Tstramer
Models by this creator
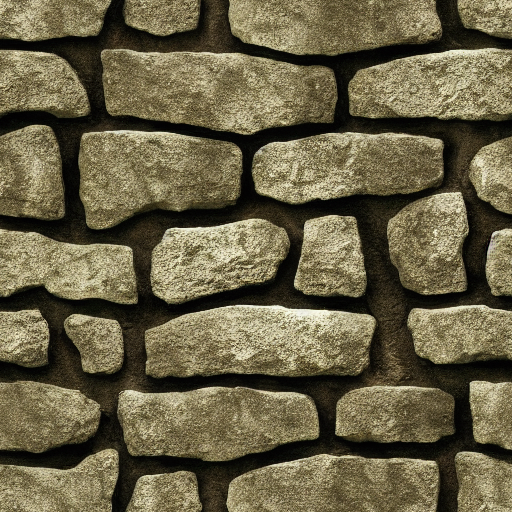
material-diffusion

2.1K
material-diffusion is a fork of the popular Stable Diffusion AI model, created by Replicate user tstramer. This model is designed for generating tileable outputs, building on the capabilities of the v1.5 Stable Diffusion model. It shares similarities with other Stable Diffusion forks like material-diffusion-sdxl and stable-diffusion-v2, as well as more experimental models like multidiffusion and stable-diffusion. Model inputs and outputs material-diffusion takes a variety of inputs, including a text prompt, a mask image, an initial image, and various settings to control the output. The model then generates one or more images based on the provided inputs. Inputs Prompt**: The text prompt that describes the desired image. Mask**: A black and white image used to mask the initial image, with black pixels inpainted and white pixels preserved. Init Image**: An initial image to generate variations of, which will be resized to the specified dimensions. Seed**: A random seed value to control the output image. Scheduler**: The diffusion scheduler algorithm to use, such as K-LMS. Guidance Scale**: A scale factor for the classifier-free guidance, which controls the balance between the input prompt and the initial image. Prompt Strength**: The strength of the input prompt when using an initial image, with 1.0 corresponding to full destruction of the initial image information. Num Inference Steps**: The number of denoising steps to perform during the image generation process. Outputs Output Images**: One or more images generated by the model, based on the provided inputs. Capabilities material-diffusion is capable of generating high-quality, photorealistic images from text prompts, similar to the base Stable Diffusion model. However, the key differentiator is its ability to generate tileable outputs, which can be useful for creating seamless patterns, textures, or backgrounds. What can I use it for? material-diffusion can be useful for a variety of applications, such as: Generating unique and customizable patterns, textures, or backgrounds for design projects, websites, or products. Creating tiled artwork or wallpapers for personal or commercial use. Exploring creative text-to-image generation with a focus on tileable outputs. Things to try With material-diffusion, you can experiment with different prompts, masks, and initial images to create a wide range of tileable outputs. Try using the model to generate seamless patterns or textures, or to create variations on a theme by modifying the prompt or other input parameters.
