Tencentarc
Models by this creator
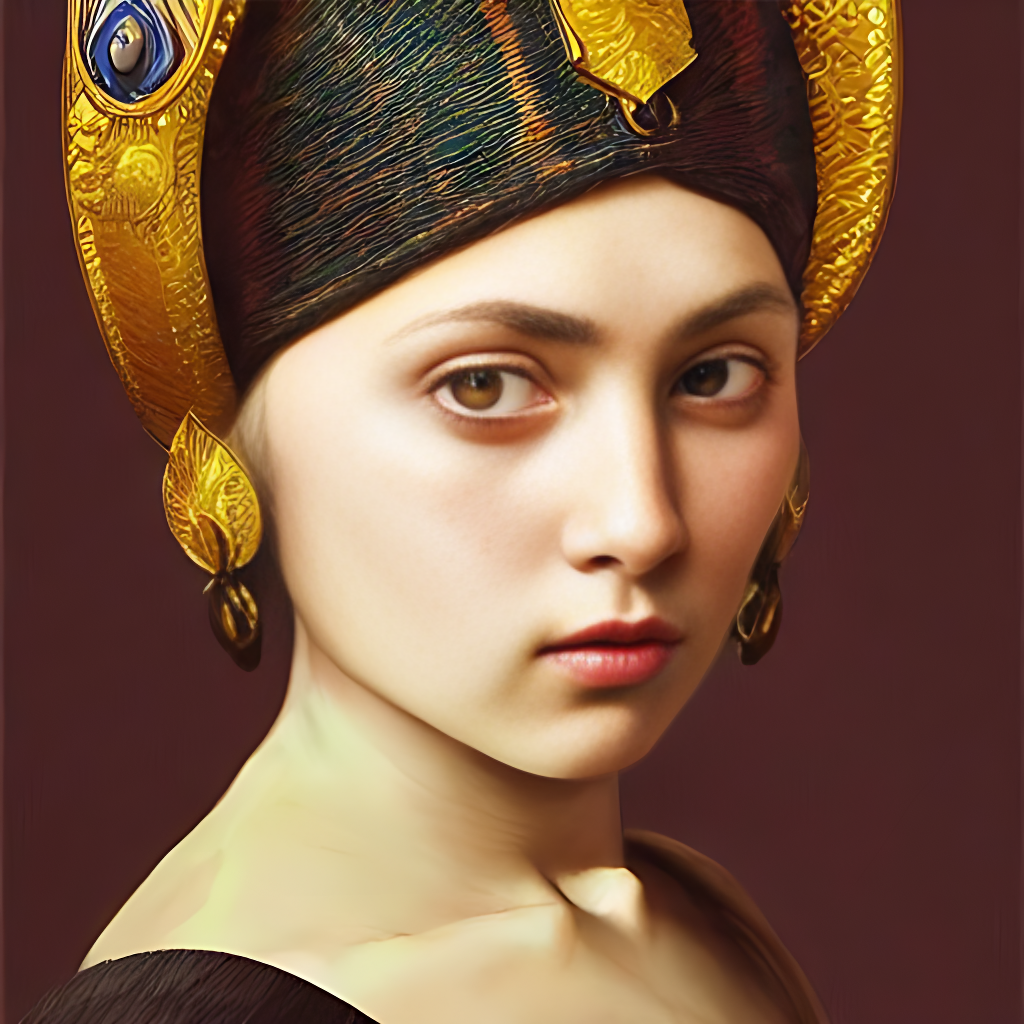
gfpgan

72.6K
gfpgan is a practical face restoration algorithm developed by the Tencent ARC team. It leverages the rich and diverse priors encapsulated in a pre-trained face GAN (such as StyleGAN2) to perform blind face restoration on old photos or AI-generated faces. This approach contrasts with similar models like Real-ESRGAN, which focuses on general image restoration, or PyTorch-AnimeGAN, which specializes in anime-style photo animation. Model inputs and outputs gfpgan takes an input image and rescales it by a specified factor, typically 2x. The model can handle a variety of face images, from low-quality old photos to high-quality AI-generated faces. Inputs Img**: The input image to be restored Scale**: The factor by which to rescale the output image (default is 2) Version**: The gfpgan model version to use (v1.3 for better quality, v1.4 for more details and better identity) Outputs Output**: The restored face image Capabilities gfpgan can effectively restore a wide range of face images, from old, low-quality photos to high-quality AI-generated faces. It is able to recover fine details, fix blemishes, and enhance the overall appearance of the face while preserving the original identity. What can I use it for? You can use gfpgan to restore old family photos, enhance AI-generated portraits, or breathe new life into low-quality images of faces. The model's capabilities make it a valuable tool for photographers, digital artists, and anyone looking to improve the quality of their facial images. Additionally, the maintainer tencentarc offers an online demo on Replicate, allowing you to try the model without setting up the local environment. Things to try Experiment with different input images, varying the scale and version parameters, to see how gfpgan can transform low-quality or damaged face images into high-quality, detailed portraits. You can also try combining gfpgan with other models like Real-ESRGAN to enhance the background and non-facial regions of the image.
