resnet
Maintainer: replicate

7
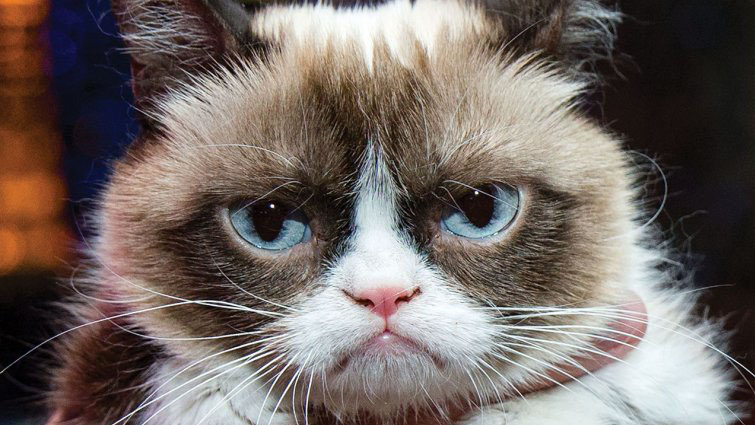
Property | Value |
---|---|
Model Link | View on Replicate |
API Spec | View on Replicate |
Github Link | View on Github |
Paper Link | No paper link provided |
Get summaries of the top AI models delivered straight to your inbox:
Model overview
The resnet
model is a popular image classification model developed by Microsoft. It is based on the ResNet (Residual Network) architecture, which uses skip connections to enable the training of deeper neural networks. The resnet
model is pre-trained on the ImageNet-1k dataset and can be used to classify images into 1,000 different categories.
Similar models include the ResNet-50 v1.5 model, which is a slightly more accurate version of the original ResNet-50 model, as well as the Stable Diffusion, GFPGAN, Real-ESRGAN, and BLIP models, which address different image-related tasks.
Model inputs and outputs
The resnet
model takes an image as input and outputs a classification of that image into one of the 1,000 ImageNet classes.
Inputs
- Image: The image to be classified, in the format of a URI.
Outputs
- Title: The output of the model, which is the predicted class label for the input image.
Capabilities
The resnet
model is capable of accurately classifying a wide variety of images into 1,000 different categories, making it a versatile tool for image recognition tasks. It has been widely adopted and used in a range of applications, from object detection to scene understanding.
What can I use it for?
The resnet
model can be used for a variety of image classification tasks, such as identifying objects, scenes, or activities in an image. It can be fine-tuned on specialized datasets to adapt it to specific use cases, such as medical image analysis or product recognition. Additionally, the model can be used as a feature extractor to provide input to other machine learning models, such as those used for image captioning or visual question answering.
Things to try
Some ideas for experimenting with the resnet
model include:
- Trying the model on a diverse set of images to see its performance across different categories.
- Fine-tuning the model on a specialized dataset to adapt it to a specific task.
- Using the model as a feature extractor for other machine learning models.
- Exploring the model's internal representations to gain insights into how it makes its predictions.
This summary was produced with help from an AI and may contain inaccuracies - check out the links to read the original source documents!