Updated 5/15/2024
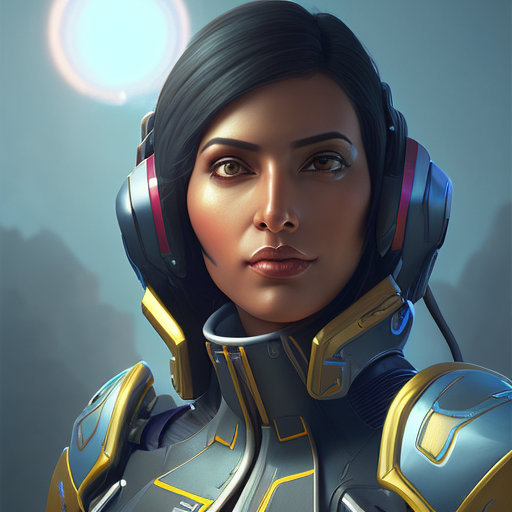
midjourney-diffusion

1.5K
The midjourney-diffusion model is a text-to-image AI model developed by tstramer at Replicate. It is similar to other diffusion-based models like openjourney, stable-diffusion, and multidiffusion, which leverage the power of diffusion processes to generate photorealistic images from textual descriptions. Model inputs and outputs The midjourney-diffusion model takes in a variety of inputs, including a textual prompt, image dimensions, and various parameters to control the output. These inputs are used to generate one or more images that match the provided prompt. The outputs are URLs pointing to the generated images. Inputs Prompt**: The textual description of the desired image Seed**: A random seed value to control the output Width/Height**: The desired dimensions of the output image Scheduler**: The algorithm used to generate the image Num Outputs**: The number of images to generate Guidance Scale**: A value to control the influence of the text prompt Negative Prompt**: A textual description of elements to exclude from the output Prompt Strength**: A value to control the strength of the prompt when using an init image Num Inference Steps**: The number of steps in the diffusion process Outputs Image URLs**: One or more URLs pointing to the generated images Capabilities The midjourney-diffusion model is capable of generating highly detailed and imaginative images from textual descriptions. It can create scenes, characters, and objects that blend realistic elements with fantastical and surreal components. The model's outputs often have a distinct visual style reminiscent of the Midjourney AI assistant. What can I use it for? The midjourney-diffusion model can be a powerful tool for creative projects, concept art, and visual storytelling. Its ability to transform text into visuals can be leveraged for things like book covers, game assets, product designs, and more. Businesses and individuals can explore the model's capabilities and experiment with different prompts to see what kinds of images it can produce. Things to try One interesting aspect of the midjourney-diffusion model is its ability to blend realistic and fantastical elements. Try combining specific real-world objects or settings with more imaginative prompts to see how the model responds. You can also experiment with different prompt strengths and negative prompts to refine the output and achieve your desired results.
Updated 5/15/2024
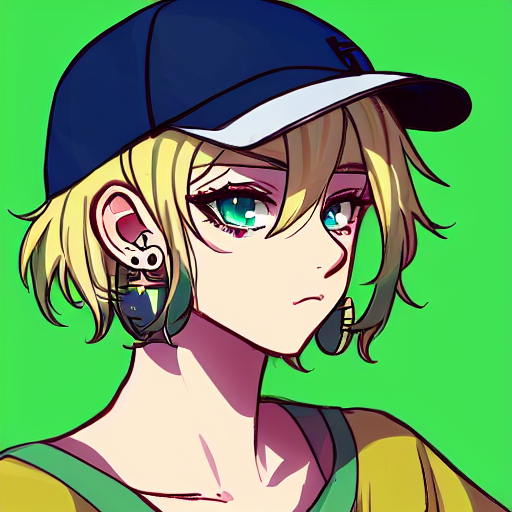
waifu-diffusion

650
waifu-diffusion is a latent text-to-image diffusion model that has been fine-tuned on high-quality anime images. It is similar to other anime-focused models like waifu-diffusion-v1-4 and waifu-diffusion-xl, as well as the more general stable-diffusion model. These models leverage the power of diffusion to generate highly detailed and photorealistic anime-style images from text prompts. Model inputs and outputs The waifu-diffusion model takes a text prompt as input and generates one or more anime-style images as output. The input prompt can describe various attributes like characters, settings, and artistic styles, and the model will attempt to generate matching images. Inputs Prompt**: The text prompt describing the desired image Seed**: A random seed value to control the generated image's randomness Width/Height**: The desired size of the output image Scheduler**: The diffusion scheduler algorithm to use Num Outputs**: The number of images to generate Guidance Scale**: The strength of the guidance toward the text prompt Negative Prompt**: Text describing attributes to avoid in the generated image Prompt Strength**: The strength of the prompt when using an initialization image Outputs Image(s)**: One or more generated anime-style images matching the input prompt Capabilities The waifu-diffusion model can generate a wide variety of high-quality anime-style images, from character portraits to detailed scenes. The model is capable of capturing complex artistic styles, intricate details, and vibrant colors. It can produce images of both human and non-human characters, as well as fantastical settings and environments. What can I use it for? The waifu-diffusion model can be used for a variety of creative and entertainment purposes, such as: Generating illustrations and concept art for anime, manga, or games Producing images for use in digital art, webcomics, or social media Experimenting with different prompts and styles to inspire new ideas Aiding in the visualization of creative writing or worldbuilding The model's open-source nature and permissive licensing also allow for potential commercial use, such as in downstream applications or as a service offering. Things to try One interesting aspect of the waifu-diffusion model is its ability to capture nuanced artistic styles and details. Try experimenting with specific prompt elements like "best quality", "masterpiece", or references to traditional media like "watercolor" to see how the model responds. You can also explore the impact of the "negative prompt" to refine the generated images further. Another avenue to explore is the model's capacity for generating diverse character designs and settings. Challenge the model with prompts that combine unusual elements or push the boundaries of what you expect from an anime-style image.