Updated 4/28/2024
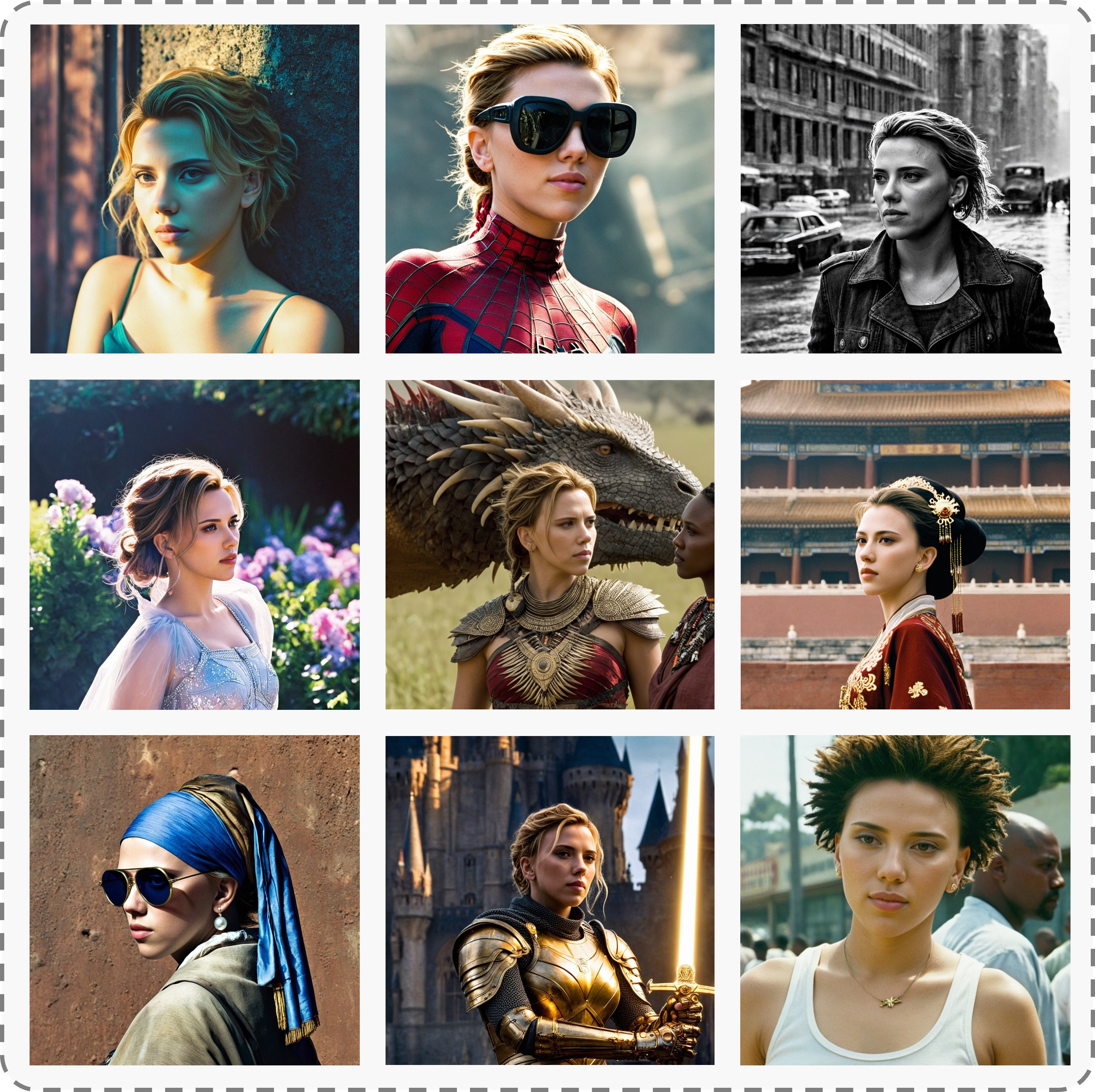
photomaker

1.1K
PhotoMaker is a text-to-image AI model developed by TencentARC that allows users to input one or a few face photos along with a text prompt to receive a customized photo or painting within seconds. The model can be adapted to any base model based on SDXL or used in conjunction with other LoRA modules. PhotoMaker produces both realistic and stylized results, as shown in the examples on the project page. Similar models include photomaker, GFPGAN, and PixArt-XL-2-1024-MS. Model inputs and outputs PhotoMaker takes one or more face photos and a text prompt as input, and generates a customized photo or painting as output. The model is capable of producing both realistic and stylized results, allowing users to experiment with different artistic styles. Inputs Face photos**: One or more face photos that the model can use to generate the customized image. Text prompt**: A description of the desired image, which the model uses to generate the output. Outputs Customized photo/painting**: The generated image, which can be either a realistic photo or a stylized painting, depending on the input prompt. Capabilities PhotoMaker is capable of generating high-quality, customized images from face photos and text prompts. The model can produce both realistic and stylized results, allowing users to explore different artistic styles. For example, the model can generate images of a person in a specific pose or setting, or it can create paintings in the style of a particular artist. What can I use it for? PhotoMaker can be used for a variety of creative and artistic projects. For example, you could use the model to generate personalized portraits, create concept art for a story or game, or experiment with different