailed, creative prompt. Seed**: An optional random seed value to control the randomness of the image generation process. Width and Height**: The desired dimensions of the generated image, which must be multiples of 64. Scheduler**: The algorithm used to generate the image, with options like DPMSolverMultistep. Num Outputs**: The number of images to generate (up to 4). Guidance Scale**: The scale for classifier-free guidance, which controls the trade-off between image quality and faithfulness to the input prompt. Negative Prompt**: Text that specifies things the model should avoid including in the generated image. Num Inference Steps**: The number of denoising steps to perform during the image generation process. Outputs Array of image URLs**: The generated images are returned as an array of URLs pointing to the created images. Capabilities Stable Diffusion is capable of generating a wide variety of photorealistic images from text prompts. It can create images of people, animals, landscapes, architecture, and more, with a high level of detail and accuracy. The model is particularly skilled at rendering complex scenes and capturing the essence of the input prompt. One of the key strengths of Stable Diffusion is its ability to handle diverse prompts, from simple descriptions to more creative and imaginative ideas. The model can generate images of fantastical creatures, surreal landscapes, and even abstract concepts with impressive results. What can I use it for? Stable Diffusion can be used for a variety of creative applications, such as: Visualizing ideas and concepts for art, design, or storytelling Generating images for use in marketing, advertising, or social media Aiding in the development of games, movies, or other visual media Exploring and experimenting with new ideas and artistic styles The model's versatility and high-quality output make it a valuable tool for anyone looking to bring their ideas to life through visual art. By combining the power of AI with human creativity, Stable Diffusion opens up new possibilities for visual expression and innovation. Things to try One interesting aspect of Stable Diffusion is its ability to generate images with a high level of detail and realism. Users can experiment with prompts that combine specific elements, such as "a steam-powered robot exploring a lush, alien jungle," to see how the model handles complex and imaginative scenes. Additionally, the model's support for different image sizes and resolutions allows users to explore the limits of its capabilities. By generating images at various scales, users can see how the model handles the level of detail and complexity required for different use cases, such as high-resolution artwork or smaller social media graphics. Overall, Stable Diffusion is a powerful and versatile AI model that offers endless possibilities for creative expression and exploration. By experimenting with different prompts, settings, and output formats, users can unlock the full potential of this cutting-edge text-to-image technology.
Updated Invalid Date
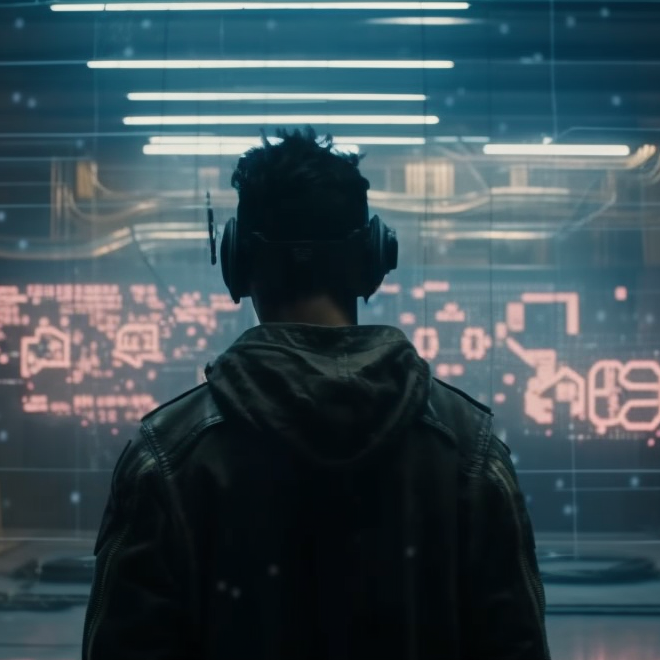
workgpt

1
workgpt is an AI model that helps with various work-related tasks. While it does not have a research paper abstract or detailed README, the maintainer's description indicates that it is designed to assist users with their work. Compared to similar models like exllama-airoboros-7b-gpt4-1.4-gptq, wizardcoder-34b-v1.0, and stable-diffusion, workgpt appears to have a more focused use case on work-related tasks. Model inputs and outputs workgpt takes in a variety of inputs to generate relevant outputs. These include the prompt, the number of output sequences to generate, the target temperature, the total number of tokens, and the repetition penalty. Inputs Prompt**: The text prompt to send to the LLaMA language model N**: The number of output sequences to generate, up to 5 Temperature**: Adjusts the randomness of the outputs, with higher values being more random Total Tokens**: The maximum number of tokens for the input and generation Repetition Penalty**: Adjusts the penalty for repeated words in the generated text Outputs Output**: An array of generated text sequences based on the provided inputs Capabilities workgpt can assist with a wide range of work-related tasks, such as writing, research, analysis, and task planning. It can generate text that is tailored to specific prompts and requirements, making it a useful tool for professionals in various industries. What can I use it for? You can use workgpt to help with tasks like drafting reports, creating presentations, brainstorming ideas, and summarizing research. It could be particularly useful for [Company Name] employees, as it can save time and improve the quality of their work outputs. The model's focus on work-related tasks sets it apart from more general-purpose language models. Things to try One interesting aspect of workgpt is its ability to generate text that is tailored to specific prompts and requirements. You could try providing it with detailed instructions or guidelines for a specific work task, and see how it responds. Additionally, experimenting with the different input parameters, such as temperature and repetition penalty, could yield interesting variations in the generated text.