Updated 5/15/2024
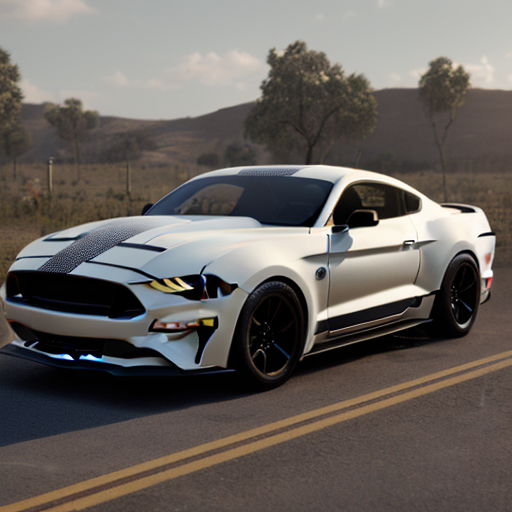
redshift-diffusion

115
redshift-diffusion is a text-to-image AI model created by tstramer that is capable of generating high-quality, photorealistic images from text prompts. It is a fine-tuned version of the Stable Diffusion 2.0 model, trained on a dataset of 3D images at 768x768 resolution. This model can produce stunning visuals in a "redshift" style, which features vibrant colors, futuristic elements, and a sense of depth and complexity. Compared to similar models like stable-diffusion, multidiffusion, and redshift-diffusion-768, redshift-diffusion offers a distinct visual style that can be particularly useful for creating futuristic, sci-fi, or cyberpunk-inspired imagery. The model's attention to detail and color palette make it well-suited for generating compelling character designs, fantastical landscapes, and imaginative scenes. Model inputs and outputs redshift-diffusion takes in a text prompt as its primary input, along with a variety of parameters that allow users to fine-tune the output, such as the number of inference steps, guidance scale, and more. The model outputs one or more high-resolution images (up to 1024x768 or 768x1024) that match the provided prompt. Inputs Prompt**: The text prompt describing the desired image. Seed**: An optional random seed value to ensure consistent outputs. Width/Height**: The desired dimensions of the output image. Scheduler**: The diffusion scheduler to use, such as DPMSolverMultistep. Num Outputs**: The number of images to generate (up to 4). Guidance Scale**: The scale for the classifier-free guidance, which affects the balance between the prompt and the model's learned priors. Negative Prompt**: Text describing elements that should not be present in the output image. Prompt Strength**: The strength of the input prompt when using an initialization image. Num Inference Steps**: The number of denoising steps to perform during image generation. Outputs Images**: One or more high-resolution images matching the provided prompt. Capabilities redshift-diffusion can generate a wide variety of photorealistic images, from fantastical characters and creatures to detailed landscapes and cityscapes. The model's strength lies in its ability to capture a distinct "redshift" visual style, which features vibrant colors, futuristic elements, and a sense of depth and complexity. This makes the model particularly well-suited for creating imaginative, sci-fi, and cyberpunk-inspired imagery. What can I use it for? redshift-diffusion can be a powerful tool for artists, designers, and creatives looking to generate unique and visually striking imagery. The model's capabilities lend themselves well to a range of applications, such as concept art, character design, album cover art, and even product visualizations. By leveraging the model's "redshift" style, users can create captivating, futuristic visuals that stand out from more conventional text-to-image outputs. Things to try One interesting aspect of redshift-diffusion is its ability to seamlessly blend fantastical and realistic elements. Try prompts that combine futuristic or science-fiction themes with recognizable objects or environments, such as "a robot bartender serving drinks in a neon-lit cyberpunk bar" or "a majestic alien spacecraft hovering over a lush, colorful landscape." The model's attention to detail and color palette can produce truly mesmerizing results that push the boundaries of what is possible with text-to-image generation.