artistic styles. The model could also be integrated into educational or creative tools to help users express their ideas visually. Things to try One interesting thing to try with PhotoMaker is to experiment with different text prompts and see how the model responds. You could try prompts that combine specific details about the desired image with more abstract or creative language, or prompts that ask the model to mix different artistic styles. Additionally, you could try using the model in conjunction with other LoRA modules or fine-tuning it on different datasets to see how it performs in different contexts.
Updated 4/29/2024
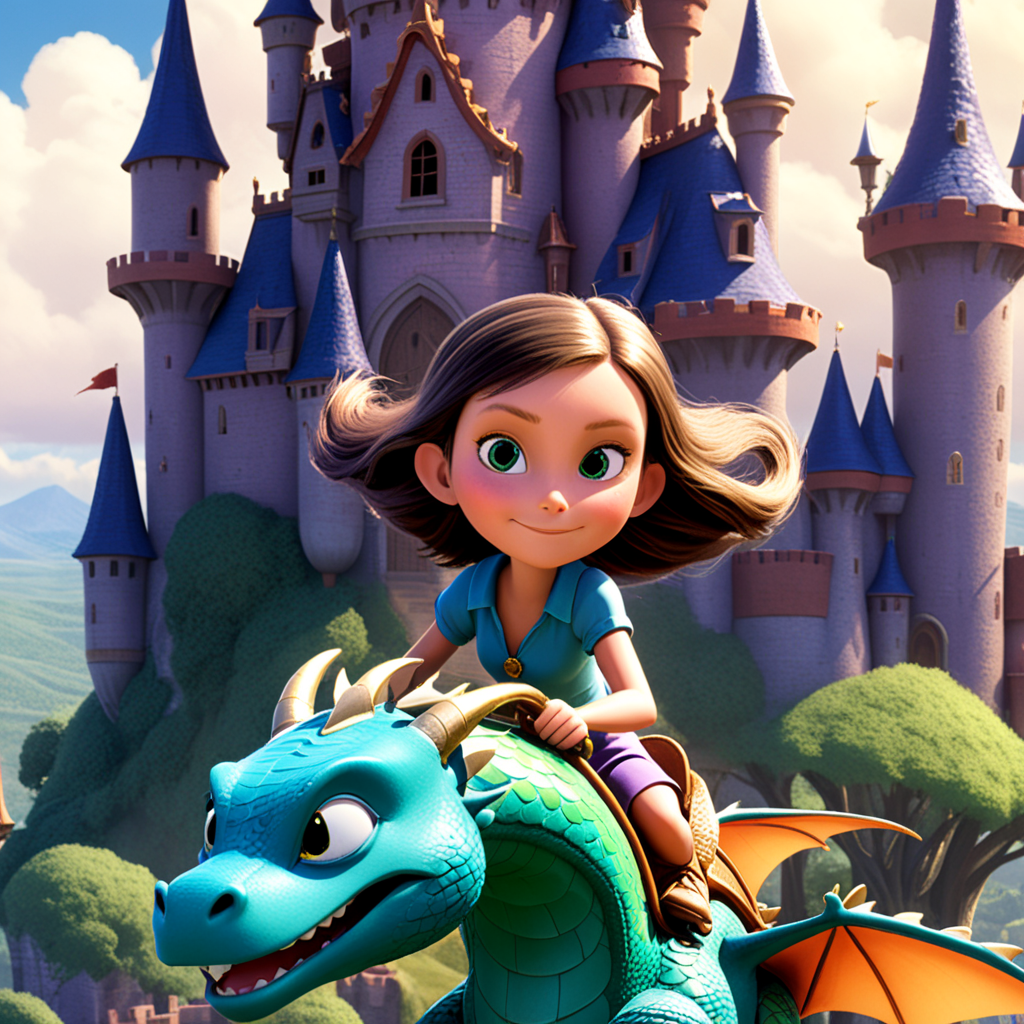
photomaker-style

276.864
photomaker-style is an AI model created by Tencent ARC Lab that can customize realistic human photos in various artistic styles. It builds upon the base Stable Diffusion XL model and adds a stacked ID embedding module for high-fidelity face personalization. Compared to similar models like GFPGAN for face restoration or the original PhotoMaker for realistic photo generation, photomaker-style specializes in applying artistic styles to personalized human faces. It can quickly generate photos, paintings, and avatars in diverse styles within seconds. Model inputs and outputs photomaker-style takes in one or more face photos of the person to be customized, along with a text prompt describing the desired style and appearance. The model then outputs a set of customized images in the requested style, preserving the identity of the input face. Inputs Input Image(s)**: One or more face photos of the person to be customized Prompt**: Text prompt describing the desired style and appearance, e.g. "a photo of a woman img in the style of Vincent Van Gogh" Negative Prompt**: Text prompt