Lambdal
Models by this creator
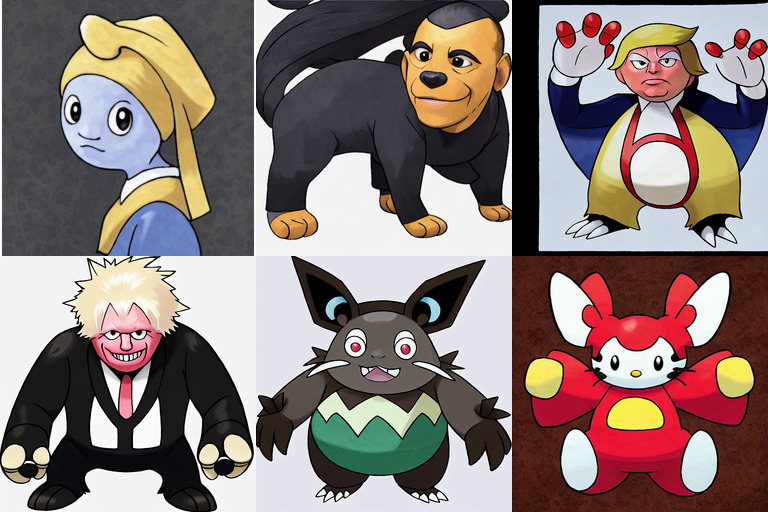
text-to-pokemon

7.8K
The text-to-pokemon model, created by Lambda Labs, is a Stable Diffusion-based AI model that can generate Pokémon characters from text prompts. This model builds upon the capabilities of the Stable Diffusion model, which is a latent text-to-image diffusion model capable of generating photo-realistic images from any text input. The text-to-pokemon model has been fine-tuned on a dataset of BLIP captioned Pokémon images, allowing it to generate unique Pokémon creatures based on text prompts. This is similar to other Stable Diffusion variants, such as the sd-pokemon-diffusers and pokemon-stable-diffusion models, which also focus on generating Pokémon-themed images. Model inputs and outputs Inputs Prompt**: A text description of the Pokémon character you would like to generate. Seed**: An optional integer value to set the random seed, allowing you to reproduce the same generated image. Guidance Scale**: A value that controls the influence of the text prompt on the generated image, with higher values leading to outputs that more closely match the prompt. Num Inference Steps**: The number of denoising steps to perform during the image generation process. Num Outputs**: The number of Pokémon images to generate based on the provided prompt. Outputs Images**: The generated Pokémon images, returned as a list of image URLs. Capabilities The text-to-pokemon model can generate a wide variety of unique Pokémon creatures based on text prompts, ranging from descriptions of existing Pokémon species to completely novel creatures. The model is capable of capturing the distinct visual characteristics and features of Pokémon, such as their body shapes, coloration, and distinctive features like wings, tails, or other appendages. What can I use it for? The text-to-pokemon model can be used to create custom Pokémon art and content for a variety of applications, such as: Generating unique Pokémon characters for use in fan art, stories, or games Exploring creative and imaginative Pokémon designs and concepts Developing Pokémon-themed assets for use in web content, mobile apps, or other digital media Things to try Some interesting prompts to try with the text-to-pokemon model include: Describing a Pokémon with a unique type or elemental affinity, such as a "fire and ice type dragon Pokémon" Combining different Pokémon features or characteristics, like a "Pokémon that is part cat, part bird, and part robot" Generating Pokémon based on real-world animals or mythological creatures, such as a "majestic unicorn Pokémon" or a "Pokémon based on a giant panda" Experimenting with the guidance scale and number of inference steps can also produce a range of different results, from more realistic to more abstract or stylized Pokémon designs.