Updated 5/15/2024
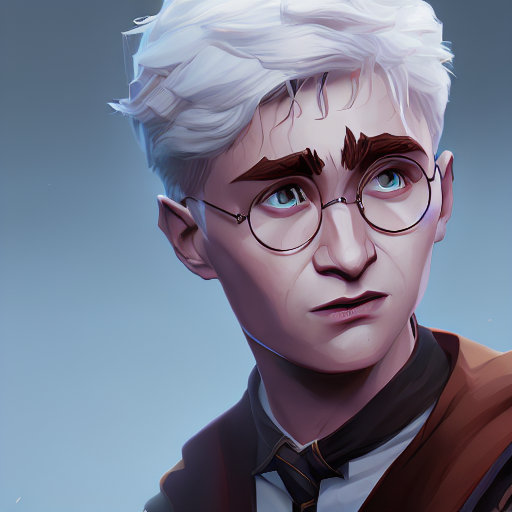
arcane-diffusion

100
arcane-diffusion is a fine-tuned Stable Diffusion model trained on images from the TV show Arcane. It can generate images with a distinct "Arcane style" when prompted with the token arcane style. The model is similar to other text-to-image diffusion models like Stable Diffusion, MultiDiffusion, and Stable Diffusion Speed Lab, but with a specialized training process to capture the visual style of Arcane. Model inputs and outputs arcane-diffusion takes a text prompt as input and generates one or more images as output. The model can generate images up to 1024x768 or 768x1024 pixels in size. Users can also specify a seed value, scheduler, number of outputs, guidance scale, negative prompt, and number of inference steps. Inputs Prompt**: The text prompt describing the desired image Seed**: A random seed value (leave blank to randomize) Width**: The width of the output image (max 1024) Height**: The height of the output image (max 768) Scheduler**: The denoising scheduler to use Num Outputs**: The number of images to generate (1-4) Guidance Scale**: The scale for classifier-free guidance Negative Prompt**: Text describing elements to exclude from the output Prompt Strength**: The strength of the prompt when using an init image Num Inference Steps**: The number of denoising steps (1-500) Outputs Image(s)**: One or more generated images matching the input prompt Capabilities arcane-diffusion can generate a wide variety of high-quality images with a distinct "Arcane style" inspired by the visual aesthetics of the TV show. The model is capable of producing detailed, photorealistic images of characters, environments, and objects in the Arcane universe. What can I use it for? You can use arcane-diffusion to create custom artwork, concept designs, and promotional materials inspired by the Arcane series. The model's specialized training allows it to capture the unique visual style of the show, making it a valuable tool for Arcane fans, artists, and content creators. You could potentially monetize the model's capabilities by offering custom image generation services or creating and selling Arcane-themed digital art and assets. Things to try Experiment with different prompts to see the range of images the arcane-diffusion model can produce. Try including details about characters, locations, or objects from the Arcane universe, and see how the model interprets and renders them. You can also play with the various input parameters like seed, guidance scale, and number of inference steps to fine-tune the output and achieve different visual effects.
