Pwntus
Models by this creator
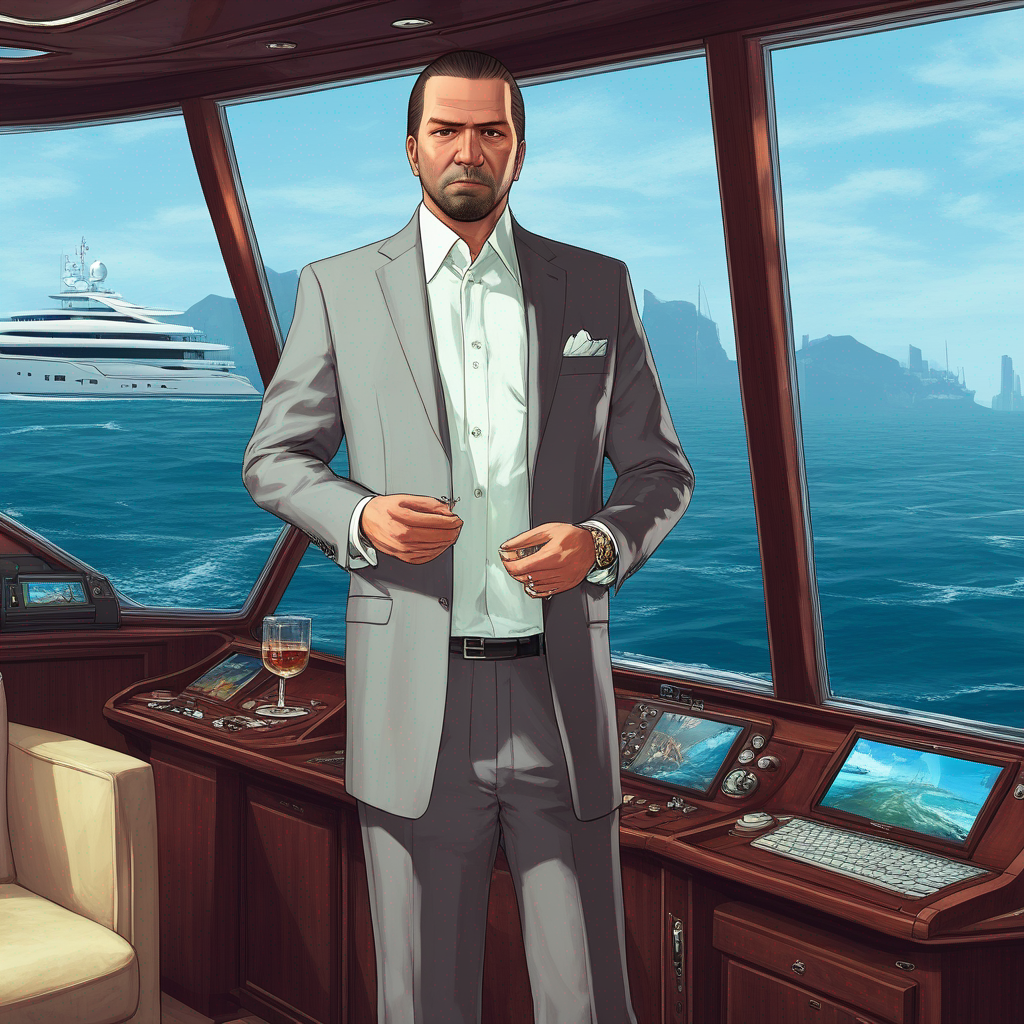
sdxl-gta-v

35
sdxl-gta-v is a fine-tuned version of the SDXL (Stable Diffusion XL) model, trained on art from the popular video game Grand Theft Auto V. This model was developed by pwntus, who has also created other interesting AI models like gfpgan, a face restoration algorithm for old photos or AI-generated faces. Model Inputs and Outputs The sdxl-gta-v model accepts a variety of inputs to generate unique images, including a prompt, an input image for img2img or inpaint mode, and various settings to control the output. The model can produce one or more images per run, with options to adjust aspects like the image size, guidance scale, and number of inference steps. Inputs Prompt**: The text prompt that describes the desired image Image**: An input image for img2img or inpaint mode Mask**: A mask for the inpaint mode, where black areas will be preserved and white areas will be inpainted Seed**: A random seed value, which can be left blank to randomize the output Width/Height**: The desired dimensions of the output image Num Outputs**: The number of images to generate (up to 4) Scheduler**: The denoising scheduler to use Guidance Scale**: The scale for classifier-free guidance Num Inference Steps**: The number of denoising steps to perform Prompt Strength**: The strength of the prompt when using img2img or inpaint mode Refine**: The refine style to use LoRA Scale**: The additive scale for LoRA (only applicable on trained models) High Noise Frac**: The fraction of noise to use for the expert_ensemble_refiner Apply Watermark**: Whether to apply a watermark to the generated images Outputs One or more output images generated based on the provided inputs Capabilities The sdxl-gta-v model is capable of generating high-quality, GTA V-themed images based on text prompts. It can also perform inpainting tasks, where it fills in missing or damaged areas of an input image. The model's fine-tuning on GTA V art allows it to capture the unique aesthetics and style of the game, making it a useful tool for creators and artists working in the GTA V universe. What Can I Use It For? The sdxl-gta-v model could be used for a variety of projects, such as creating promotional