Related Models
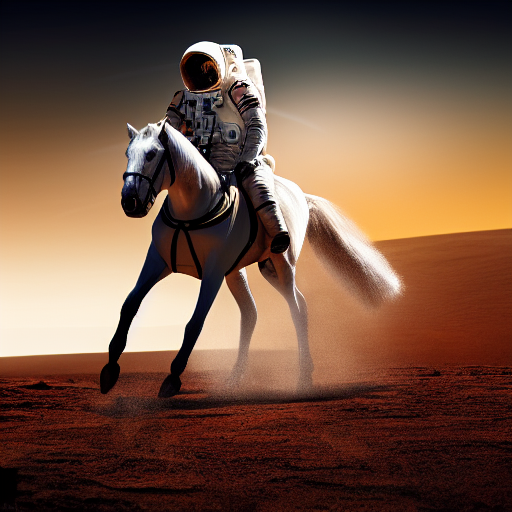
stable-diffusion

107.9K
Stable Diffusion is a latent text-to-image diffusion model capable of generating photo-realistic images given any text input. Developed by Stability AI, it is an impressive AI model that can create stunning visuals from simple text prompts. The model has several versions, with each newer version being trained for longer and producing higher-quality images than the previous ones. The main advantage of Stable Diffusion is its ability to generate highly detailed and realistic images from a wide range of textual descriptions. This makes it a powerful tool for creative applications, allowing users to visualize their ideas and concepts in a photorealistic way. The model has been trained on a large and diverse dataset, enabling it to handle a broad spectrum of subjects and styles. Model inputs and outputs Inputs Prompt**: The text prompt that describes the desired image. This can be a simple description or a more detailed, creative prompt. Seed**: An optional random seed value to control the randomness of the image generation process. Width and Height**: The desired dimensions of the generated image, which must be multiples of 64. Scheduler**: The algorithm used to generate the image, with options like DPMSolverMultistep. Num Outputs**: The number of images to generate (up to 4). Guidance Scale**: The scale for classifier-free guidance, which controls the trade-off between image quality and faithfulness to the input prompt. Negative Prompt**: Text that specifies things the model should avoid including in the generated image. Num Inference Steps**: The number of denoising steps to perform during the image generation process. Outputs Array of image URLs**: The generated images are returned as an array of URLs pointing to the created images. Capabilities Stable Diffusion is capable of generating a wide variety of photorealistic images from text prompts. It can create images of people, animals, landscapes, architecture, and more, with a high level of detail and accuracy. The model is particularly skilled at rendering complex scenes and capturing the essence of the input prompt. One of the key strengths of Stable Diffusion is its ability to handle diverse prompts, from simple descriptions to more creative and imaginative ideas. The model can generate images of fantastical creatures, surreal landscapes, and even abstract concepts with impressive results. What can I use it for? Stable Diffusion can be used for a variety of creative applications, such as: Visualizing ideas and concepts for art, design, or storytelling Generating images for use in marketing, advertising, or social media Aiding in the development of games, movies, or other visual media Exploring and experimenting with new ideas and artistic styles The model's versatility and high-quality output make it a valuable tool for anyone looking to bring their ideas to life through visual art. By combining the power of AI with human creativity, Stable Diffusion opens up new possibilities for visual expression and innovation. Things to try One interesting aspect of Stable Diffusion is its ability to generate images with a high level of detail and realism. Users can experiment with prompts that combine specific elements, such as "a steam-powered robot exploring a lush, alien jungle," to see how the model handles complex and imaginative scenes. Additionally, the model's support for different image sizes and resolutions allows users to explore the limits of its capabilities. By generating images at various scales, users can see how the model handles the level of detail and complexity required for different use cases, such as high-resolution artwork or smaller social media graphics. Overall, Stable Diffusion is a powerful and versatile AI model that offers endless possibilities for creative expression and exploration. By experimenting with different prompts, settings, and output formats, users can unlock the full potential of this cutting-edge text-to-image technology.