Updated 5/15/2024
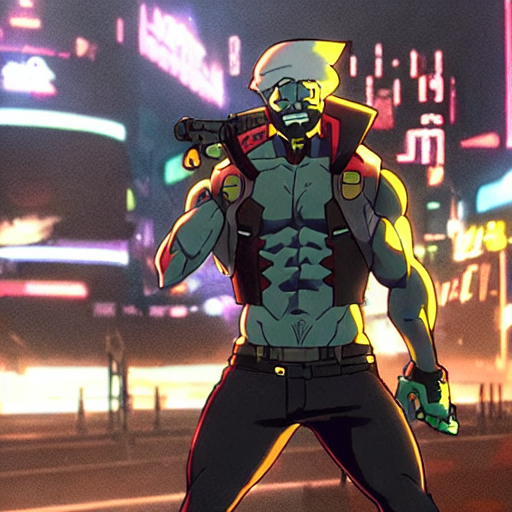
cyberpunk-anime-diffusion

80
The cyberpunk-anime-diffusion model is a text-to-image AI model trained by tstramer to generate cyberpunk-themed anime-style characters and scenes. It is based on the Waifu Diffusion V1.3 and Stable Diffusion V1.5 models, with additional training in Dreambooth to specialize in this unique art style. Similar models like eimis_anime_diffusion, stable-diffusion, dreamlike-anime, and lora-niji also specialize in generating high-quality anime-inspired imagery, but with a unique cyberpunk twist. Model inputs and outputs The cyberpunk-anime-diffusion model takes in a text prompt as its primary input, which is used to guide the image generation process. The model also accepts additional parameters like seed, image size, number of outputs, and various sampling configurations to further control the generated images. Inputs Prompt**: The text prompt describing the desired image Seed**: A random seed value to control image generation (leave blank to randomize) Width**: The width of the output image, up to a maximum of 1024 pixels Height**: The height of the output image, up to a maximum of 1024 pixels Scheduler**: The denoising scheduler to use, such as DPMSolverMultistep Num Outputs**: The number of images to generate (up to 4) Guidance Scale**: The scale for classifier-free guidance Negative Prompt**: Text describing things not to include in the output Prompt Strength**: The strength of the prompt when using an initial image Num Inference Steps**: The number of denoising steps to perform (1-500) Outputs Array of image URLs**: The generated images in the form of URLs, one for each requested output. Capabilities The cyberpunk-anime-diffusion model can generate highly detailed and stylized anime-inspired images with a cyberpunk aesthetic. The model excels at producing portraits of complex, expressive anime characters set against futuristic urban backdrops. It can also generate dynamic action scenes, machinery, and other cyberpunk-themed elements. What can I use it for? The cyberpunk-anime-diffusion model could be used to create illustrations, concept art, and promotional assets for anime, manga, or cyberpunk-themed media and projects. It could also be used to generate unique character designs or backgrounds for video games, films, or other visual storytelling mediums. Creators and developers interested in the intersection of anime and cyberpunk aesthetics would likely find this model particularly useful. Things to try When using the cyberpunk-anime-diffusion model, try incorporating the keyword "dgs" into your prompts to take advantage of the specialized training on the DGSpitzer illustration style. Experiment with different prompts that blend cyberpunk elements like futuristic cityscapes, advanced technology, and gritty urban environments with anime-inspired character designs and themes. The model responds well to detailed, specific prompts that allow it to showcase its unique capabilities.