materials, fan art, or even generating assets for GTA V-inspired games or mods. Its inpainting capabilities could also be useful for restoring or enhancing existing GTA V artwork. Additionally, the model's versatility allows it to be used for more general image generation tasks, making it a potentially valuable tool for a wide range of creative applications. Things to Try Some interesting things to try with the sdxl-gta-v model include experimenting with different prompt styles to capture various aspects of the GTA V universe, such as specific locations, vehicles, or characters. You could also try using the inpainting feature to modify existing GTA V-themed images or to create seamless composites of different game elements. Additionally, exploring the model's capabilities with different settings, like adjusting the guidance scale or number of inference steps, could lead to unique and unexpected results.
Updated 5/16/2024
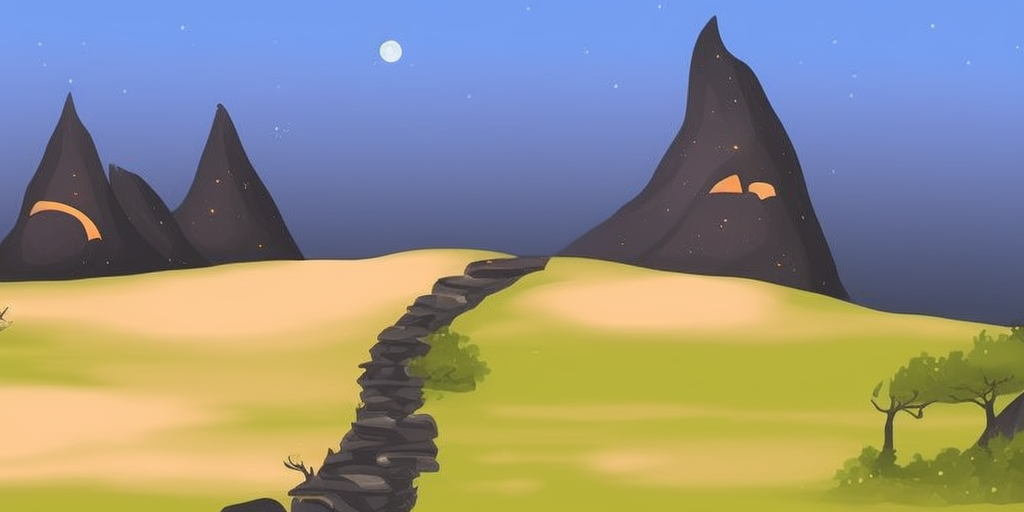
stable-diffusion-depth2img

6
stable-diffusion-depth2img is a Cog implementation of the Diffusers Stable Diffusion v2 model, which is capable of generating variations of an image while preserving its shape and depth. This model builds upon the Stable Diffusion model, which is a powerful latent text-to-image diffusion model that can generate photo-realistic images from any text input. The stable-diffusion-depth2img model adds the ability to create variations of an existing image, while maintaining the overall structure and depth information. Model inputs and outputs The stable-diffusion-depth2img model takes a variety of inputs to control the image generation process, including a prompt, an existing image, and various parameters to fine-tune the output. The model then generates one or more new images based on these inputs. Inputs Prompt**: The text prompt that guides the image generation process. Image**: The existing image that will be used as the starting point for the process. Seed**: An optional random seed value to control the image generation. Scheduler**: The type of scheduler to use for the diffusion process. Num Outputs**: The number of images to generate (up to 8). Guidance Scale**: The scale for classifier-free guidance, which controls the balance between