Updated Invalid Date
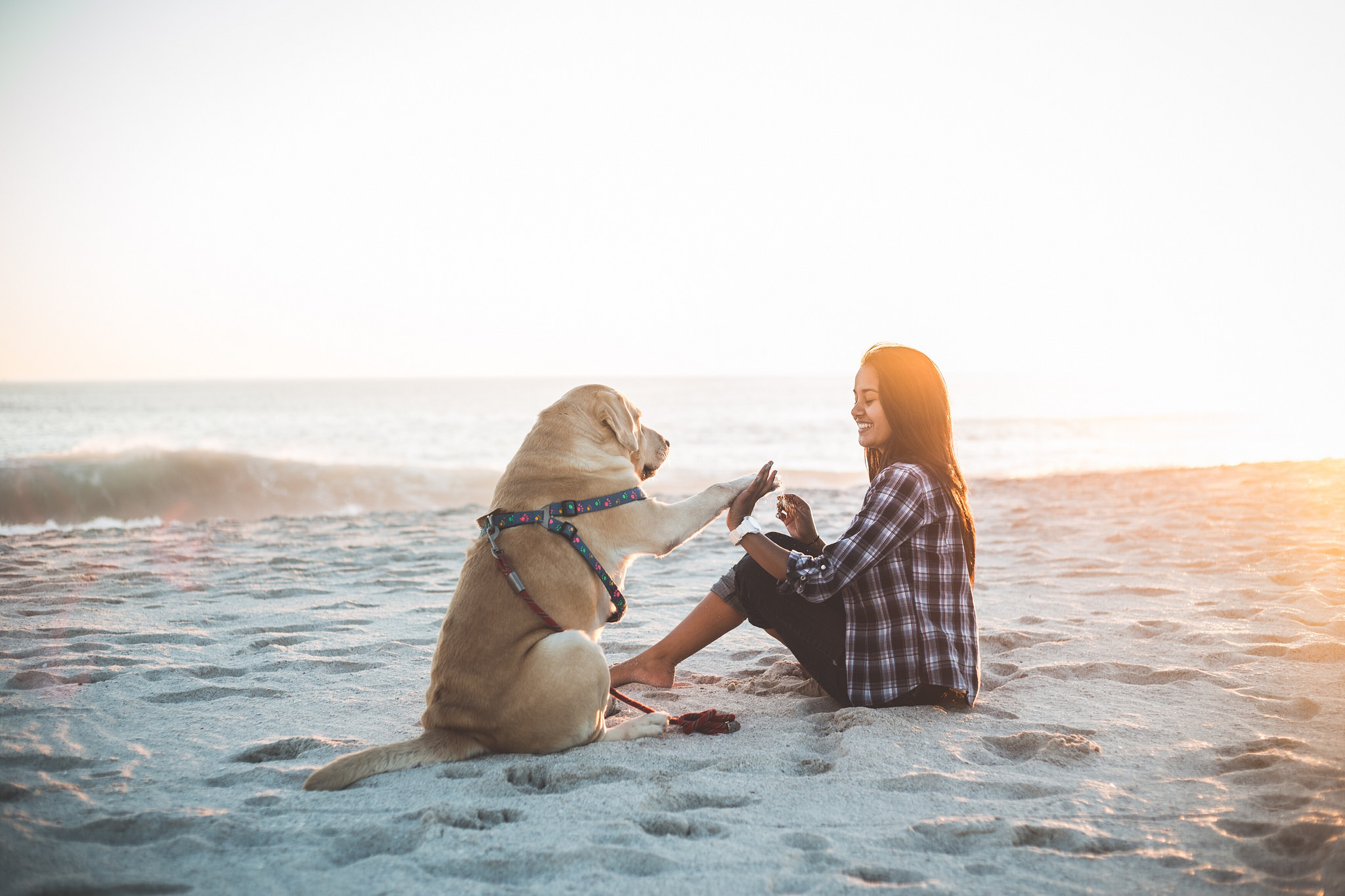
blip

82.1K
BLIP (Bootstrapping Language-Image Pre-training) is a vision-language model developed by Salesforce that can be used for a variety of tasks, including image captioning, visual question answering, and image-text retrieval. The model is pre-trained on a large dataset of image-text pairs and can be fine-tuned for specific tasks. Compared to similar models like blip-vqa-base, blip-image-captioning-large, and blip-image-captioning-base, BLIP is a more general-purpose model that can be used for a wider range of vision-language tasks. Model inputs and outputs BLIP takes in an image and either a caption or a question as input, and generates an output response. The model can be used for both conditional and unconditional image captioning, as well as open-ended visual question answering. Inputs Image**: An image to be processed Caption**: A caption for the image (for image-text matching tasks) Question**: A question about the image (for visual question answering tasks) Outputs Caption**: A generated caption for the input image Answer**: An answer to the input question about the image Capabilities BLIP is capable of generating high-quality captions for images and answering questions about the visual content of images. The model has been shown to achieve state-of-the-art results on a range of vision-language tasks, including image-text retrieval, image captioning, and visual question answering. What can I use it for? You can use BLIP for a variety of applications that involve processing and understanding visual and textual information, such as: Image captioning**: Generate descriptive captions for images, which can be useful for accessibility, image search, and content moderation. Visual question answering**: Answer questions about the content of images, which can be useful for building interactive interfaces and automating customer support. Image-text retrieval**: Find relevant images based on textual queries, or find relevant text based on visual input, which can be useful for building image search engines and content recommendation systems. Things to try One interesting aspect of BLIP is its ability to perform zero-shot video-text retrieval, where the model can directly transfer its understanding of vision-language relationships to the video domain without any additional training. This suggests that the model has learned rich and generalizable representations of visual and textual information that can be applied to a variety of tasks and modalities. Another interesting capability of BLIP is its use of a "bootstrap" approach to pre-training, where the model first generates synthetic captions for web-scraped image-text pairs and then filters out the noisy captions. This allows the model to effectively utilize large-scale web data, which is a common source of supervision for vision-language models, while mitigating the impact of noisy or irrelevant image-text pairs.