Updated 5/15/2024
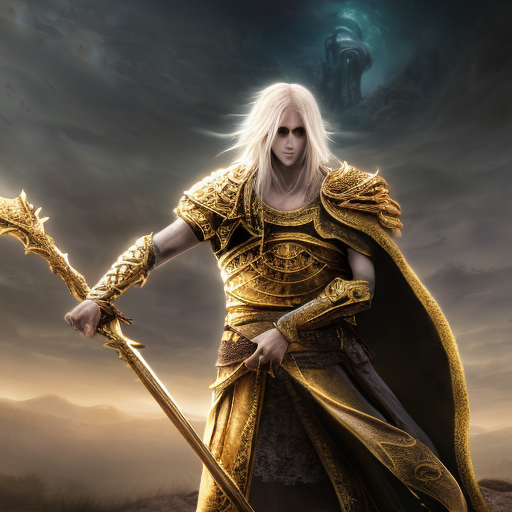
elden-ring-diffusion

63
elden-ring-diffusion is a fine-tuned version of the Stable Diffusion model, trained on game art from the popular video game Elden Ring. This model can generate images with a distinctive Elden Ring style, capturing the game's dark fantasy aesthetic. Compared to the original Stable Diffusion model, elden-ring-diffusion is specialized for creating content inspired by the Elden Ring universe. Other related AI models include MultiDiffusion, which explores fusing diffusion paths for controlled image generation, and OOT Diffusion, a virtual dressing room application. Model inputs and outputs The elden-ring-diffusion model takes in a text prompt as input and generates one or more images as output. The prompt can describe a scene, character, or concept in the Elden Ring style, and the model will attempt to create a corresponding image. The model also accepts parameters such as the number of outputs, the image size, and the seed value for reproducibility. Inputs Prompt**: The text prompt describing the desired image in the Elden Ring style Seed**: The random seed value, which can be left blank to randomize Width**: The width of the output image, up to a maximum of 1024 pixels Height**: The height of the output image, up to a maximum of 768 pixels Num Outputs**: The number of images to generate, up to a maximum of 4 Guidance Scale**: The scale for classifier-free guidance, which controls the influence of the text prompt Negative Prompt**: Additional text to specify things not to include in the output Num Inference Steps**: The number of denoising steps to perform during image generation Outputs Image(s)**: One or more images generated based on the input prompt and parameters Capabilities The elden-ring-diffusion model can generate a wide variety of images in the distinctive Elden Ring art style, including portraits of characters, landscapes, and fantastical scenes. The model's capabilities include creating highly detailed, photorealistic images that capture the dark, atmospheric quality of the Elden Ring universe. What can I use it for? You can use elden-ring-diffusion to create concept art, character designs, and background illustrations for Elden Ring-inspired projects, such as fan art, indie games, or personal creative endeavors. The model's specialized training on Elden Ring assets makes it well-suited for generating visuals that fit seamlessly into the game's world. Additionally, you could potentially use the model to create unique digital assets for commercial projects, such as book covers, movie posters, or merchandise, as long as you follow the terms of the model's CreativeML OpenRAIL-M license. Things to try Experiment with different prompts to see the range of Elden Ring-inspired images the model can generate. Try combining the elden ring style token with other descriptors, such as "dark fantasy", "gothic", or "medieval", to see how the model blends these elements. You can also play with the various input parameters, such as guidance scale and number of inference steps, to fine-tune the output and achieve the desired visual style.