the text prompt and the input image. Negative Prompt**: An optional prompt that specifies what the model should not generate. Prompt Strength**: The strength of the text prompt relative to the input image. Num Inference Steps**: The number of denoising steps to perform during the image generation process. Outputs Images**: One or more new images generated based on the provided inputs. Capabilities The stable-diffusion-depth2img model can be used to generate a wide variety of image variations based on an existing image. By preserving the shape and depth information from the input image, the model can create new images that maintain the overall structure and composition, while introducing new elements and variations based on the provided text prompt. This can be useful for tasks such as art generation, product design, and architectural visualization. What can I use it for? The stable-diffusion-depth2img model can be used for a variety of creative and design-related projects. For example, you could use it to generate concept art for a fantasy landscape, create variations of a product design, or explore different architectural styles for a building. The ability to preserve the shape and depth information of the input image can be particularly useful for these types of applications, as it allows you to maintain the overall structure and composition while introducing new elements and variations. Things to try One interesting thing to try with the stable-diffusion-depth2img model is to experiment with different prompts and input images to see how the model generates new variations. Try using a variety of input images, from landscapes to still lifes to abstract art, and see how the model responds to different types of visual information. You can also play with the various parameters, such as guidance scale and prompt strength, to fine-tune the output and explore the limits of the model's capabilities.
Updated 5/16/2024
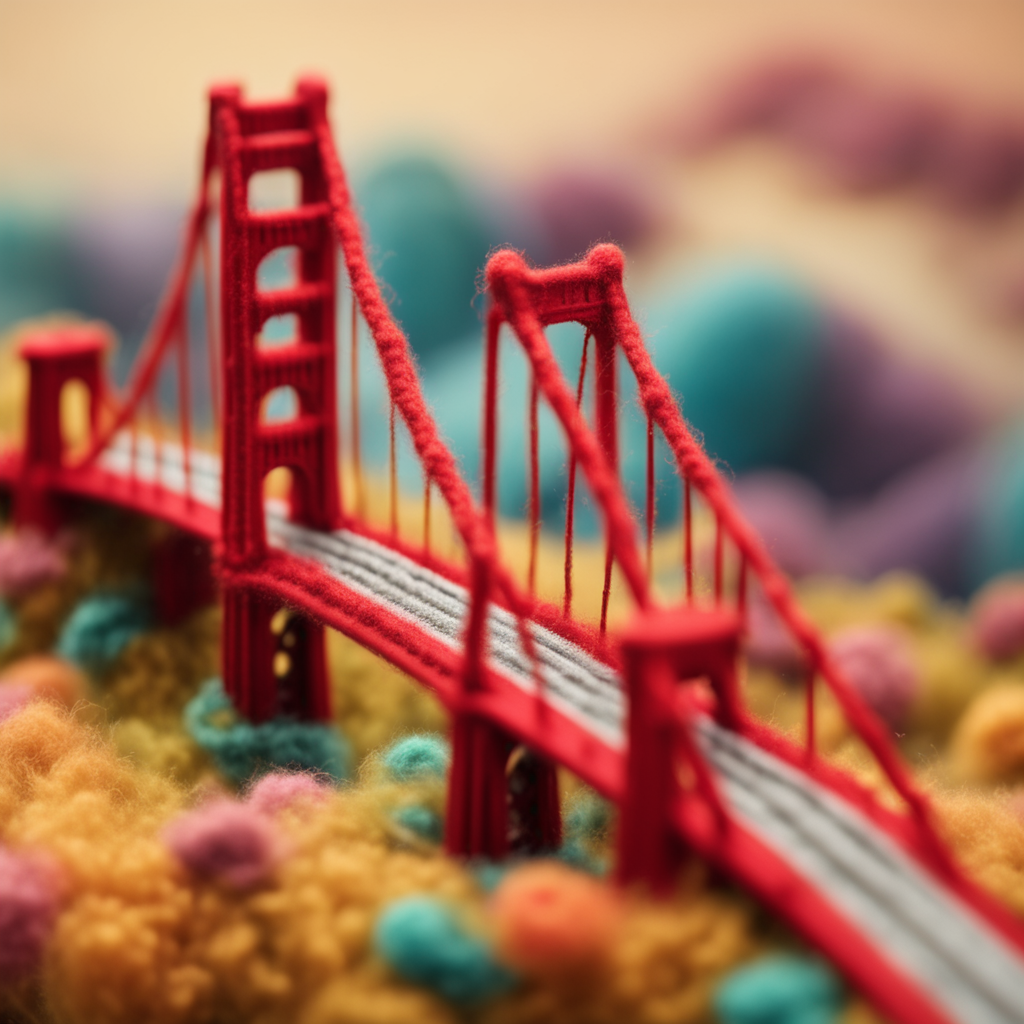
sdxl-woolitize

1