Updated Invalid Date
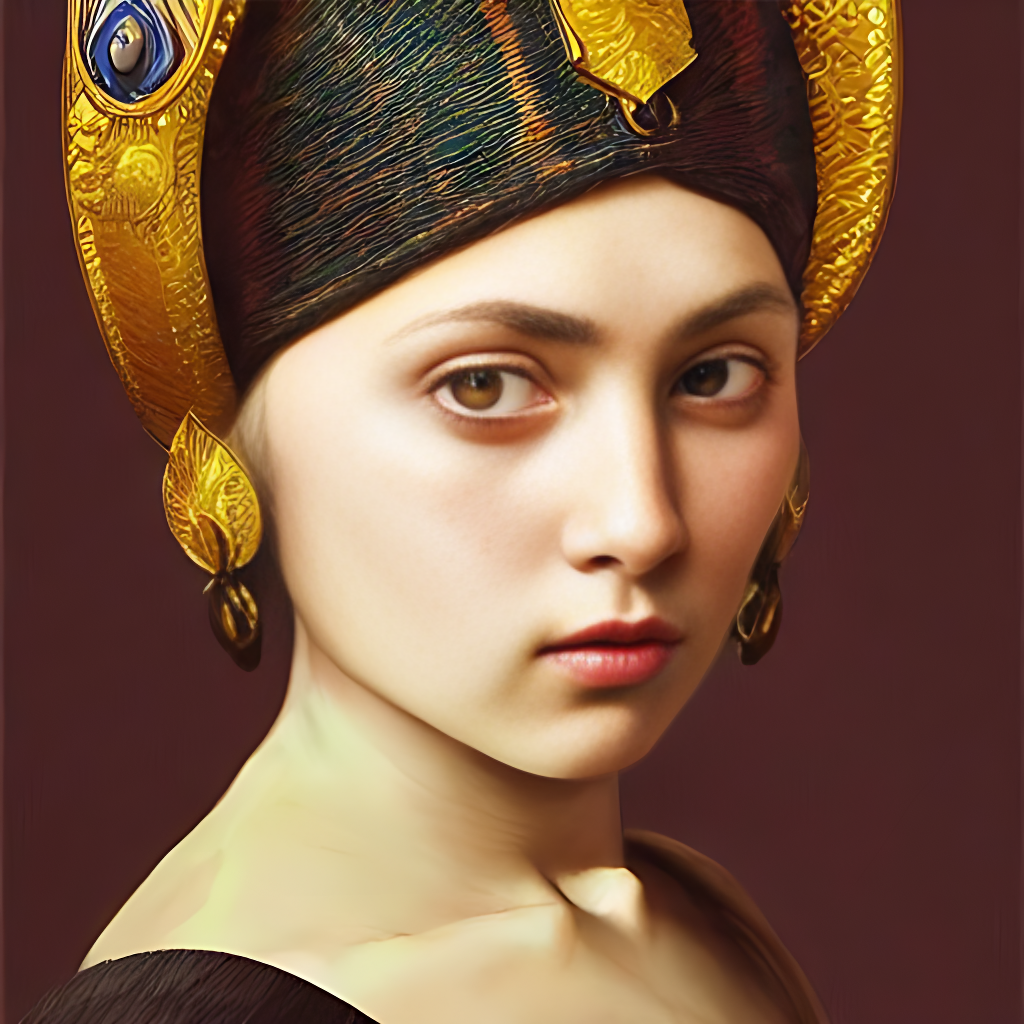
gfpgan

74.2K
gfpgan is a practical face restoration algorithm developed by the Tencent ARC team. It leverages the rich and diverse priors encapsulated in a pre-trained face GAN (such as StyleGAN2) to perform blind face restoration on old photos or AI-generated faces. This approach contrasts with similar models like Real-ESRGAN, which focuses on general image restoration, or PyTorch-AnimeGAN, which specializes in anime-style photo animation. Model inputs and outputs gfpgan takes an input image and rescales it by a specified factor, typically 2x. The model can handle a variety of face images, from low-quality old photos to high-quality AI-generated faces. Inputs Img**: The input image to be restored Scale**: The factor by which to rescale the output image (default is 2) Version**: The gfpgan model version to use (v1.3 for better quality, v1.4 for more details and better identity) Outputs Output**: The restored face image Capabilities gfpgan can effectively restore a wide range of face images, from old, low-quality photos to high-quality AI-generated faces. It is able to recover fine details, fix blemishes, and enhance the overall appearance of the face while preserving the original identity. What can I use it for? You can use gfpgan to restore old family photos, enhance AI-generated portraits, or breathe new life into low-quality images of faces. The model's capabilities make it a valuable tool for photographers, digital artists, and anyone looking to improve the quality of their facial images. Additionally, the maintainer tencentarc offers an online demo on Replicate, allowing you to try the model without setting up the local environment. Things