Updated Invalid Date
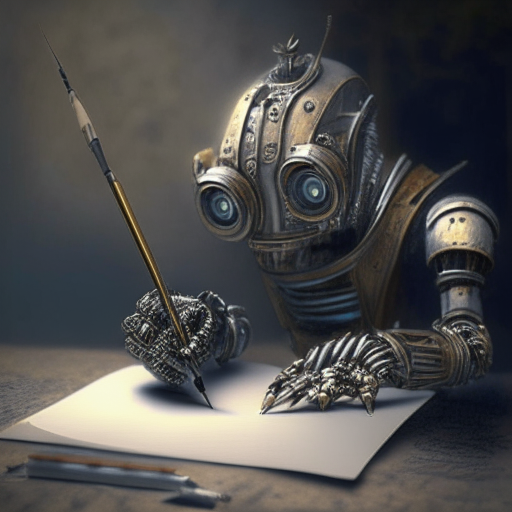
mpt-7b-storywriter

8
mpt-7b-storywriter is a 7 billion parameter language model fine-tuned by MosaicML to excel at generating long-form fictional stories. It was built by fine-tuning the MPT-7B model on a filtered subset of the books3 dataset, with a focus on stories. Unlike a standard language model, mpt-7b-storywriter can handle very long context lengths of up to 65,536 tokens thanks to the use of Attention with Linear Biases (ALiBi). MosaicML has demonstrated the model's ability to generate coherent stories with up to 84,000 tokens on a single node of 8 A100 GPUs. This model shares similarities with other large language models like LLAMA-7B and LLAMA-2-7B in terms of model size and architecture. However, mpt-7b-storywriter is specifically tailored for long-form story generation through its fine-tuning on fiction datasets and use of ALiBi. Model inputs and outputs Inputs Prompt**: The starting text to use as a prompt for the model to continue generating. Max Length**: The maximum number of tokens to generate. Temperature**: Controls the randomness of the generated text, with higher values producing more diverse and unpredictable output. Top P**: Limits the model to sampling from the top P% of the most likely tokens, reducing randomness. Repetition Penalty**: Discourages the model from repeating the same words or phrases. Length Penalty**: Adjusts the model's preference for generating longer or shorter sequences. Seed**: Sets a random seed for reproducible outputs. Debug**: Provides additional logging for debugging purposes. Outputs Generated Text**: The text generated by the model, continuing the provided prompt. Capabilities mpt-7b-storywriter excels at generating long-form, coherent fictional stories. It can maintain narrative consistency and flow over thousands of tokens, making it a powerful tool for creative writing tasks. The model's ability to handle extremely long context lengths sets it apart from standard language models, allowing for more immersive and engaging story generation. What can I use it for? mpt-7b-storywriter is well-suited for a variety of creative writing and storytelling applications. Writers and authors could use it to generate story ideas, plot outlines, or even full-length novels with the model's guidance. Content creators could leverage the model to produce engaging fiction for interactive experiences, games, or multimedia projects. Additionally, the model's capabilities could be harnessed for educational purposes, such as helping students with creative writing exercises or inspiring them to explore their own storytelling abilities. Things to try One interesting aspect of mpt-7b-storywriter is its ability to extrapolate beyond its training context length of 65,536 tokens. By adjusting the max_seq_len parameter in the model's configuration, you can experiment with generating even longer stories, potentially unlocking new narrative possibilities. Another avenue to explore is the model's behavior with different prompt styles or genres. Try providing it with various types of story starters, from fantasy epics to slice-of-life dramas, and observe how the generated content adapts to the specific narrative context.