The sdxl-woolitize model is a fine-tuned version of the SDXL (Stable Diffusion XL) model, created by the maintainer pwntus. It is based on felted wool, a unique material that gives the generated images a distinctive textured appearance. Similar models like woolitize and sdxl-color have also been created to explore different artistic styles and materials. Model inputs and outputs The sdxl-woolitize model takes a variety of inputs, including a prompt, image, mask, and various parameters to control the output. It generates one or more output images based on the provided inputs. Inputs Prompt**: The text prompt describing the desired image Image**: An input image for img2img or inpaint mode Mask**: An input mask for inpaint mode, where black areas will be preserved and white areas will be inpainted Width/Height**: The desired width and height of the output image Seed**: A random seed value to control the output Refine**: The refine style to use Scheduler**: The scheduler algorithm to use LoRA Scale**: The LoRA additive scale (only applicable on trained models) Num Outputs**: The number of images to generate Refine Steps**: The number of steps to refine the image (for base_image_refiner) Guidance Scale**: The scale for classifier-free guidance Apply Watermark**: Whether to apply a watermark to the generated image High Noise Frac**: The fraction of noise to use (for expert_ensemble_refiner) Negative Prompt**: An optional negative prompt to guide the image generation Outputs Image(s)**: One or more generated images in the specified size Capabilities The sdxl-woolitize model is capable of generating images with a unique felted wool-like texture. This style can be used to create a wide range of artistic and whimsical images, from fantastical creatures to abstract compositions. What can I use it for? The sdxl-woolitize model could be used for a variety of creative projects, such as generating concept art, illustrations, or even textiles and fashion designs. The distinct felted wool aesthetic could be particularly appealing for children's books, fantasy-themed projects, or any application where a handcrafted, organic look is desired. Things to try Experiment with different prompt styles and modifiers to see how the model responds. Try combining the sdxl-woolitize model with other fine-tuned models, such as sdxl-gta-v or sdxl-deep-down, to create unique hybrid styles. Additionally, explore the limits of the model by providing challenging or abstract prompts and see how it handles them.