to try Experiment with different input images, varying the scale and version parameters, to see how gfpgan can transform low-quality or damaged face images into high-quality, detailed portraits. You can also try combining gfpgan with other models like Real-ESRGAN to enhance the background and non-facial regions of the image.
Updated Invalid Date
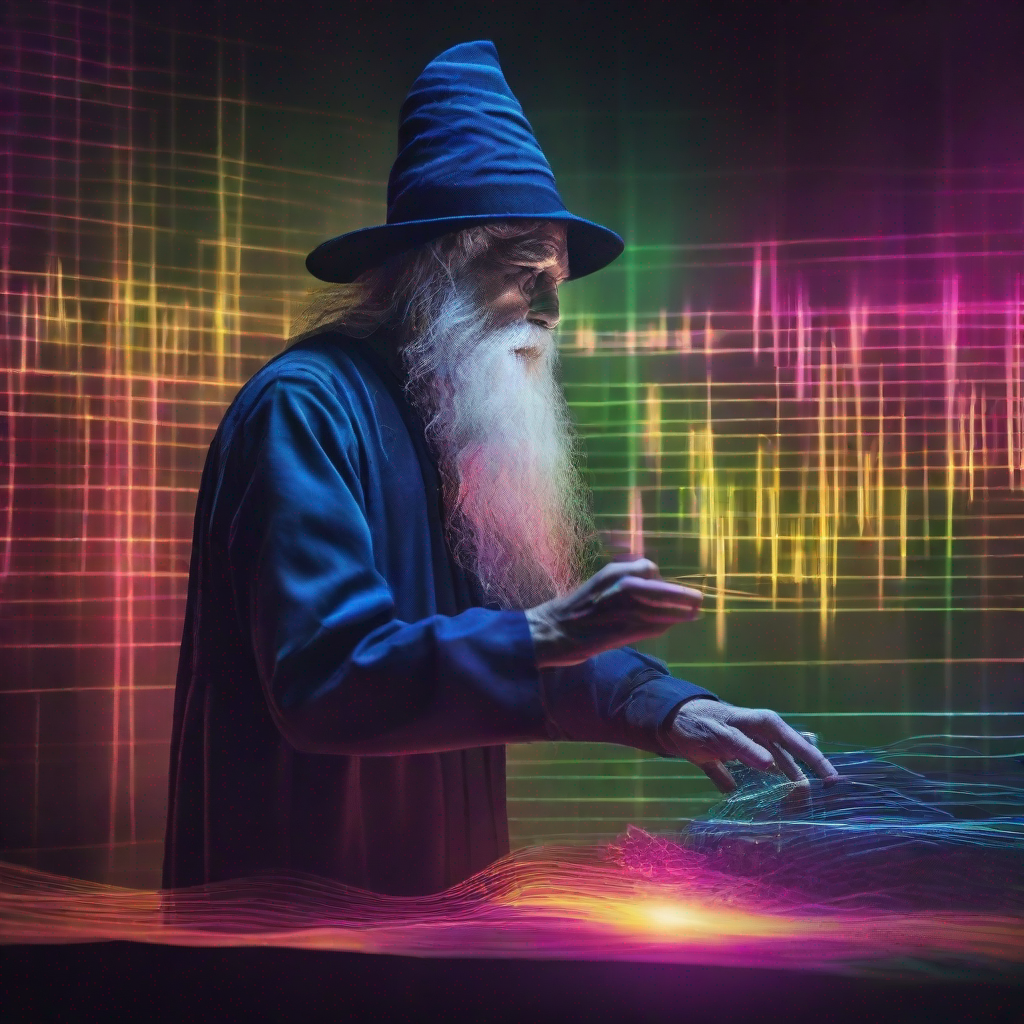
train-rvc-model

18
The train-rvc-model is a retrieval-based voice conversion framework developed by Replicate that allows users to train their own custom RVC (Retrieval-based Voice Conversion) models. It is built upon the VITS (Variational Inference for Text-to-Speech) architecture and aims to provide a simple and easy-to-use voice conversion solution. The model leverages techniques such as top-1 retrieval to prevent audio quality degradation and supports training with relatively small datasets, making it accessible for users with limited resources. The RVC framework can also be used to blend models for changing the output voice characteristics. Model inputs and outputs The train-rvc-model takes in various inputs to configure the training process, including the training dataset, the model version, the F0 (fundamental frequency) extraction method, the training epoch, and the batch size. The key inputs are: Inputs Dataset Zip**: A zip file containing the training dataset, with the dataset split into individual WAV files. Version**: The version of the RVC model to train, with the latest version being v2. F0 method**: The method used for extracting the fundamental frequency of the audio, with the recommended option being rmvpe_gpu. Epoch**: The number of training epochs to run. Batch Size**: The batch size to use during