Updated 5/17/2024
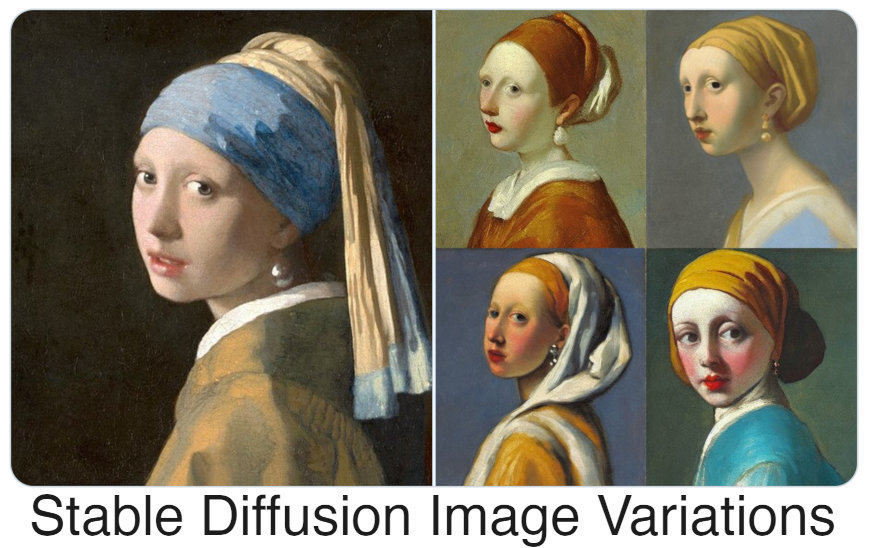
stable-diffusion-image-variation

236
stable-diffusion-image-variation is a fine-tuned version of the Stable Diffusion model created by Lambda Labs. This model is conditioned on CLIP image embeddings, enabling it to generate image variations based on an input image. This is in contrast to the original Stable Diffusion model, which generates images from text prompts. The stable-diffusion-image-variation model can be used to create stylized or altered versions of an existing image. Model inputs and outputs The stable-diffusion-image-variation model takes an input image and parameters such as guidance scale and number of inference steps to control the generation process. It outputs a set of new images that are variations on the input. Inputs Input Image**: The image to generate variations from Guidance Scale**: A scaling factor that controls the strength of the CLIP image guidance Num Inference Steps**: The number of denoising steps to perform during generation Outputs Output Images**: A set of generated image variations based on the input Capabilities The stable-diffusion-image-variation model can be used to create unique and creative image variations from a starting point. This can be useful for tasks like image editing, artistic exploration, and content generation. The model is able to generate a diverse range of outputs while maintaining the overall structure and content of the input image. What can I use it for? The stable-diffusion-image-variation model can be used for a variety of creative and practical applications. For example, you could use it to generate concept art, design assets, or experiment with different artistic styles. The model's ability to produce unique variations on an input image makes it well-suited for tasks like product visualization, fashion design, and visual effects. Things to try One interesting thing to try with the stable-diffusion-image-variation model is to provide it with a range of diverse input images and see how it generates variations. This can lead to unexpected and serendipitous results, as the model may combine elements from the input images in novel ways. You could also experiment with adjusting the guidance scale and number of inference steps to see how they affect the output.
Updated 5/17/2024
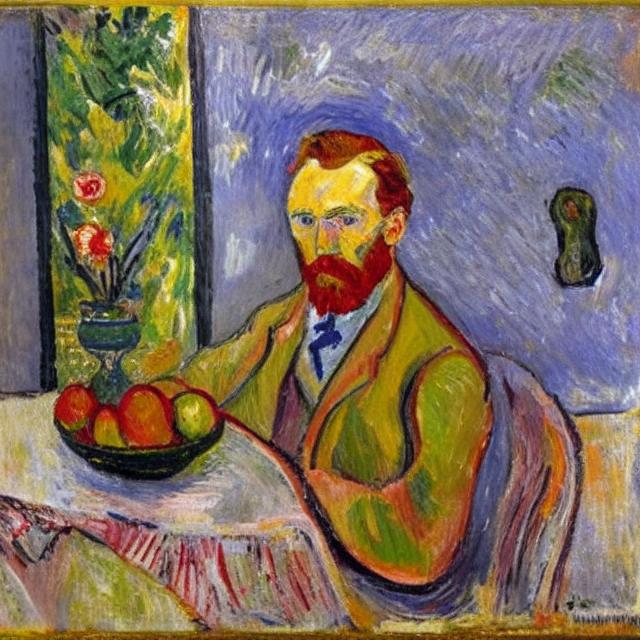
image-mixer

8
The image-mixer model, created by lambdal, allows users to blend and mix two input images using Stable Diffusion. This model is similar to other Stable Diffusion-based models like stable-diffusion-inpainting, masactrl-stable-diffusion-v1-4, realisticoutpainter, ssd-1b-img2img, and stable-diffusion-x4-upscaler, which offer various image editing and generation capabilities. Model inputs and outputs The image-mixer model takes two input images, along with various parameters to control the mixing and generation process. The output is an array of generated images that blend the two input images. Inputs image1**: The first input image image2**: The second input image image1_strength**: The mixing strength of the first image image2_strength**: The mixing strength of the second image num_steps**: The number of iterations for the generation process cfg_scale**: The Classifier-Free Guidance Scale, which controls the balance between image fidelity and creativity num_samples**: The number of output images