Updated 5/15/2024
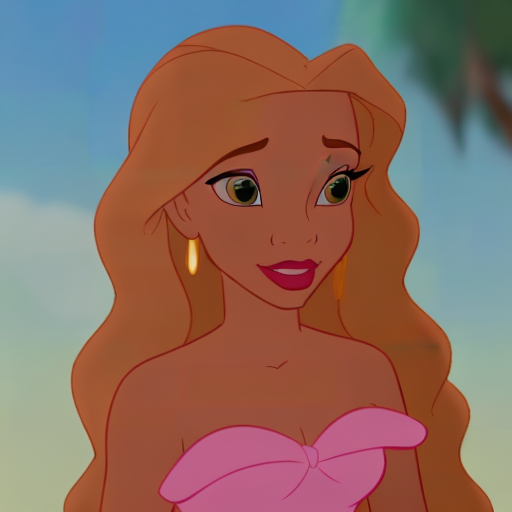
classic-anim-diffusion

52
classic-anim-diffusion is a text-to-image diffusion model that can generate animated images. It is similar to models like stable-diffusion, animate-diff, eimis_anime_diffusion, and animatediff-lightning-4-step, all of which aim to produce high-quality, detailed images from text prompts. Model inputs and outputs classic-anim-diffusion takes in a text prompt, along with parameters like image size, seed, and guidance scale. It outputs one or more animated images that match the input prompt. Inputs Prompt**: The text description of the image to generate Seed**: A random seed value, which can be left blank to randomize Width and Height**: The size of the output image, with a maximum of 1024x768 or 768x1024 Scheduler**: The algorithm used to generate the image Num Outputs**: The number of images to generate (up to 4) Guidance Scale**: The strength of the guidance towards the text prompt Negative Prompt**: Text to specify things not to include in the output Outputs One or more animated images matching the input prompt Capabilities classic-anim-diffusion can generate high-quality, detailed animated images from text prompts. It is capable of producing a wide range of scenes and styles, from realistic to fantastical. The model's ability to animate these images sets it apart from more static text-to-image models. What can I use it for? classic-anim-diffusion could be used for a variety of creative applications, such as generating animated illustrations, concept art, or short animated sequences. The model's flexibility and ability to produce unique, personalized content make it a powerful tool for artists, animators, and content creators looking to explore new ideas and styles. Things to try Experiment with different types of prompts to see the range of animated images classic-anim-diffusion can produce. Try combining the model with other tools or techniques, such as image editing software or post-processing, to further refine and enhance the generated output. Additionally, the model's ability to randomize the seed value allows for the exploration of numerous variations on a single prompt, potentially leading to unexpected and serendipitous results.
Updated 5/15/2024
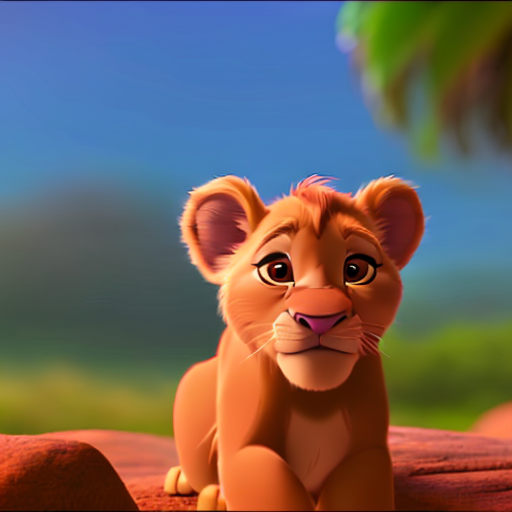
mo-di-diffusion

46
mo-di-diffusion is a diffusion model that can be used to generate videos by interpolating the latent space of Stable Diffusion. It was created by tstramer, who has also developed other video-focused diffusion models like Stable Diffusion Videos. The model is similar to MultiDiffusion, which also explores fusing diffusion paths for controlled image generation. Model inputs and outputs The mo-di-diffusion model takes in a text prompt, an optional init image, and various parameters to control the output. The inputs include the prompt, a random seed, image size, number of outputs, guidance scale, and the number of inference steps. The model then generates one or more images based on the input. Inputs Prompt**: The text prompt describing the desired output image Seed**: A random seed value to control the output Width**: The width of the output image (max 1024x768) Height**: The height of the output image (max 1024x768) Negative Prompt**: Specify things not to include in the output Num Outputs**: The number of images to generate (up to 4) Prompt Strength**: Controls how much the prompt influences an init image Guidance Scale**: Scales the influence of the classifier-free guidance Num Inference Steps**: The number of denoising steps to perform Outputs Array of image URIs**: The generated image(s) as a list of URIs Capabilities The mo-di-diffusion model can generate high-quality, photorealistic images from text prompts, similar to the capabilities of Stable Diffusion. However, the unique capability of this model is its ability to generate videos by interpolating the latent space of Stable Diffusion. This allows for the creation of dynamic, moving imagery that evolves over time based on the input prompt. What can I use it for? The mo-di-diffusion model could be used for a variety of creative and commercial applications, such as generating animated visuals for videos, making interactive art installations, or creating dynamic product visualizations. The ability to control the output through detailed prompts and parameters also opens up possibilities for use in film, gaming, or other media production. Additionally, as with other text-to-image models, the mo-di-diffusion model could be leveraged for content creation, visual marketing, and prototyping. Things to try One interesting aspect of the mo-di-diffusion model is its potential for generating dynamic, transformative imagery. By playing with the prompt, seed, and other parameters, users could experiment with creating videos that morph and evolve over time, leading to surreal and unexpected visual narratives. Additionally, combining the model's video capabilities with other tools for audio, 3D modeling, or animation could result in highly immersive and engaging multimedia experiences.