training. Outputs Output**: The trained RVC model, which can be used for voice conversion tasks. Capabilities The train-rvc-model is capable of training custom RVC models that can perform high-quality voice conversion, even with relatively small datasets. The model leverages advanced techniques like top-1 retrieval to prevent audio quality degradation and supports training on limited hardware resources. Additionally, the RVC framework allows for model blending, enabling users to adjust the output voice characteristics. What can I use it for? The train-rvc-model can be used for a variety of voice conversion applications, such as generating synthetic voices, dubbing audio in different languages, or creating personalized voice assistants. By training custom RVC models, users can tailor the voice characteristics to their specific needs, whether it's for personal projects, commercial applications, or creative endeavors. The model's ability to work with small datasets and its simple web-based interface make it accessible for a wide range of users. Things to try One interesting feature to explore with the train-rvc-model is the ability to blend multiple RVC models together. By utilizing the "ckpt-merge" option in the web interface, users can combine different trained models to create unique voice characteristics. This can be used to experiment with various voice styles or to refine the output based on specific preferences. Another aspect worth exploring is the model's performance on different hardware setups, including AMD Radeon and Intel IPEX-enabled GPUs. The RVC framework is designed to be hardware-agnostic, allowing users to leverage a variety of hardware configurations to train their models.
Updated Invalid Date