Updated Invalid Date
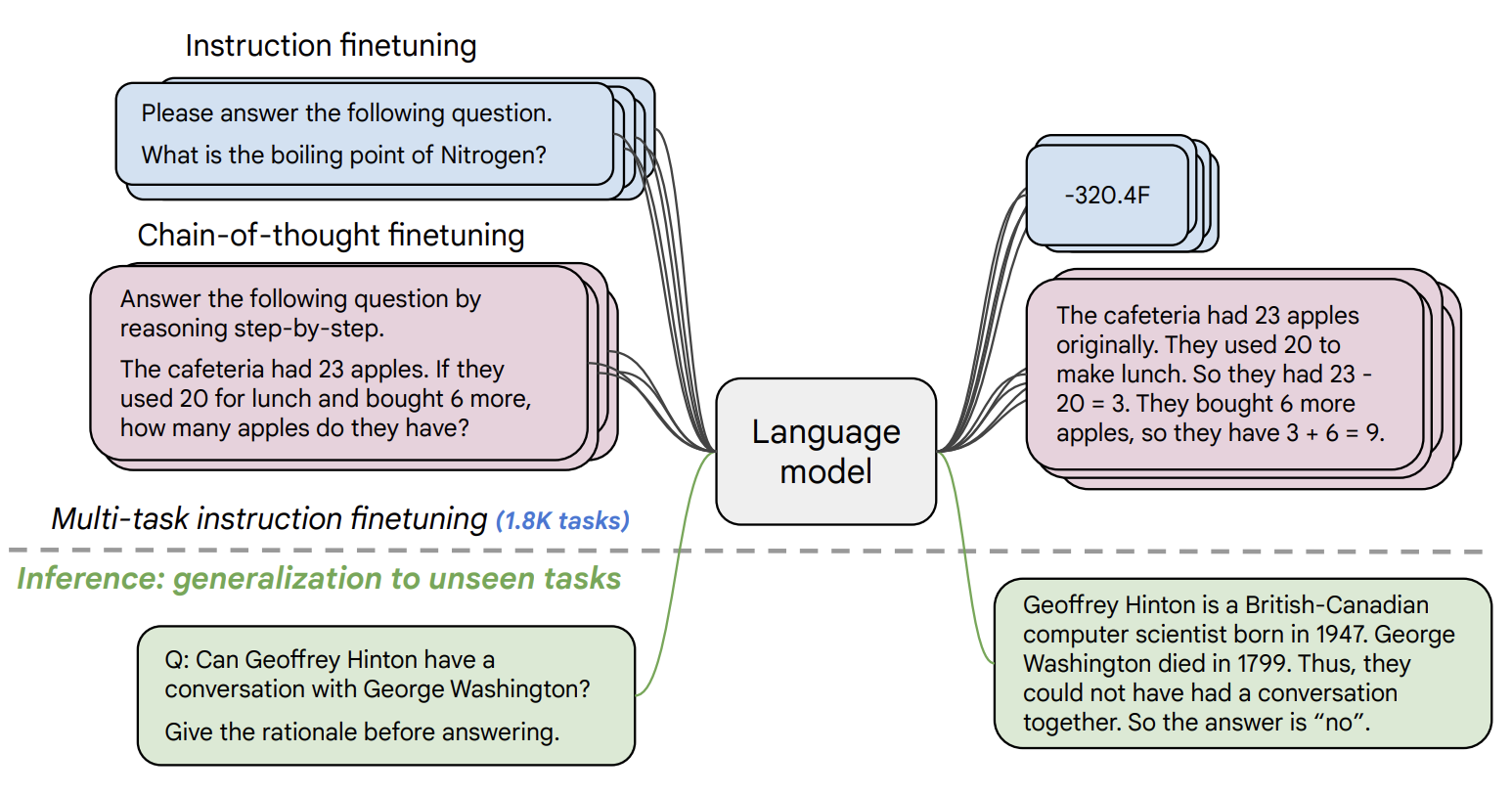
flan-t5-xl

134
flan-t5-xl is a large language model developed by Google that is based on the T5 model architecture. It is a "FLAN" (Finetuned Language Model) model, meaning it has been fine-tuned on a diverse set of over 1,000 tasks and datasets to improve its performance on a wide range of language understanding and generation tasks. The flan-t5-xl model is the extra-large variant, with more parameters than the standard T5 model. Similar models include the smaller flan-t5-large model and the even larger FLAN-T5-XXL model. There is also the multilingual multilingual-e5-large model which is designed for multi-language tasks. Model inputs and outputs The flan-t5-xl model takes in text prompts as input and generates text outputs. The model can be used for a variety of natural language processing tasks such as classification, summarization, translation, and more. Inputs prompt**: The text prompt to send to the FLAN-T5 model Outputs generated text**: The text generated by the model in response to the input prompt Capabilities flan-t5-xl is a highly capable language model that can perform a wide range of NLP tasks. It has been fine-tuned on over 1,000 different tasks and datasets, giving it broad competence. The model can excel at tasks like summarization, translation, question answering, and open-ended text generation. What can I use it for? The flan-t5-xl model could be used for a variety of applications that require natural language processing, such as: Content generation**: Use the model to generate human-like text for things like product descriptions, marketing copy, or creative writing. Summarization**: Leverage the model's summarization capabilities to automatically generate concise summaries of long documents or articles. Translation**: Fine-tune the model on translation data to create a multilingual language model that can translate between various languages. Question answering**: Use the model to build chatbots or virtual assistants that can understand and respond to user questions. Things to try One interesting aspect of the flan-t5-xl model is its strong few-shot learning performance. This means that it can often achieve good results on new tasks with just a handful of training examples, without requiring extensive fine-tuning. Experimenting with different prompting techniques and few-shot learning setups could yield some surprising and novel applications for the model. Another intriguing area to explore would be using the flan-t5-xl model in a multi-modal setting, combining its language understanding capabilities with visual or other modalities. This could unlock new ways of interacting with and reasoning about the world.
Updated Invalid Date