describing undesired elements to avoid in the output Seed**: Optional integer seed value for reproducible generation Guidance Scale**: Strength of the text-to-image guidance Style Strength Ratio**: Strength of the artistic style application Outputs Customized Images**: Set of images generated in the requested style, preserving the identity of the input face Capabilities photomaker-style can rapidly generate personalized images in diverse artistic styles, from photorealistic portraits to impressionistic paintings and stylized avatars. By leveraging the Stable Diffusion XL backbone and its stacked ID embedding module, the model ensures impressive identity fidelity while offering versatile text controllability and high-quality generation. What can I use it for? photomaker-style can be a powerful tool for quickly creating custom profile pictures, avatars, or artistic renditions of oneself or others. It could be used by individual users, content creators, or even businesses to generate personalized images for a variety of applications, such as social media, virtual events, or even product packaging and marketing. The ability to seamlessly blend identity and artistic style opens up new possibilities for self-expression, creative projects, and unique visual content. Things to try Experiment with different input face photos and prompts to see how photomaker-style can transform them into diverse artistic interpretations. Try out various styles like impressionism, expressionism, or surrealism. You can also combine photomaker-style with other LoRA modules or base models to explore even more creative possibilities. Additionally, consider using photomaker-style as an adapter to collaborate with other models in your projects, leveraging its powerful face personalization capabilities.