Updated 5/16/2024
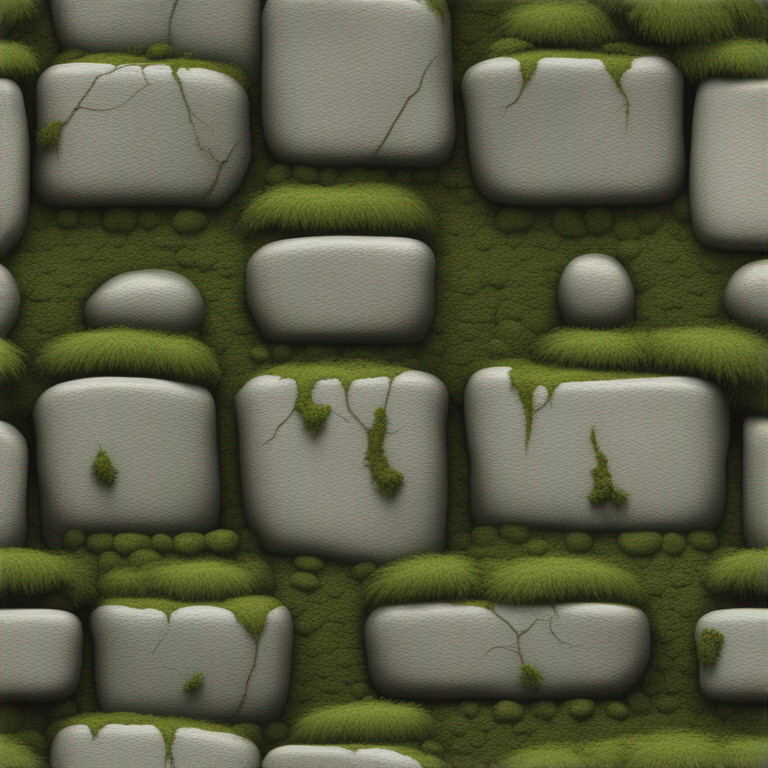
material-diffusion-sdxl

1
material-diffusion-sdxl is a Stable Diffusion XL model developed by pwntus that outputs tileable images for use in 3D applications such as Monaverse. It builds upon the Diffusers Stable Diffusion XL model by optimizing the output for seamless tiling. This can be useful for creating textures, patterns, and seamless backgrounds for 3D environments and virtual worlds. Model inputs and outputs The material-diffusion-sdxl model takes a variety of inputs to control the generation process, including a text prompt, image size, number of outputs, and more. The outputs are URLs pointing to the generated image(s). Inputs Prompt**: The text prompt that describes the desired image Negative Prompt**: Text to guide the model away from certain outputs Width/Height**: The dimensions of the generated image Num Outputs**: The number of images to generate Num Inference Steps**: The number of denoising steps to use during generation Guidance Scale**: The scale for classifier-free guidance Seed**: A random seed to control the generation process Refine**: The type of refiner to use on the output Refine Steps**: The number of refine steps to use High Noise Frac**: The fraction of noise to use for the expert ensemble refiner Apply Watermark**: Whether to apply a watermark to the generated images Outputs Image URLs**: A list of URLs pointing to the generated images Capabilities The material-diffusion-sdxl model is capable of generating high-quality, tileable images across a variety of subjects and styles. It can be used to create seamless textures, patterns, and backgrounds for 3D environments and virtual worlds. The model's ability to output images in a tileable format sets it apart from more general text-to-image models like Stable Diffusion. What can I use it for? The material-diffusion-sdxl model can be used to generate tileable textures, patterns, and backgrounds for 3D applications, virtual environments, and other visual media. This can be particularly useful for game developers, 3D artists, and designers who need to create seamless and repeatable visual elements. The model can also be fine-tuned on specific materials or styles to create custom assets, as demonstrated by the sdxl-woolitize model. Things to try Experiment with different prompts and input parameters to see the variety of tileable images the material-diffusion-sdxl model can generate. Try prompts that describe specific materials, patterns, or textures to see how the model responds. You can also try using the model in combination with other tools and techniques, such as 3D modeling software or image editing programs, to create unique and visually striking assets for your projects.
Updated 5/16/2024