Updated 5/15/2024
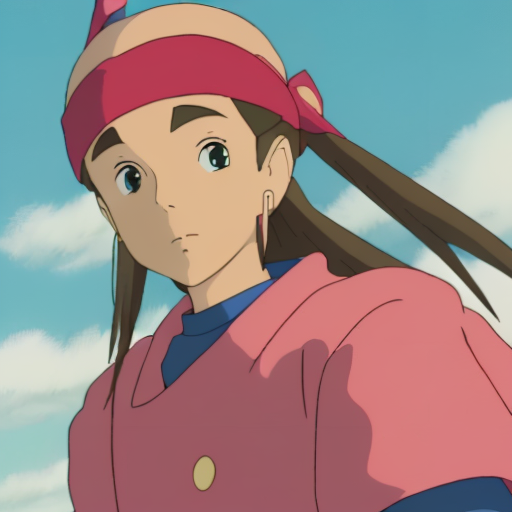
ghibli-diffusion

44
The ghibli-diffusion model is a fine-tuned Stable Diffusion model trained on images from modern anime feature films by Studio Ghibli. This model can generate images in the distinctive visual style of Studio Ghibli, known for its detailed, imaginative worlds and memorable characters. Compared to the original Stable Diffusion model, the ghibli-diffusion model has been specialized to produce art with a Ghibli-esque aesthetic. Other similar models include studio-ghibli, eimis_anime_diffusion, and sdxl-pixar, each with their own unique specializations. Model inputs and outputs The ghibli-diffusion model takes a text prompt as input and generates one or more corresponding images. Users can control various aspects of the image generation, including the size, number of outputs, guidance scale, and number of inference steps. The model also accepts a seed value to allow for reproducible random generation. Inputs Prompt**: The text description of the desired image Seed**: A random seed value to use for generating the image Width/Height**: The desired size of the output image Num Outputs**: The number of images to generate Guidance Scale**: The scale for classifier-free guidance Num Inference Steps**: The number of denoising steps to perform Negative Prompt**: Text describing aspects to avoid in the output Outputs Images**: One or more generated images matching the input prompt Capabilities The ghibli-diffusion model can generate a wide variety of Ghibli-inspired images, from detailed characters and creatures to fantastical landscapes and environments. The model excels at capturing the whimsical, hand-drawn aesthetic of classic Ghibli films, with soft brushstrokes, vibrant colors, and a sense of wonder. What can I use it for? The ghibli-diffusion model is well-suited for creating concept art, illustrations, and fan art inspired by Studio Ghibli films. Artists and designers could use this model to quickly generate Ghibli-style images as a starting point for their own creative projects, or to produce Ghibli-themed artwork, merchandise, and promotional materials. The model's ability to generate multiple variations on a single prompt also makes it useful for ideation and experimentation. Things to try Try using the model to generate images of specific Ghibli characters, animals, or settings by incorporating relevant keywords into your prompts. Experiment with adjusting the guidance scale and number of inference steps to find the right balance between detail and cohesion. You can also try using the model to create unique blends of Ghibli aesthetics with other styles or genres, such as science fiction or fantasy.
Updated 5/15/2024