Updated 4/29/2024
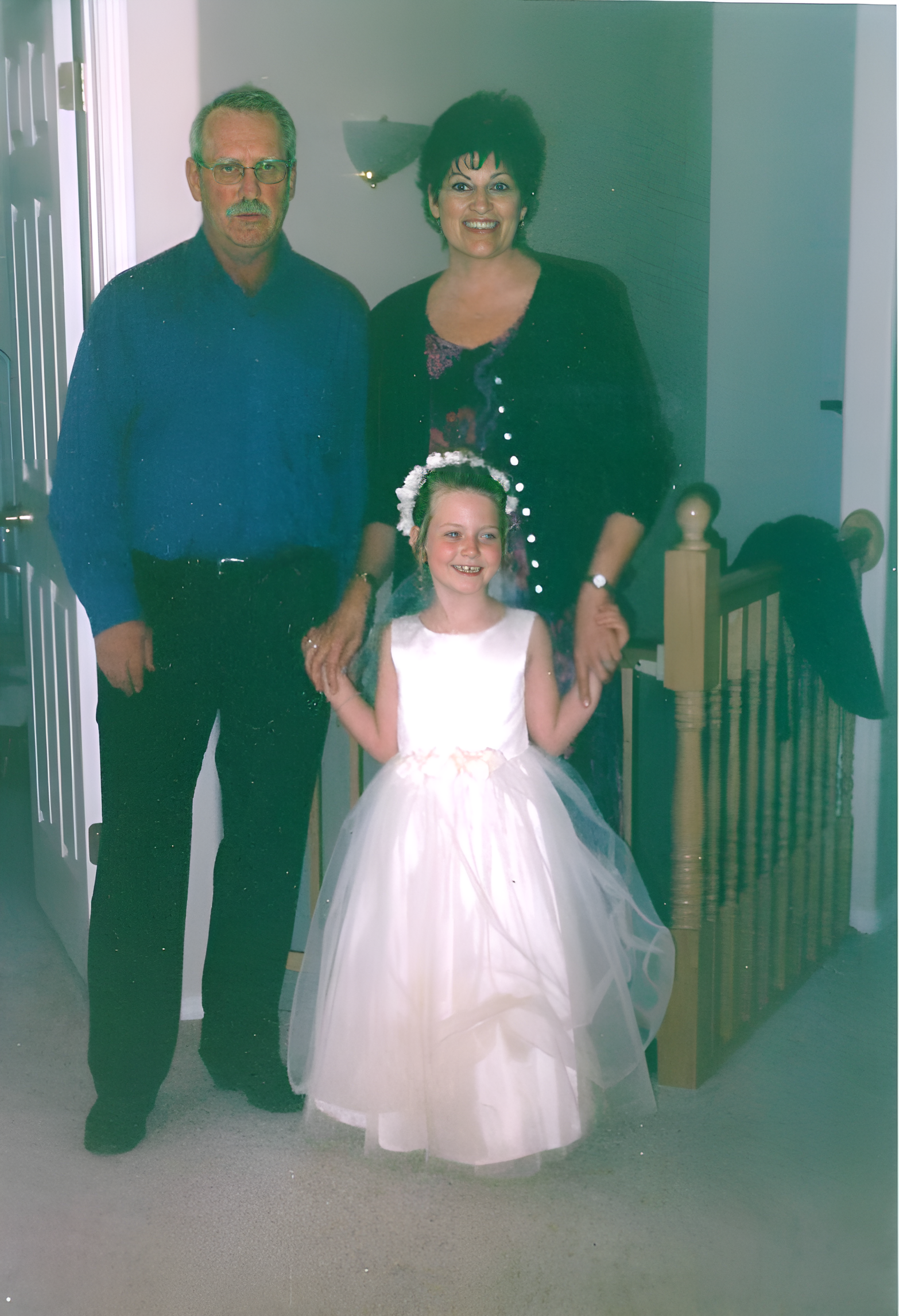
vqfr

152.938
vqfr is a blind face restoration model developed by Tencent ARC that uses a vector-quantized dictionary and parallel decoder to produce realistic facial details while maintaining comparable fidelity. It builds upon prior face restoration models like GFPGAN and CodeFormer by incorporating a novel vector-quantized dictionary mechanism. Compared to these models, vqfr is able to generate more detailed and natural-looking facial textures. Model inputs and outputs vqfr takes in an input image, which can be either a full image containing a face or a cropped/aligned face. The model then outputs the restored face with improved details and the whole image with the face region enhanced. Inputs Image**: Input image, which can be a full image with a face or a cropped/aligned face. Aligned**: Boolean flag indicating whether the input is an aligned face. Outputs Restored Faces**: The model outputs the restored face regions with improved details. Whole Image**: The model also outputs the whole image with the face region enhanced. Capabilities vqfr is capable of blindly restoring faces in low-quality images, whether they are old photos, AI-generated faces, or images with other degradation factors. It can produce realistic facial details while maintaining comparable fidelity to the input. The model's vector-quantized dictionary mechanism allows it to generate more natural-looking textures compared to previous face restoration models. What can I use it for? vqfr can be used for a variety of applications that involve restoring low-quality or degraded facial images, such as: Enhancing old family photos Improving the quality of AI-generated faces Restoring damaged or low-resolution facial images By using vqfr, you can breathe new life into your old photos or fix up AI-generated images to make them look more realistic and natural. Things to try One interesting aspect of vqfr is its ability to balance fidelity and quality through a user-controllable fidelity ratio. By adjusting this ratio, you can experiment with different trade-offs between the overall quality of the restored face and its similarity to the original input. This allows you to customize the model's output to your specific needs or preferences. Another thing to try is using vqfr in conjunction with background upsampling models like Real-ESRGAN to enhance the entire image, not just the face region. This can produce more visually compelling and consistent results for your restoration projects.
