Cszn
Models by this creator
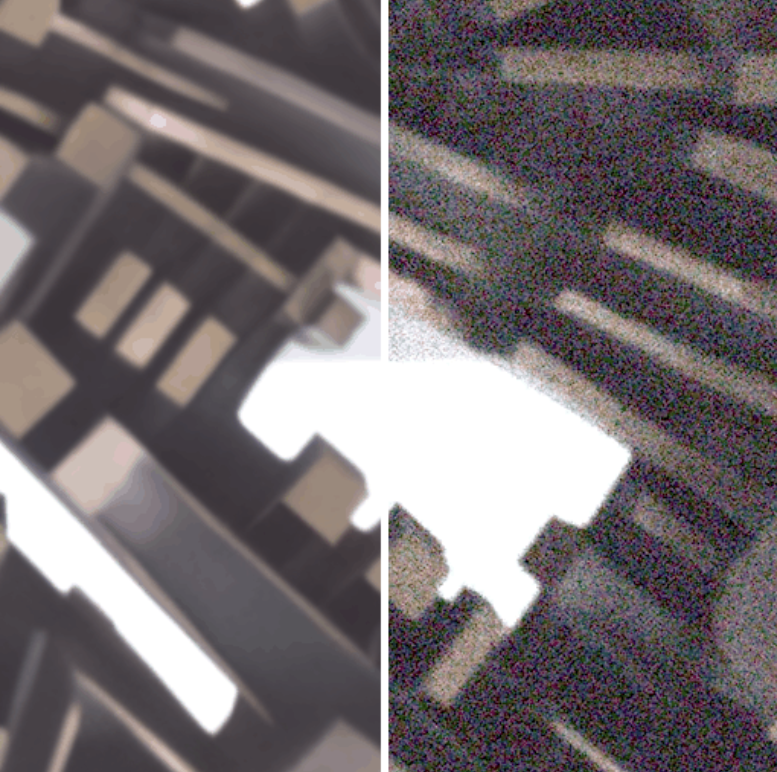
scunet

21
SCUNet is a powerful blind image denoising model developed by researcher cszn. It utilizes a Swin-Conv-UNet (SCUNet) architecture that combines the strengths of Swin Transformer blocks and residual convolutional blocks within a UNet backbone. This unique design allows SCUNet to effectively remove various types of noise from images, including Gaussian, Poisson, and realistic camera sensor noise. Compared to similar models like lednet, swinir, and codeformer from the same creator, SCUNet focuses specifically on the task of blind image denoising, achieving state-of-the-art performance. Model inputs and outputs SCUNet takes a single image as input and outputs a denoised version of that image. The model is designed to handle a variety of noise types, including Gaussian, Poisson, and realistic camera sensor noise, making it a practical solution for real-world image denoising tasks. Inputs Image**: The input image that needs to be denoised. Outputs Denoised Image**: The denoised version of the input image, with various types of noise removed. Image with Added Noise**: The input image with simulated noise added, for comparison purposes. Capabilities SCUNet has demonstrated impressive capabilities in blind image denoising, outperforming many state-of-the-art models in both Gaussian and real-world noise removal tasks. The model's unique architecture, which combines the strengths of Swin Transformer and residual convolutional blocks, allows it to effectively capture both local and global image features, resulting in high-quality denoising results. What can I use it for? SCUNet can be a valuable tool for a wide range of applications that require high-quality image denoising, such as: Photography**: Enhancing low-light or noisy images captured by cameras, smartphones, or other imaging devices. Video processing**: Improving the visual quality of video footage by removing unwanted noise and artifacts. Medical imaging**: Enhancing the clarity of medical images, such as X-rays, CT scans, or MRI scans, to aid in diagnosis and analysis. Satellite and aerial imagery**: Improving the quality of images captured by satellites or drones, which can be affected by atmospheric conditions or sensor noise. Things to try One interesting aspect of SCUNet is its ability to achieve high-quality denoising results using only synthetic training data, without relying on paired noisy/clean datasets like DND and SIDD. This demonstrates the model's robustness and flexibility, as it can be applied to a wide range of real-world denoising scenarios where paired training data may not be available. Researchers and practitioners may be interested in exploring the potential of SCUNet for other image restoration tasks, such as super-resolution or inpainting, where the model's ability to capture multi-scale features could be beneficial.
Updated 5/9/2024