to generate Outputs An array of generated images that blend the two input images Capabilities The image-mixer model can be used to create unique and visually striking images by blending two input images. This can be useful for a variety of applications, such as: Generating artistic and surreal-looking images Experimenting with different image combinations and styles Creating unique background images or textures for digital art or design projects What can I use it for? The image-mixer model can be used in a variety of creative projects, such as: Generating unique artwork or digital illustrations Experimenting with different image blending techniques Creating custom backgrounds or textures for graphic design or web development Exploring the possibilities of AI-generated imagery Things to try One interesting thing to try with the image-mixer model is to experiment with different input image combinations and parameter settings. Try using a range of different image types, from photographs to digital artwork, and see how the model blends them together. You can also play with the mixing strength and number of steps to create more abstract or realistic-looking outputs.
Updated 5/17/2024
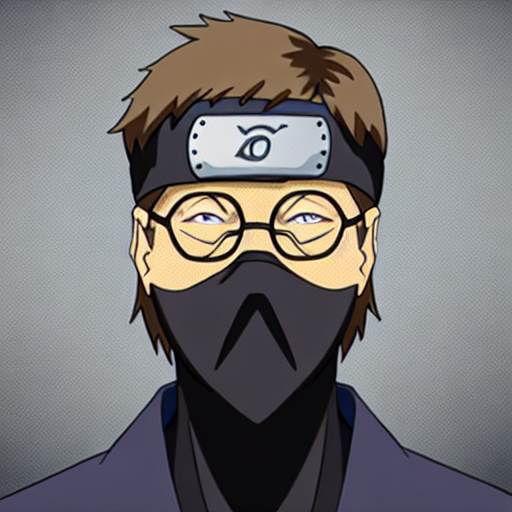
sd-naruto-diffusers

2
sd-naruto-diffusers is a fine-tuned version of the Stable Diffusion model, trained specifically on Naruto-themed images. Like the original Stable Diffusion model, it is a latent text-to-image diffusion model capable of generating photo-realistic images from text prompts. The model was created by lambdal, who has also developed other Stable Diffusion variants such as stable-diffusion-image-variation. Model inputs and outputs sd-naruto-diffusers takes in a text prompt and various parameters to control the output image generation, and returns one or more generated images. The key inputs include the prompt, image size, number of outputs, guidance scale, and number of inference steps. Inputs Prompt**: The text prompt that describes the desired image Seed**: A random seed value to control the randomness of the output Width/Height**: The desired size of the output image Num Outputs**: The number of images to generate Guidance Scale**: A scale value to control the influence of the text prompt Num Inference Steps**: The number of denoising steps to perform during image generation Outputs Image(s)**: One or more generated image(s) as image URLs Capabilities sd-naruto-diffusers is capable of generating a wide variety of Naruto-themed images based on text prompts. This includes scenes, characters, and items from the Naruto universe. The model can capture the distinct visual style and aesthetics of the Naruto franchise. What can I use it for? You can use sd-naruto-diffusers to create custom Naruto-themed artwork, illustrations, and visualizations. This could be useful for Naruto fans, content creators, or anyone looking to incorporate Naruto elements into their projects. The model's flexibility allows for a broad range of potential applications, from fan art to commercial projects. Things to try Experiment with different text prompts to see the range of Naruto-inspired images the model can generate. Try prompts that combine Naruto characters, settings, and objects in unique ways. You can also adjust the various input parameters to fine-tune the output and explore the model's capabilities further.
Updated 5/17/2024