Updated 4/29/2024
📶
animesr

9.856
animesr is a real-world super-resolution model for animation videos developed by the Tencent ARC Lab. It can effectively enhance the resolution and quality of low-quality animation videos, producing clear and high-quality results. Compared to similar models like GFPGAN, Real-ESRGAN, RealESRGAN, and PhotoMaker Style, animesr is specifically designed for animation videos, providing superior performance on this type of content. Model inputs and outputs animesr takes either a video file or a zip file of image frames as input, and outputs a high-quality, upscaled video. The model is capable of 4x super-resolution, meaning it can quadruple the resolution of the input video while preserving details and reducing artifacts. Inputs Video**: Input video file Frames**: Zip file of frames from a video Outputs Video**: High-quality, upscaled video Capabilities animesr excels at enhancing the resolution and visual quality of animation videos, producing clear and detailed results with fewer artifacts compared to traditional upscaling methods. The model has been trained on a large dataset of animation videos, allowing it to effectively handle a wide range of animation styles and content. What can I use it for? The animesr model can be used to improve the quality of low-resolution animation videos, making them more suitable for larger displays or video streaming platforms. This can be particularly useful for restoring old or degraded animation content, or for upscaling lower-quality animation created for mobile devices. Additionally, the model could be leveraged by animation studios or video editors to efficiently enhance the production value of their animated projects. Things to try One interesting aspect of animesr is its ability to handle a diverse range of animation styles and content. Try experimenting with the model on different types of animation, from classic cartoons to modern anime, to see how it performs across various visual styles and genres. Additionally, you can explore the impact of adjusting the output scale, as the model supports arbitrary scaling factors beyond the default 4x resolution.
Updated 4/29/2024